GMAI-MMBench: A Comprehensive Multimodal Evaluation Benchmark Towards General Medical AI
Pengcheng Chen, Jin Ye, Guoan Wang, Yanjun Li, Zhongying Deng, Wei Li, Tianbin Li, Haodong Duan, Ziyan Huang, Yanzhou Su, Benyou Wang, Shaoting Zhang, Bin Fu, Jianfei Cai, Bohan Zhuang, Eric J Seibel, Junjun He, Yu Qiao
2024-08-09
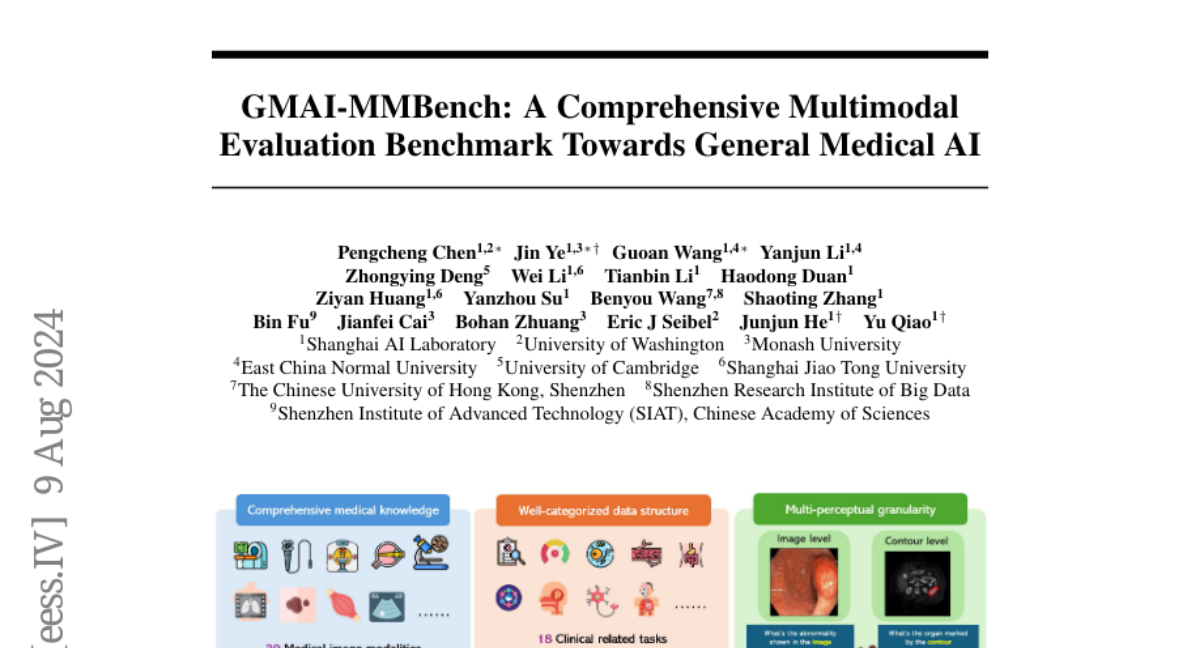
Summary
This paper introduces GMAI-MMBench, a comprehensive evaluation benchmark designed to assess the performance of large vision-language models (LVLMs) in medical applications.
What's the problem?
As AI technology grows in the medical field, it's important to have effective ways to evaluate how well these models work. Current benchmarks often focus on specific areas and lack the variety needed to fully test the capabilities of LVLMs. This leads to challenges like limited relevance to real clinical situations and incomplete assessments of model performance.
What's the solution?
To address these issues, the authors created GMAI-MMBench, which includes a diverse set of data from 285 datasets covering 39 medical image types and 18 clinical tasks. This benchmark allows for a more thorough evaluation by using a structured approach that categorizes tasks and provides multiple levels of detail. It also features a customizable structure that lets users tailor evaluations to their specific needs, making it easier to assess different aspects of model performance.
Why it matters?
This research is significant because it provides a more robust tool for evaluating AI systems in healthcare. By improving how we assess these models, GMAI-MMBench can help drive advancements in medical AI, leading to better tools for diagnosis and treatment that are more effective in real-world situations.
Abstract
Large Vision-Language Models (LVLMs) are capable of handling diverse data types such as imaging, text, and physiological signals, and can be applied in various fields. In the medical field, LVLMs have a high potential to offer substantial assistance for diagnosis and treatment. Before that, it is crucial to develop benchmarks to evaluate LVLMs' effectiveness in various medical applications. Current benchmarks are often built upon specific academic literature, mainly focusing on a single domain, and lacking varying perceptual granularities. Thus, they face specific challenges, including limited clinical relevance, incomplete evaluations, and insufficient guidance for interactive LVLMs. To address these limitations, we developed the GMAI-MMBench, the most comprehensive general medical AI benchmark with well-categorized data structure and multi-perceptual granularity to date. It is constructed from 285 datasets across 39 medical image modalities, 18 clinical-related tasks, 18 departments, and 4 perceptual granularities in a Visual Question Answering (VQA) format. Additionally, we implemented a lexical tree structure that allows users to customize evaluation tasks, accommodating various assessment needs and substantially supporting medical AI research and applications. We evaluated 50 LVLMs, and the results show that even the advanced GPT-4o only achieves an accuracy of 52%, indicating significant room for improvement. Moreover, we identified five key insufficiencies in current cutting-edge LVLMs that need to be addressed to advance the development of better medical applications. We believe that GMAI-MMBench will stimulate the community to build the next generation of LVLMs toward GMAI. Project Page: https://uni-medical.github.io/GMAI-MMBench.github.io/