GRUtopia: Dream General Robots in a City at Scale
Hanqing Wang, Jiahe Chen, Wensi Huang, Qingwei Ben, Tai Wang, Boyu Mi, Tao Huang, Siheng Zhao, Yilun Chen, Sizhe Yang, Peizhou Cao, Wenye Yu, Zichao Ye, Jialun Li, Junfeng Long, Zirui Wang, Huiling Wang, Ying Zhao, Zhongying Tu, Yu Qiao, Dahua Lin, Jiangmiao Pang
2024-07-16
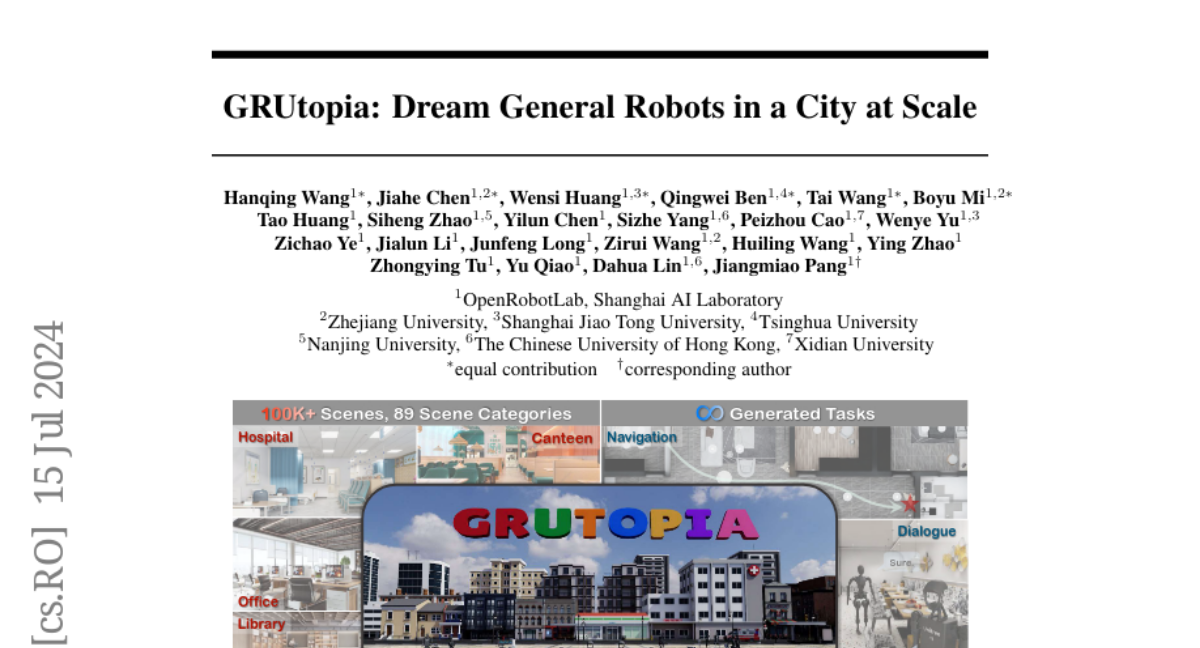
Summary
This paper introduces GRUtopia, a project that creates a simulated 3D environment for robots to learn and interact, helping advance the field of Embodied AI.
What's the problem?
Training robots to operate in real-world environments is expensive and time-consuming because it requires collecting a lot of real-world data. This makes it difficult to develop robots that can perform tasks in various settings, especially in complex urban environments.
What's the solution?
GRUtopia addresses this issue by providing a virtual city where robots can practice and learn. It includes a large dataset called GRScenes with 100,000 interactive scenes that cover a wide range of environments beyond just homes. Additionally, it features GRResidents, which are AI characters that help simulate social interactions and tasks for the robots. The project also includes GRBench, a benchmark for testing robot performance on different tasks like navigating and manipulating objects.
Why it matters?
This research is important because it helps create high-quality training environments for robots without the high costs associated with real-world data collection. By using a simulated society, GRUtopia allows researchers to develop and test robots more effectively, which could lead to better robots that can assist in various service roles in our daily lives.
Abstract
Recent works have been exploring the scaling laws in the field of Embodied AI. Given the prohibitive costs of collecting real-world data, we believe the Simulation-to-Real (Sim2Real) paradigm is a crucial step for scaling the learning of embodied models. This paper introduces project GRUtopia, the first simulated interactive 3D society designed for various robots. It features several advancements: (a) The scene dataset, GRScenes, includes 100k interactive, finely annotated scenes, which can be freely combined into city-scale environments. In contrast to previous works mainly focusing on home, GRScenes covers 89 diverse scene categories, bridging the gap of service-oriented environments where general robots would be initially deployed. (b) GRResidents, a Large Language Model (LLM) driven Non-Player Character (NPC) system that is responsible for social interaction, task generation, and task assignment, thus simulating social scenarios for embodied AI applications. (c) The benchmark, GRBench, supports various robots but focuses on legged robots as primary agents and poses moderately challenging tasks involving Object Loco-Navigation, Social Loco-Navigation, and Loco-Manipulation. We hope that this work can alleviate the scarcity of high-quality data in this field and provide a more comprehensive assessment of Embodied AI research. The project is available at https://github.com/OpenRobotLab/GRUtopia.