Harnessing Webpage UIs for Text-Rich Visual Understanding
Junpeng Liu, Tianyue Ou, Yifan Song, Yuxiao Qu, Wai Lam, Chenyan Xiong, Wenhu Chen, Graham Neubig, Xiang Yue
2024-10-18
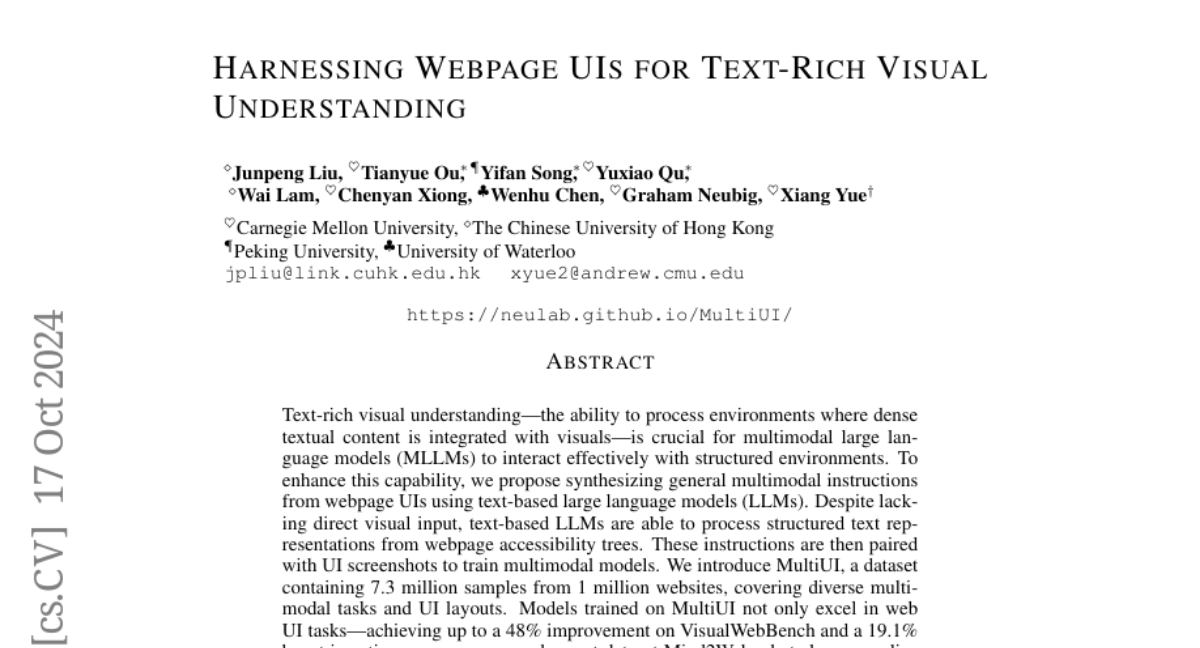
Summary
This paper discusses a new approach to help AI models better understand and interpret visual information that includes a lot of text, using data from webpages to improve their performance.
What's the problem?
AI models, especially those that combine visual and language understanding (called multimodal models), often struggle when it comes to environments where images contain a lot of text. This is particularly challenging for models that need to interact with structured environments like websites, where text and visuals are closely integrated. Current methods do not effectively teach these models how to process this type of information.
What's the solution?
To solve this problem, the authors created a dataset called MultiUI, which includes 7.3 million examples taken from 1 million different websites. This dataset contains pairs of text and images that represent various user interface (UI) layouts and tasks. By training AI models on this data, they can learn to understand complex visual information that includes text. The authors found that models trained with MultiUI showed significant improvements in tasks related to web UIs and even performed well in other areas, such as document understanding and interpreting charts.
Why it matters?
This research is important because it enhances the ability of AI systems to process and understand real-world environments where text and visuals are combined. By improving how these models work with text-rich visual content, the findings can lead to better AI applications in areas like web browsing, virtual assistants, and information retrieval, making technology more effective and user-friendly.
Abstract
Text-rich visual understanding-the ability to process environments where dense textual content is integrated with visuals-is crucial for multimodal large language models (MLLMs) to interact effectively with structured environments. To enhance this capability, we propose synthesizing general multimodal instructions from webpage UIs using text-based large language models (LLMs). Despite lacking direct visual input, text-based LLMs are able to process structured text representations from webpage accessibility trees. These instructions are then paired with UI screenshots to train multimodal models. We introduce MultiUI, a dataset containing 7.3 million samples from 1 million websites, covering diverse multimodal tasks and UI layouts. Models trained on MultiUI not only excel in web UI tasks-achieving up to a 48\% improvement on VisualWebBench and a 19.1\% boost in action accuracy on a web agent dataset Mind2Web-but also generalize surprisingly well to non-web UI tasks and even to non-UI domains, such as document understanding, OCR, and chart interpretation. These results highlight the broad applicability of web UI data for advancing text-rich visual understanding across various scenarios.