HelloBench: Evaluating Long Text Generation Capabilities of Large Language Models
Haoran Que, Feiyu Duan, Liqun He, Yutao Mou, Wangchunshu Zhou, Jiaheng Liu, Wenge Rong, Zekun Moore Wang, Jian Yang, Ge Zhang, Junran Peng, Zhaoxiang Zhang, Songyang Zhang, Kai Chen
2024-09-25
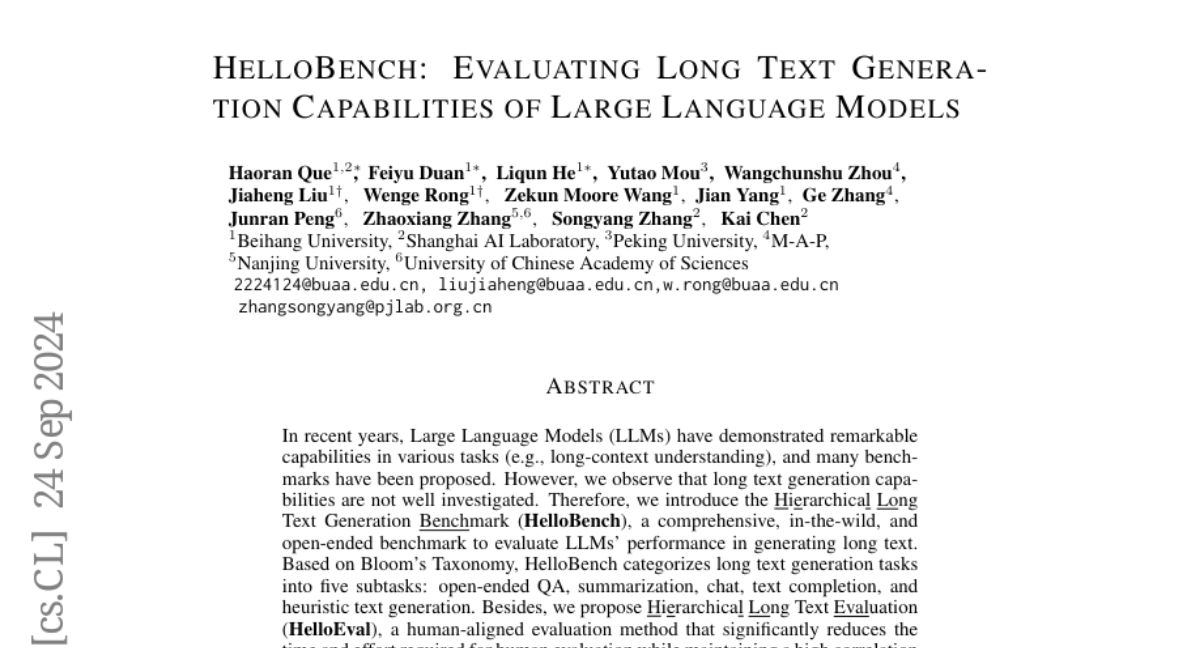
Summary
This paper introduces HelloBench, a new benchmark designed to evaluate how well large language models (LLMs) can generate long texts. It aims to assess their performance across various tasks and improve understanding of their capabilities in producing coherent and contextually relevant content.
What's the problem?
While LLMs have demonstrated impressive skills in generating short texts, their ability to create long, complex pieces of writing has not been thoroughly examined. Many existing benchmarks do not adequately test this capability, leaving a gap in understanding how these models perform with longer content. Additionally, current models struggle with maintaining quality and coherence over extended texts, which is essential for many applications.
What's the solution?
To address this issue, the researchers developed HelloBench, which categorizes long text generation tasks into five specific subtasks: open-ended question answering, summarization, chatting, text completion, and heuristic text generation. They also introduced HelloEval, a new evaluation method that aligns closely with human judgment while being efficient to use. Through extensive testing on around 30 different LLMs, they found that many models could not generate texts longer than 4,000 words without issues like repetition and decreased quality. HelloEval was shown to correlate better with human evaluations compared to traditional metrics.
Why it matters?
This research is important because it sets a new standard for evaluating the long text generation capabilities of LLMs. By providing a comprehensive benchmark and a reliable evaluation method, HelloBench can help researchers and developers identify strengths and weaknesses in current models. This understanding is crucial for improving LLMs, which can lead to better applications in fields like content creation, automated reporting, and more advanced conversational agents.
Abstract
In recent years, Large Language Models (LLMs) have demonstrated remarkable capabilities in various tasks (e.g., long-context understanding), and many benchmarks have been proposed. However, we observe that long text generation capabilities are not well investigated. Therefore, we introduce the Hierarchical Long Text Generation Benchmark (HelloBench), a comprehensive, in-the-wild, and open-ended benchmark to evaluate LLMs' performance in generating long text. Based on Bloom's Taxonomy, HelloBench categorizes long text generation tasks into five subtasks: open-ended QA, summarization, chat, text completion, and heuristic text generation. Besides, we propose Hierarchical Long Text Evaluation (HelloEval), a human-aligned evaluation method that significantly reduces the time and effort required for human evaluation while maintaining a high correlation with human evaluation. We have conducted extensive experiments across around 30 mainstream LLMs and observed that the current LLMs lack long text generation capabilities. Specifically, first, regardless of whether the instructions include explicit or implicit length constraints, we observe that most LLMs cannot generate text that is longer than 4000 words. Second, we observe that while some LLMs can generate longer text, many issues exist (e.g., severe repetition and quality degradation). Third, to demonstrate the effectiveness of HelloEval, we compare HelloEval with traditional metrics (e.g., ROUGE, BLEU, etc.) and LLM-as-a-Judge methods, which show that HelloEval has the highest correlation with human evaluation. We release our code in https://github.com/Quehry/HelloBench.