Generative Photomontage
Sean J. Liu, Nupur Kumari, Ariel Shamir, Jun-Yan Zhu
2024-08-15
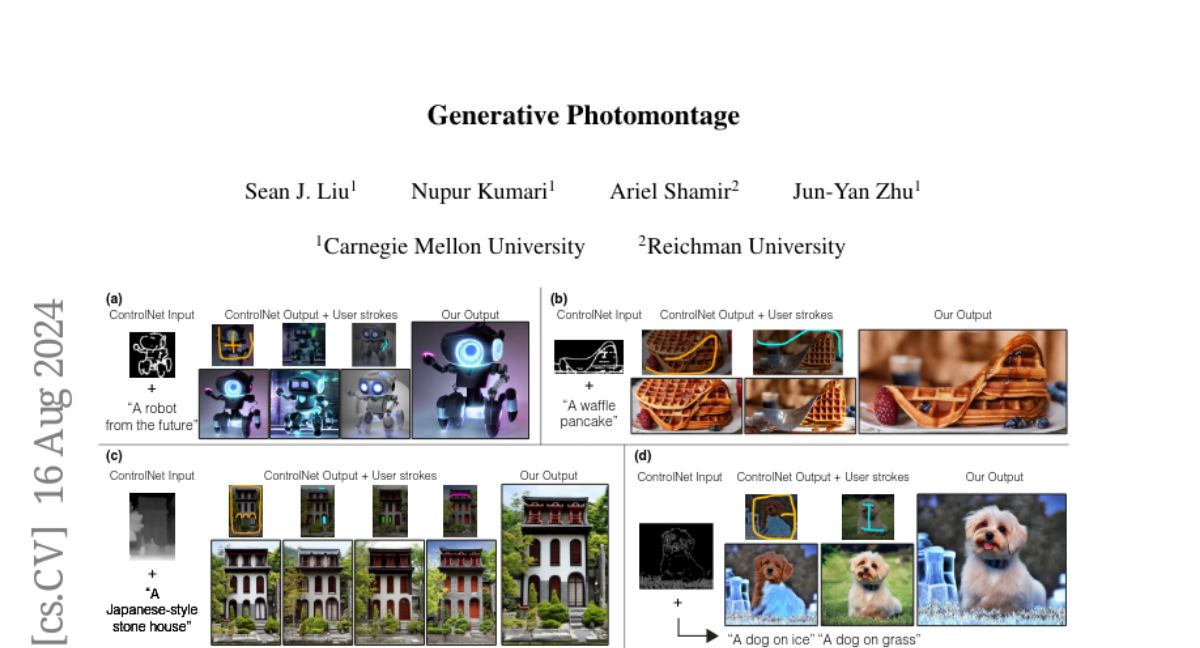
Summary
This paper presents Generative Photomontage, a new method for creating images by combining different parts from various generated images to better match what users want.
What's the problem?
Current text-to-image models can create images but often produce results that don't fully capture what a user is looking for. The generation process can feel random, making it hard to get a single perfect image that includes all desired elements.
What's the solution?
The authors propose a framework called Generative Photomontage, which allows users to select specific parts from multiple generated images using a brush stroke interface. The system then uses advanced techniques to segment these selected parts and blend them together seamlessly, ensuring that the final image reflects the user's choices while maintaining quality.
Why it matters?
This research is important because it improves the way users can create images using AI, making it more interactive and tailored to individual needs. By allowing users to combine elements from different images easily, it opens up new possibilities in fields like art, design, and content creation.
Abstract
Text-to-image models are powerful tools for image creation. However, the generation process is akin to a dice roll and makes it difficult to achieve a single image that captures everything a user wants. In this paper, we propose a framework for creating the desired image by compositing it from various parts of generated images, in essence forming a Generative Photomontage. Given a stack of images generated by ControlNet using the same input condition and different seeds, we let users select desired parts from the generated results using a brush stroke interface. We introduce a novel technique that takes in the user's brush strokes, segments the generated images using a graph-based optimization in diffusion feature space, and then composites the segmented regions via a new feature-space blending method. Our method faithfully preserves the user-selected regions while compositing them harmoniously. We demonstrate that our flexible framework can be used for many applications, including generating new appearance combinations, fixing incorrect shapes and artifacts, and improving prompt alignment. We show compelling results for each application and demonstrate that our method outperforms existing image blending methods and various baselines.