Gemma 3 Technical Report
Gemma Team, Aishwarya Kamath, Johan Ferret, Shreya Pathak, Nino Vieillard, Ramona Merhej, Sarah Perrin, Tatiana Matejovicova, Alexandre Ramé, Morgane Rivière, Louis Rouillard, Thomas Mesnard, Geoffrey Cideron, Jean-bastien Grill, Sabela Ramos, Edouard Yvinec, Michelle Casbon, Etienne Pot, Ivo Penchev, Gaël Liu, Francesco Visin, Kathleen Kenealy
2025-03-27
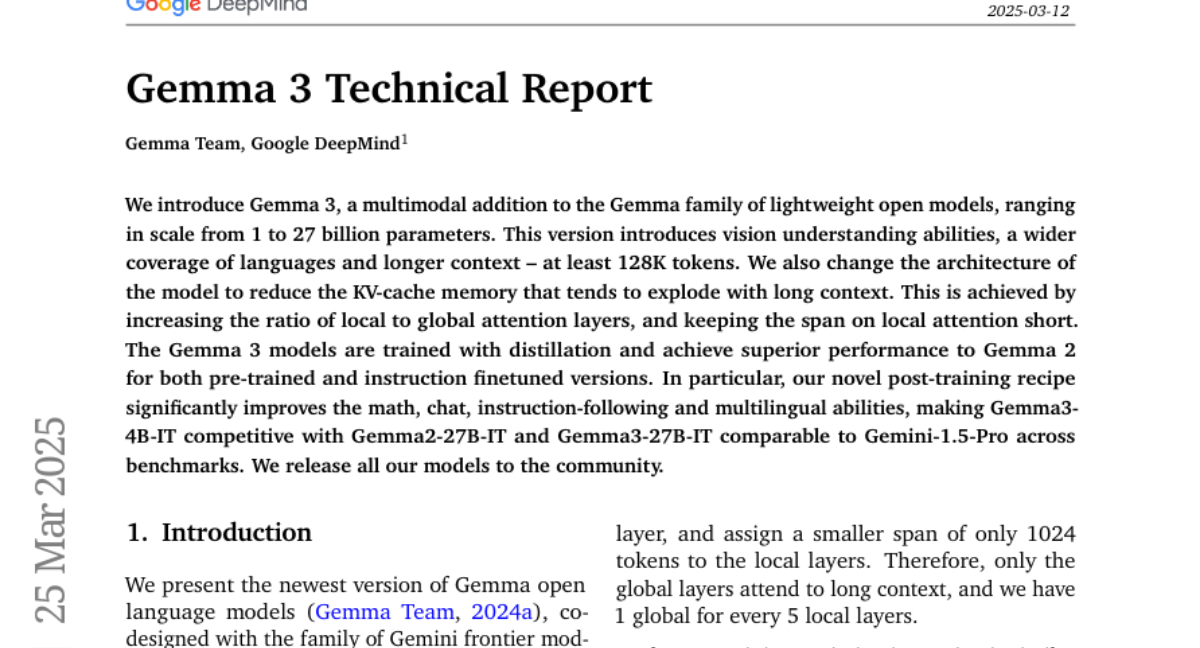
Summary
This paper introduces Gemma 3, which is a new version of AI models that are designed to be small and easy to use, but still powerful.
What's the problem?
Previous versions of these models had limitations in understanding images, languages, and long pieces of text, and they also used a lot of memory.
What's the solution?
The researchers improved the model's ability to understand images and languages, made it capable of processing longer pieces of text, and changed the way it works to use less memory.
Why it matters?
This work matters because it makes these AI models more versatile and efficient, allowing them to be used in a wider range of applications.
Abstract
We introduce Gemma 3, a multimodal addition to the Gemma family of lightweight open models, ranging in scale from 1 to 27 billion parameters. This version introduces vision understanding abilities, a wider coverage of languages and longer context - at least 128K tokens. We also change the architecture of the model to reduce the KV-cache memory that tends to explode with long context. This is achieved by increasing the ratio of local to global attention layers, and keeping the span on local attention short. The Gemma 3 models are trained with distillation and achieve superior performance to Gemma 2 for both pre-trained and instruction finetuned versions. In particular, our novel post-training recipe significantly improves the math, chat, instruction-following and multilingual abilities, making Gemma3-4B-IT competitive with Gemma2-27B-IT and Gemma3-27B-IT comparable to Gemini-1.5-Pro across benchmarks. We release all our models to the community.