Harvesting Textual and Structured Data from the HAL Publication Repository
Francis Kulumba, Wissam Antoun, Guillaume Vimont, Laurent Romary
2024-07-31
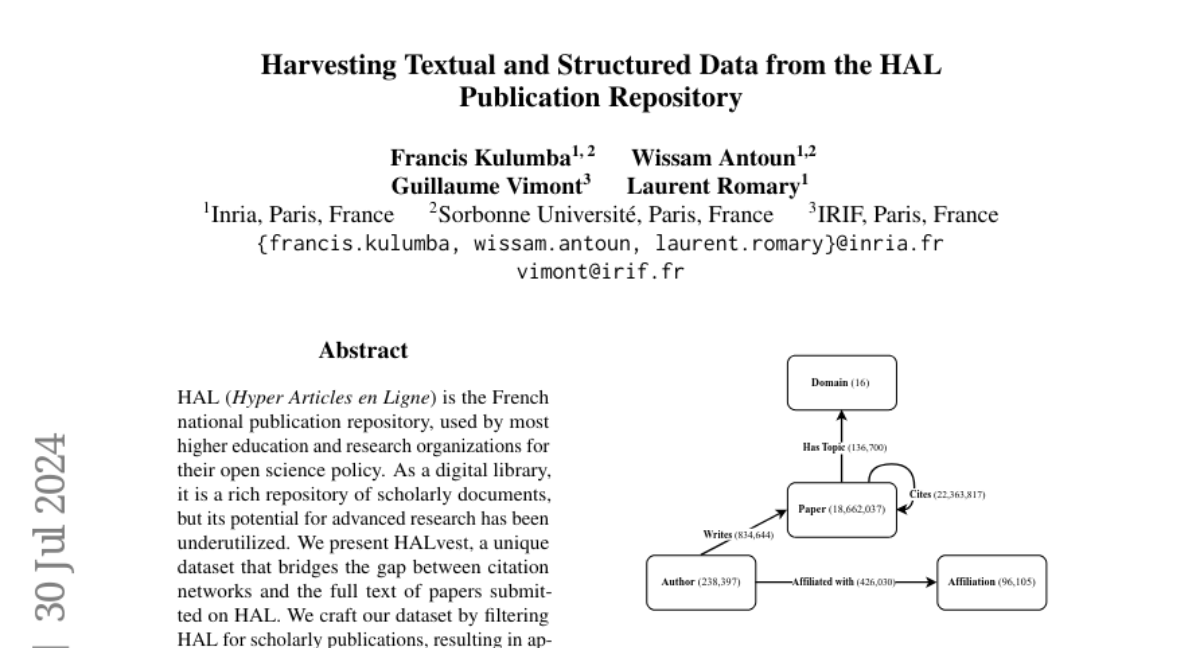
Summary
This paper discusses the creation of HALvest, a unique dataset derived from the HAL publication repository, which connects citation networks with the full texts of scholarly papers. It aims to enhance research by providing a rich resource for training language models.
What's the problem?
The HAL repository is a valuable source of scholarly documents, but its potential for advanced research has not been fully utilized. Researchers often struggle to access structured data that links citations to the actual content of papers, making it difficult to analyze and leverage this information effectively.
What's the solution?
To address this issue, the authors developed HALvest, a dataset that includes around 700,000 scholarly documents in 34 languages across 13 different fields. They transformed the metadata of these papers into a citation network, creating a directed graph that connects authors and their works. This dataset can be used for various tasks, including training language models and analyzing citation patterns. The authors also provide baseline methods for authorship attribution and demonstrate the effectiveness of their knowledge graph structure.
Why it matters?
This research is important because it opens up new possibilities for utilizing the wealth of information in the HAL repository. By providing a structured dataset that connects citations to full texts, HALvest can help researchers better understand academic relationships and trends. It also supports advancements in natural language processing and machine learning by offering high-quality data for model training, ultimately contributing to more efficient research practices.
Abstract
HAL (Hyper Articles en Ligne) is the French national publication repository, used by most higher education and research organizations for their open science policy. As a digital library, it is a rich repository of scholarly documents, but its potential for advanced research has been underutilized. We present HALvest, a unique dataset that bridges the gap between citation networks and the full text of papers submitted on HAL. We craft our dataset by filtering HAL for scholarly publications, resulting in approximately 700,000 documents, spanning 34 languages across 13 identified domains, suitable for language model training, and yielding approximately 16.5 billion tokens (with 8 billion in French and 7 billion in English, the most represented languages). We transform the metadata of each paper into a citation network, producing a directed heterogeneous graph. This graph includes uniquely identified authors on HAL, as well as all open submitted papers, and their citations. We provide a baseline for authorship attribution using the dataset, implement a range of state-of-the-art models in graph representation learning for link prediction, and discuss the usefulness of our generated knowledge graph structure.