Guiding Vision-Language Model Selection for Visual Question-Answering Across Tasks, Domains, and Knowledge Types
Neelabh Sinha, Vinija Jain, Aman Chadha
2024-09-17
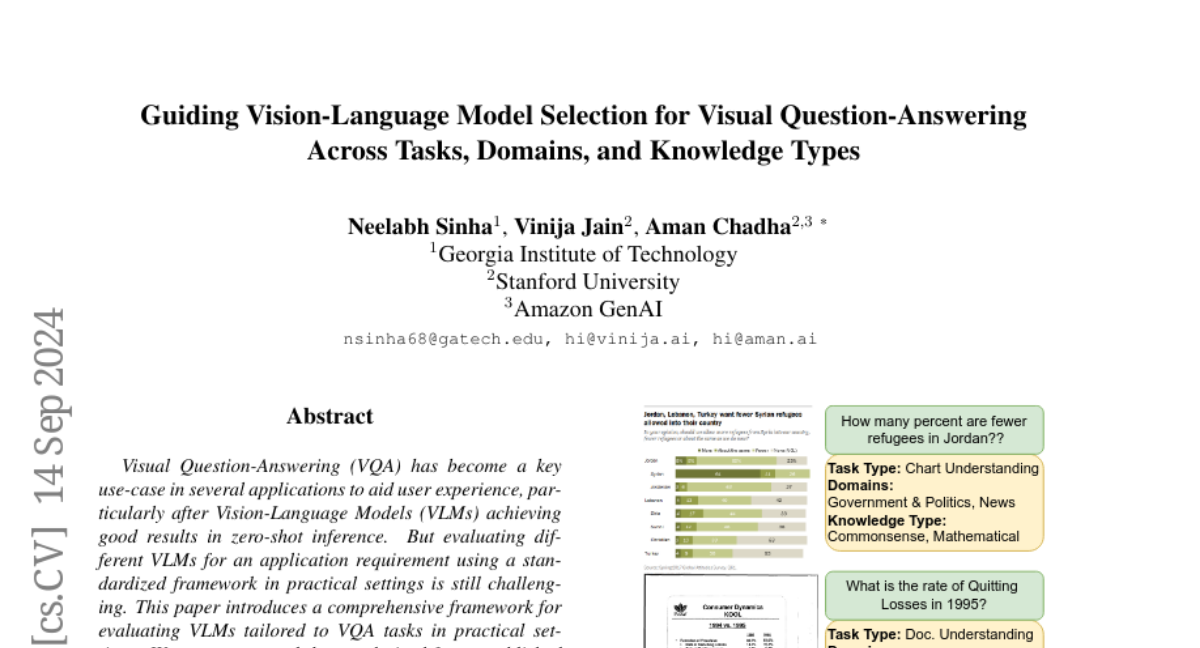
Summary
This paper discusses a new framework for evaluating Vision-Language Models (VLMs) used in Visual Question-Answering (VQA) tasks, helping to choose the best model for different applications.
What's the problem?
As VQA becomes more popular, it's important to evaluate which VLMs work best for specific tasks, but there isn't a standardized way to do this. Different models perform differently depending on the type of question and the context, making it hard to know which one to use in real-world situations.
What's the solution?
The authors introduce a structured framework that includes a new dataset annotated with task types, application domains, and knowledge types. They also create a new evaluation metric called GoEval that aligns closely with human judgments. By testing ten state-of-the-art VLMs, they find that no single model is the best for all tasks; instead, some proprietary models perform better overall, while certain open-source models excel in specific scenarios.
Why it matters?
This research is significant because it provides a clear method for selecting the right VLM based on specific needs and constraints. This can help improve user experiences in applications like customer service, education, and interactive media by ensuring that the most suitable model is used for answering visual questions.
Abstract
Visual Question-Answering (VQA) has become a key use-case in several applications to aid user experience, particularly after Vision-Language Models (VLMs) achieving good results in zero-shot inference. But evaluating different VLMs for an application requirement using a standardized framework in practical settings is still challenging. This paper introduces a comprehensive framework for evaluating VLMs tailored to VQA tasks in practical settings. We present a novel dataset derived from established VQA benchmarks, annotated with task types, application domains, and knowledge types, three key practical aspects on which tasks can vary. We also introduce GoEval, a multimodal evaluation metric developed using GPT-4o, achieving a correlation factor of 56.71% with human judgments. Our experiments with ten state-of-the-art VLMs reveals that no single model excelling universally, making appropriate selection a key design decision. Proprietary models such as Gemini-1.5-Pro and GPT-4o-mini generally outperform others, though open-source models like InternVL-2-8B and CogVLM-2-Llama-3-19B demonstrate competitive strengths in specific contexts, while providing additional advantages. This study guides the selection of VLMs based on specific task requirements and resource constraints, and can also be extended to other vision-language tasks.