Hackphyr: A Local Fine-Tuned LLM Agent for Network Security Environments
Maria Rigaki, Carlos Catania, Sebastian Garcia
2024-09-23
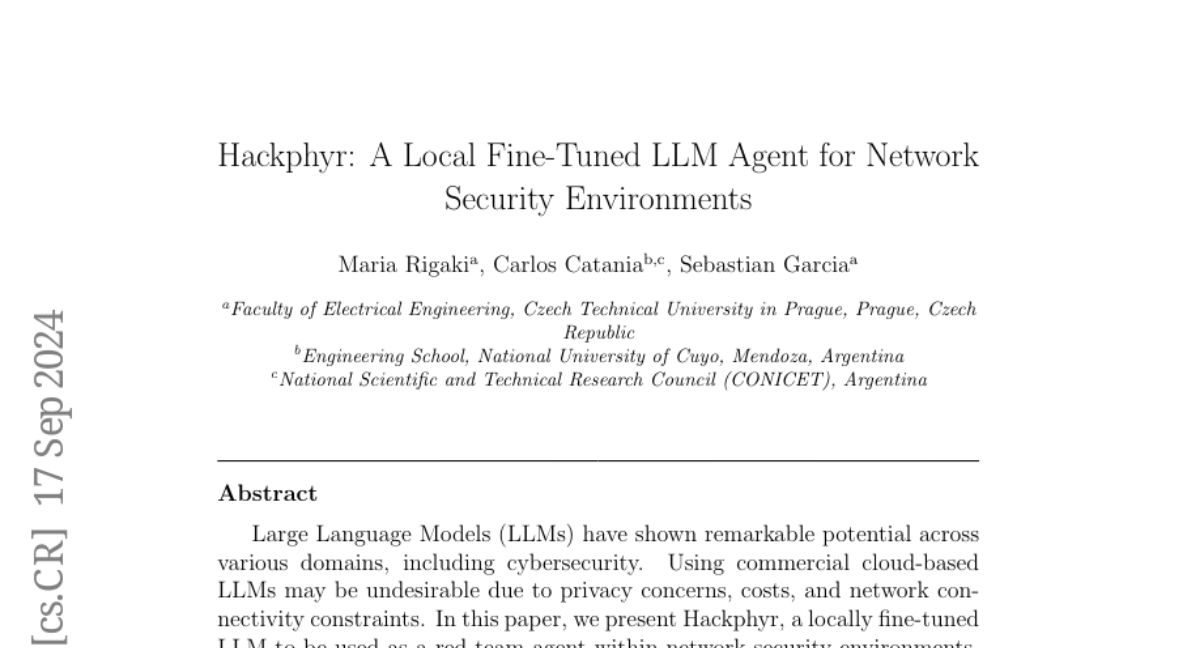
Summary
This paper discusses Hackphyr, a locally fine-tuned large language model (LLM) designed to enhance cybersecurity by acting as a red-team agent. It aims to provide a secure and efficient alternative to cloud-based models for network security tasks.
What's the problem?
Using commercial cloud-based LLMs for cybersecurity can raise issues like privacy concerns, high costs, and the need for constant internet connectivity. These factors make it challenging for organizations to rely on these models, especially when handling sensitive information about their networks.
What's the solution?
To address these challenges, the researchers developed Hackphyr, a 7 billion parameter model that can run on a single GPU. Hackphyr is specifically fine-tuned for cybersecurity tasks and has been shown to perform as well as larger commercial models like GPT-4. The researchers created a new cybersecurity dataset to improve the model's effectiveness in real-world scenarios. They also analyzed how well Hackphyr behaves in various situations, providing insights into its planning abilities and potential weaknesses.
Why it matters?
This research is important because it offers a locally deployed solution that enhances cybersecurity without compromising privacy or requiring expensive cloud services. By making advanced AI tools accessible for local use, Hackphyr can help organizations better protect their networks from cyber threats while maintaining control over their sensitive data.
Abstract
Large Language Models (LLMs) have shown remarkable potential across various domains, including cybersecurity. Using commercial cloud-based LLMs may be undesirable due to privacy concerns, costs, and network connectivity constraints. In this paper, we present Hackphyr, a locally fine-tuned LLM to be used as a red-team agent within network security environments. Our fine-tuned 7 billion parameter model can run on a single GPU card and achieves performance comparable with much larger and more powerful commercial models such as GPT-4. Hackphyr clearly outperforms other models, including GPT-3.5-turbo, and baselines, such as Q-learning agents in complex, previously unseen scenarios. To achieve this performance, we generated a new task-specific cybersecurity dataset to enhance the base model's capabilities. Finally, we conducted a comprehensive analysis of the agents' behaviors that provides insights into the planning abilities and potential shortcomings of such agents, contributing to the broader understanding of LLM-based agents in cybersecurity contexts