HelpSteer2-Preference: Complementing Ratings with Preferences
Zhilin Wang, Alexander Bukharin, Olivier Delalleau, Daniel Egert, Gerald Shen, Jiaqi Zeng, Oleksii Kuchaiev, Yi Dong
2024-10-03
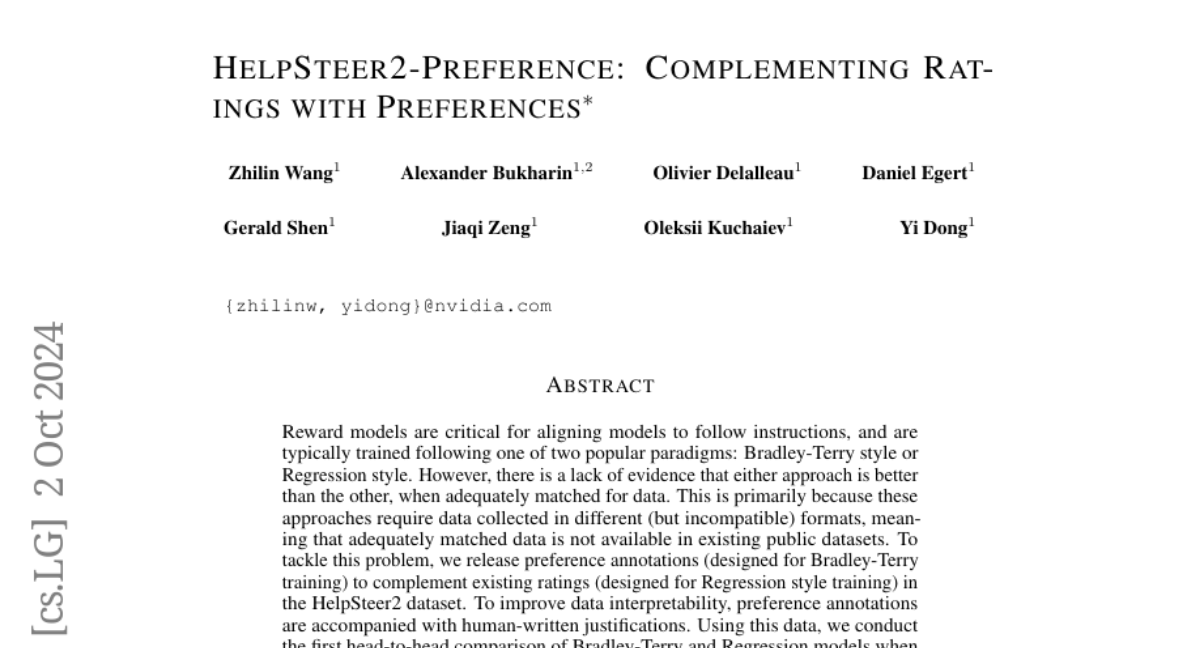
Summary
This paper discusses HelpSteer2-Preference, a new approach to improve reward models used in AI by combining different types of feedback data to enhance how well AI can follow instructions.
What's the problem?
Reward models are essential for training AI to understand and follow instructions, but there are two main methods to create these models: the Bradley-Terry style and the Regression style. However, there's no clear evidence showing which method works better because they require different types of data that don't match well. This makes it hard to compare their effectiveness directly.
What's the solution?
To solve this problem, the authors released a new dataset called HelpSteer2, which includes preference annotations designed for the Bradley-Terry model and complements existing ratings meant for the Regression model. They also included human-written justifications for these preferences to make the data easier to interpret. Using this dataset, they conducted a comparison of the two models and proposed a new method that combines both approaches. They fine-tuned a language model called Llama-3.1-70B-Instruct with this combined approach, resulting in a high performance score on benchmark tests.
Why it matters?
This research is important because it helps improve how AI systems learn from feedback, making them better at following human instructions. By understanding which methods work best for training these models, developers can create more effective AI applications that are reliable and user-friendly.
Abstract
Reward models are critical for aligning models to follow instructions, and are typically trained following one of two popular paradigms: Bradley-Terry style or Regression style. However, there is a lack of evidence that either approach is better than the other, when adequately matched for data. This is primarily because these approaches require data collected in different (but incompatible) formats, meaning that adequately matched data is not available in existing public datasets. To tackle this problem, we release preference annotations (designed for Bradley-Terry training) to complement existing ratings (designed for Regression style training) in the HelpSteer2 dataset. To improve data interpretability, preference annotations are accompanied with human-written justifications. Using this data, we conduct the first head-to-head comparison of Bradley-Terry and Regression models when adequately matched for data. Based on insights derived from such a comparison, we propose a novel approach to combine Bradley-Terry and Regression reward modeling. A Llama-3.1-70B-Instruct model tuned with this approach scores 94.1 on RewardBench, emerging top of more than 140 reward models as of 1 Oct 2024. We also demonstrate the effectiveness of this reward model at aligning models to follow instructions in RLHF. We open-source this dataset (CC-BY-4.0 license) at https://huggingface.co/datasets/nvidia/HelpSteer2 and openly release the trained Reward Model at https://huggingface.co/nvidia/Llama-3.1-Nemotron-70B-Reward