"Give Me BF16 or Give Me Death"? Accuracy-Performance Trade-Offs in LLM Quantization
Eldar Kurtic, Alexandre Marques, Shubhra Pandit, Mark Kurtz, Dan Alistarh
2024-11-05
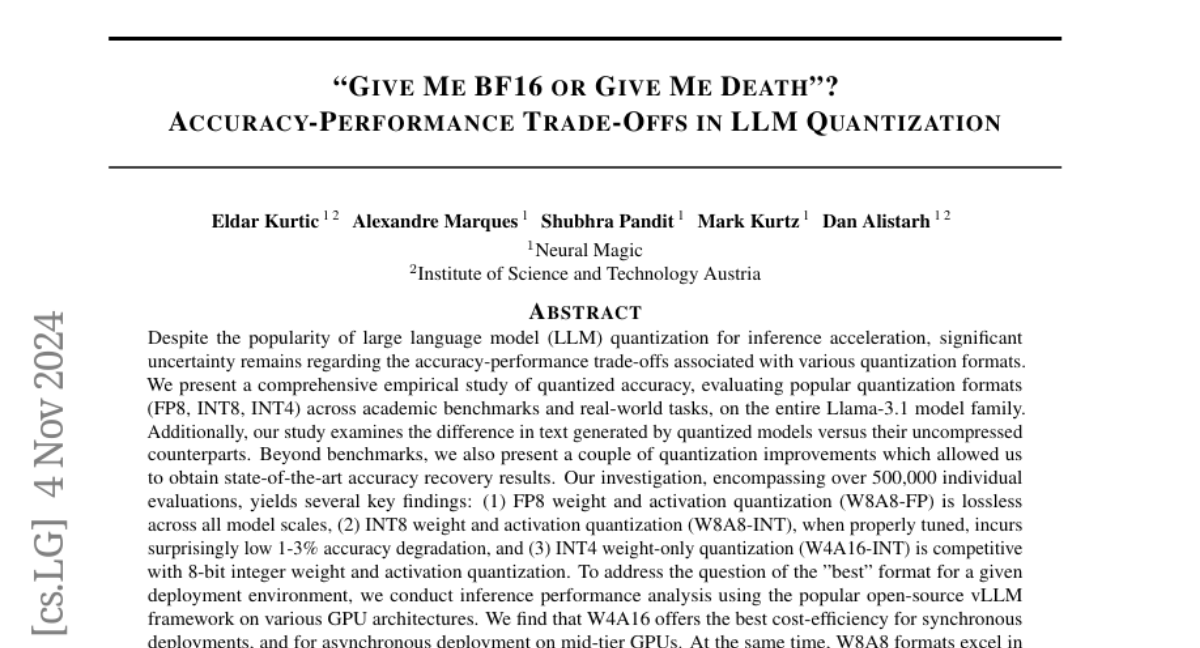
Summary
This paper discusses the trade-offs between accuracy and performance when quantizing large language models (LLMs) for faster processing. It examines different methods of reducing the data size used by these models while trying to maintain their effectiveness.
What's the problem?
As LLMs become more popular, there's a need to make them faster and more efficient without losing too much accuracy in their responses. However, different quantization methods (which reduce the amount of data the model uses) can lead to uncertainty about how much accuracy is sacrificed in exchange for speed. This makes it challenging to choose the best method for specific tasks.
What's the solution?
The authors conducted a thorough study comparing various quantization formats, including FP8, INT8, and INT4, across different tasks and benchmarks using the Llama-3.1 model family. They found that FP8 quantization maintains accuracy without any loss, while INT8 quantization only slightly reduces accuracy (by 1-3%) when properly tuned. They also discovered that INT4 quantization can perform comparably to 8-bit methods. The study provides guidelines on which quantization method works best depending on the deployment scenario, such as synchronous or asynchronous tasks.
Why it matters?
This research is important because it helps developers and researchers understand how to effectively use LLMs in real-world applications. By providing clear insights into how different quantization methods affect performance and accuracy, this study can lead to better implementations of AI models in various technologies, making them faster and more efficient while still delivering reliable results.
Abstract
Despite the popularity of large language model (LLM) quantization for inference acceleration, significant uncertainty remains regarding the accuracy-performance trade-offs associated with various quantization formats. We present a comprehensive empirical study of quantized accuracy, evaluating popular quantization formats (FP8, INT8, INT4) across academic benchmarks and real-world tasks, on the entire Llama-3.1 model family. Additionally, our study examines the difference in text generated by quantized models versus their uncompressed counterparts. Beyond benchmarks, we also present a couple of quantization improvements which allowed us to obtain state-of-the-art accuracy recovery results. Our investigation, encompassing over 500,000 individual evaluations, yields several key findings: (1) FP8 weight and activation quantization (W8A8-FP) is lossless across all model scales, (2) INT8 weight and activation quantization (W8A8-INT), when properly tuned, incurs surprisingly low 1-3% accuracy degradation, and (3) INT4 weight-only quantization (W4A16-INT) is competitive with 8-bit integer weight and activation quantization. To address the question of the "best" format for a given deployment environment, we conduct inference performance analysis using the popular open-source vLLM framework on various GPU architectures. We find that W4A16 offers the best cost-efficiency for synchronous deployments, and for asynchronous deployment on mid-tier GPUs. At the same time, W8A8 formats excel in asynchronous "continuous batching" deployment of mid- and large-size models on high-end GPUs. Our results provide a set of practical guidelines for deploying quantized LLMs across scales and performance requirements.