Geometry Image Diffusion: Fast and Data-Efficient Text-to-3D with Image-Based Surface Representation
Slava Elizarov, Ciara Rowles, Simon Donné
2024-09-06
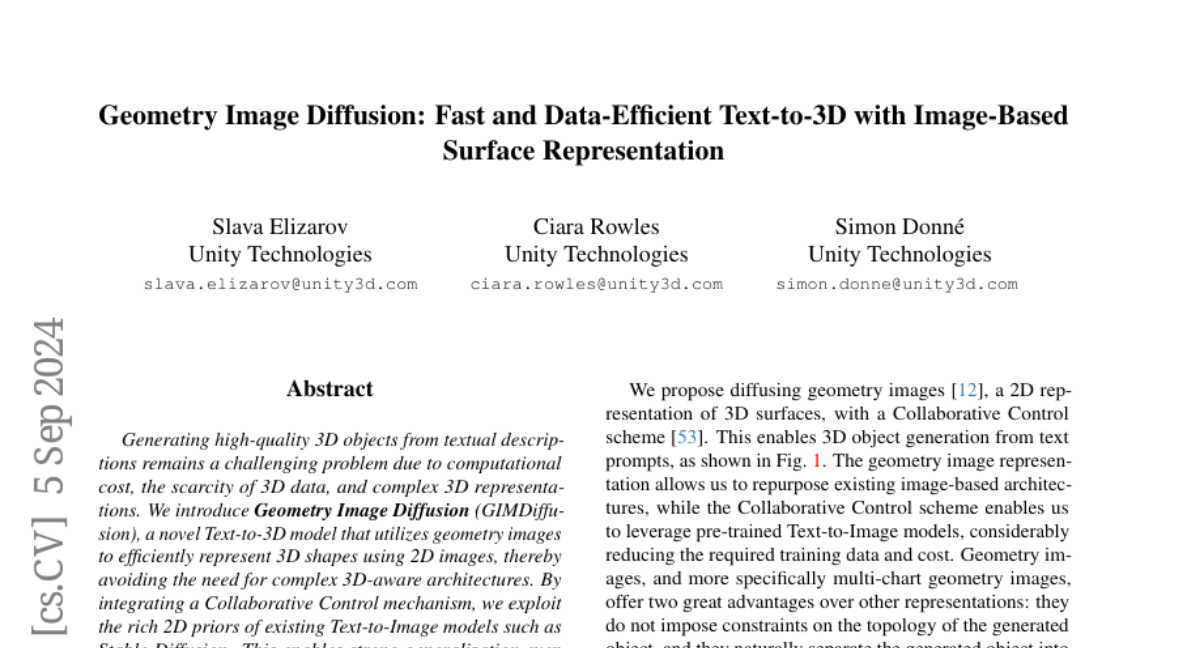
Summary
This paper talks about Geometry Image Diffusion (GIMDiffusion), a new method for quickly and efficiently creating 3D objects from text descriptions using images.
What's the problem?
Creating high-quality 3D models from text descriptions is difficult because it requires a lot of computing power and data. Most existing methods rely on complex 3D models, which can be slow and inefficient, especially when there isn't enough 3D training data available.
What's the solution?
GIMDiffusion addresses these challenges by using geometry images, which are 2D representations that simplify the process of creating 3D shapes. This method allows the model to work with existing 2D image data from text-to-image models like Stable Diffusion, making it easier to generate 3D objects even with limited training data. The approach also includes a Collaborative Control mechanism that enhances the model's ability to generalize and adapt to different tasks without needing extensive adjustments.
Why it matters?
This research is important because it makes it faster and easier to create detailed 3D models from simple text prompts. By improving the efficiency of this process, GIMDiffusion can benefit various fields, including gaming, virtual reality, and design, where high-quality 3D assets are essential.
Abstract
Generating high-quality 3D objects from textual descriptions remains a challenging problem due to computational cost, the scarcity of 3D data, and complex 3D representations. We introduce Geometry Image Diffusion (GIMDiffusion), a novel Text-to-3D model that utilizes geometry images to efficiently represent 3D shapes using 2D images, thereby avoiding the need for complex 3D-aware architectures. By integrating a Collaborative Control mechanism, we exploit the rich 2D priors of existing Text-to-Image models such as Stable Diffusion. This enables strong generalization even with limited 3D training data (allowing us to use only high-quality training data) as well as retaining compatibility with guidance techniques such as IPAdapter. In short, GIMDiffusion enables the generation of 3D assets at speeds comparable to current Text-to-Image models. The generated objects consist of semantically meaningful, separate parts and include internal structures, enhancing both usability and versatility.