HelpSteer2: Open-source dataset for training top-performing reward models
Zhilin Wang, Yi Dong, Olivier Delalleau, Jiaqi Zeng, Gerald Shen, Daniel Egert, Jimmy J. Zhang, Makesh Narsimhan Sreedhar, Oleksii Kuchaiev
2024-06-14
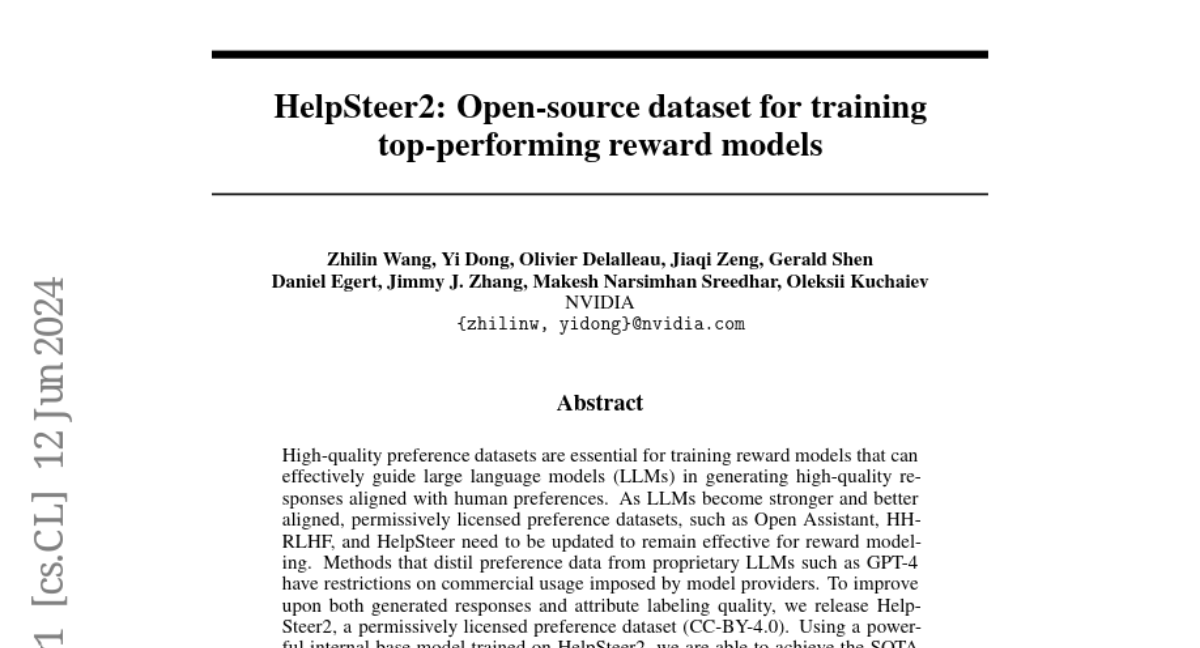
Summary
This paper introduces HelpSteer2, an open-source dataset designed to help train reward models that guide large language models (LLMs) in producing high-quality and helpful responses. The dataset aims to improve how LLMs align with human preferences.
What's the problem?
As LLMs become more advanced, there is a growing need for high-quality datasets that can effectively teach these models what kinds of responses are desirable. Existing datasets often have limitations, such as being outdated or having restrictions on their use. This makes it difficult to train models that can generate responses aligned with what people actually want.
What's the solution?
HelpSteer2 addresses these issues by providing a new dataset that includes ten thousand response pairs, which is fewer than many existing datasets but highly efficient for training. This dataset is openly licensed, meaning it can be used freely for research and development. The authors also developed a new model alignment approach called SteerLM 2.0, which utilizes the detailed scores from the reward models trained on HelpSteer2 to improve LLM performance. Their experiments show that using this dataset leads to better alignment with human preferences and higher quality outputs.
Why it matters?
This research is important because it contributes to the development of better AI systems that can understand and respond to human needs more effectively. By providing an open-source dataset and a new training approach, HelpSteer2 encourages further innovation in the field of AI, making it easier for researchers and developers to create models that are more helpful and aligned with user expectations.
Abstract
High-quality preference datasets are essential for training reward models that can effectively guide large language models (LLMs) in generating high-quality responses aligned with human preferences. As LLMs become stronger and better aligned, permissively licensed preference datasets, such as Open Assistant, HH-RLHF, and HelpSteer need to be updated to remain effective for reward modeling. Methods that distil preference data from proprietary LLMs such as GPT-4 have restrictions on commercial usage imposed by model providers. To improve upon both generated responses and attribute labeling quality, we release HelpSteer2, a permissively licensed preference dataset (CC-BY-4.0). Using a powerful internal base model trained on HelpSteer2, we are able to achieve the SOTA score (92.0%) on Reward-Bench's primary dataset, outperforming currently listed open and proprietary models, as of June 12th, 2024. Notably, HelpSteer2 consists of only ten thousand response pairs, an order of magnitude fewer than existing preference datasets (e.g., HH-RLHF), which makes it highly efficient for training reward models. Our extensive experiments demonstrate that reward models trained with HelpSteer2 are effective in aligning LLMs. In particular, we propose SteerLM 2.0, a model alignment approach that can effectively make use of the rich multi-attribute score predicted by our reward models. HelpSteer2 is available at https://huggingface.co/datasets/nvidia/HelpSteer2 and code is available at https://github.com/NVIDIA/NeMo-Aligner