Golden Touchstone: A Comprehensive Bilingual Benchmark for Evaluating Financial Large Language Models
Xiaojun Wu, Junxi Liu, Huanyi Su, Zhouchi Lin, Yiyan Qi, Chengjin Xu, Jiajun Su, Jiajie Zhong, Fuwei Wang, Saizhuo Wang, Fengrui Hua, Jia Li, Jian Guo
2024-11-12
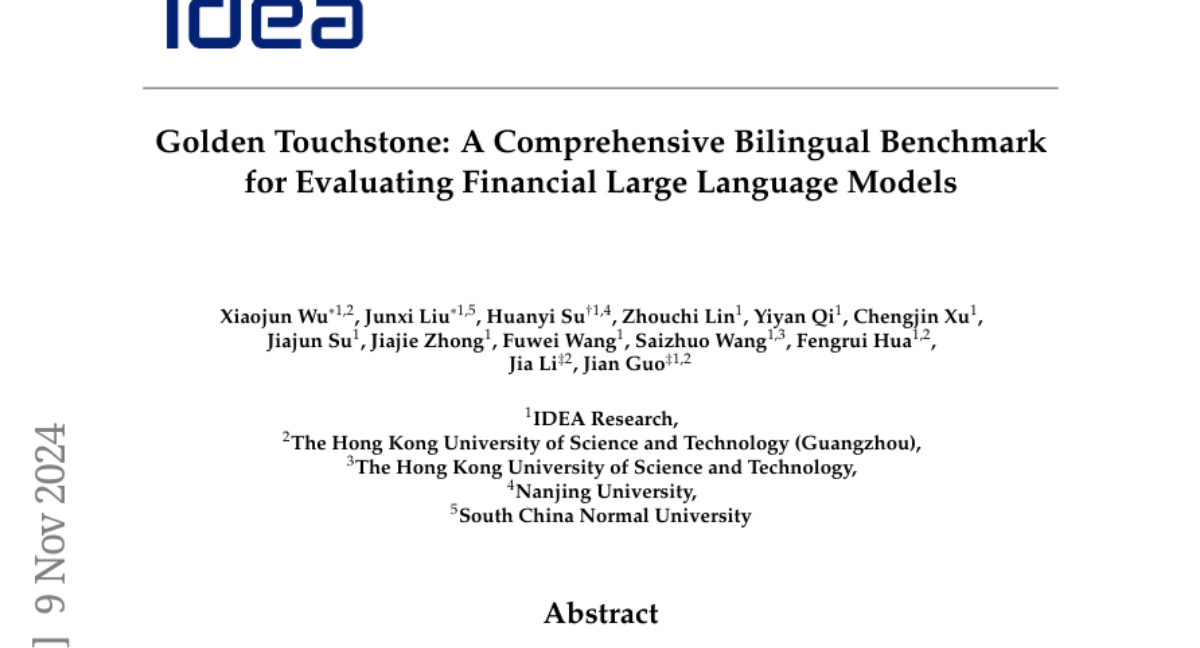
Summary
This paper introduces Golden Touchstone, a new bilingual benchmark designed to evaluate the performance of large language models (LLMs) specifically in the financial sector, using both Chinese and English datasets.
What's the problem?
As large language models are increasingly used in finance, there is a need for a standardized way to assess their performance. Many existing benchmarks are limited in the languages they cover and the types of tasks they evaluate, leading to challenges such as low-quality datasets and a lack of adaptability for different financial tasks. This makes it difficult to accurately measure how well these models understand and generate financial language.
What's the solution?
Golden Touchstone addresses these issues by providing a comprehensive bilingual benchmark that includes representative datasets from both Chinese and English across eight key financial tasks. The benchmark is developed from extensive open-source data collection and is tailored to meet industry-specific needs. It allows for thorough evaluation of LLMs' capabilities in understanding and generating financial text. Additionally, the authors introduced Touchstone-GPT, a financial LLM trained with this benchmark, which demonstrates strong performance but still has some limitations.
Why it matters?
This research is important because it offers a practical tool for evaluating financial language models, helping researchers and developers understand their strengths and weaknesses. By providing a standardized benchmark, Golden Touchstone can guide future improvements in financial AI, leading to more accurate and effective applications in areas like investment analysis, risk management, and automated customer service.
Abstract
As large language models become increasingly prevalent in the financial sector, there is a pressing need for a standardized method to comprehensively assess their performance. However, existing finance benchmarks often suffer from limited language and task coverage, as well as challenges such as low-quality datasets and inadequate adaptability for LLM evaluation. To address these limitations, we propose "Golden Touchstone", the first comprehensive bilingual benchmark for financial LLMs, which incorporates representative datasets from both Chinese and English across eight core financial NLP tasks. Developed from extensive open source data collection and industry-specific demands, this benchmark includes a variety of financial tasks aimed at thoroughly assessing models' language understanding and generation capabilities. Through comparative analysis of major models on the benchmark, such as GPT-4o Llama3, FinGPT and FinMA, we reveal their strengths and limitations in processing complex financial information. Additionally, we open-sourced Touchstone-GPT, a financial LLM trained through continual pre-training and financial instruction tuning, which demonstrates strong performance on the bilingual benchmark but still has limitations in specific tasks.This research not only provides the financial large language models with a practical evaluation tool but also guides the development and optimization of future research. The source code for Golden Touchstone and model weight of Touchstone-GPT have been made publicly available at https://github.com/IDEA-FinAI/Golden-Touchstone, contributing to the ongoing evolution of FinLLMs and fostering further research in this critical area.