Grasping Diverse Objects with Simulated Humanoids
Zhengyi Luo, Jinkun Cao, Sammy Christen, Alexander Winkler, Kris Kitani, Weipeng Xu
2024-07-17
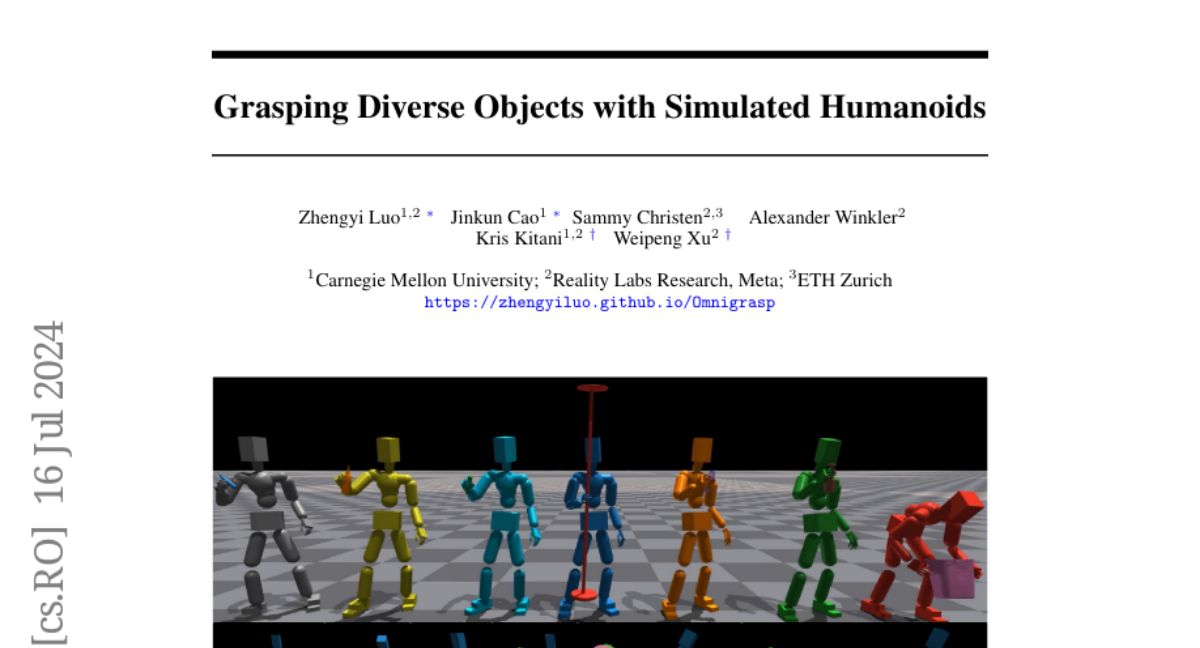
Summary
This paper introduces Animate3D, a new method for animating static 3D models using advanced techniques that combine multiple views of the model and video diffusion.
What's the problem?
Traditional methods for animating 3D models often rely on single images or text descriptions, which can lead to inconsistent and unrealistic animations. These methods struggle to effectively use multiple views of an object, making it hard to create smooth and lifelike movements.
What's the solution?
Animate3D addresses these issues by using a multi-view video diffusion model (MV-VDM) that takes advantage of various angles of a static 3D object. The framework includes a two-step process: first, it reconstructs motion from generated multi-view videos, and then it refines the animation using a technique called 4D Score Distillation Sampling (4D-SDS). This approach ensures that both the movement and appearance of the animated model are high quality and consistent.
Why it matters?
This research is important because it allows for more realistic animations of 3D models, which can be used in video games, movies, and virtual reality applications. By improving how we animate objects, Animate3D can help creators produce engaging and lifelike characters and scenes, enhancing the overall experience for viewers.
Abstract
We present a method for controlling a simulated humanoid to grasp an object and move it to follow an object trajectory. Due to the challenges in controlling a humanoid with dexterous hands, prior methods often use a disembodied hand and only consider vertical lifts or short trajectories. This limited scope hampers their applicability for object manipulation required for animation and simulation. To close this gap, we learn a controller that can pick up a large number (>1200) of objects and carry them to follow randomly generated trajectories. Our key insight is to leverage a humanoid motion representation that provides human-like motor skills and significantly speeds up training. Using only simplistic reward, state, and object representations, our method shows favorable scalability on diverse object and trajectories. For training, we do not need dataset of paired full-body motion and object trajectories. At test time, we only require the object mesh and desired trajectories for grasping and transporting. To demonstrate the capabilities of our method, we show state-of-the-art success rates in following object trajectories and generalizing to unseen objects. Code and models will be released.