HEMM: Holistic Evaluation of Multimodal Foundation Models
Paul Pu Liang, Akshay Goindani, Talha Chafekar, Leena Mathur, Haofei Yu, Ruslan Salakhutdinov, Louis-Philippe Morency
2024-07-08
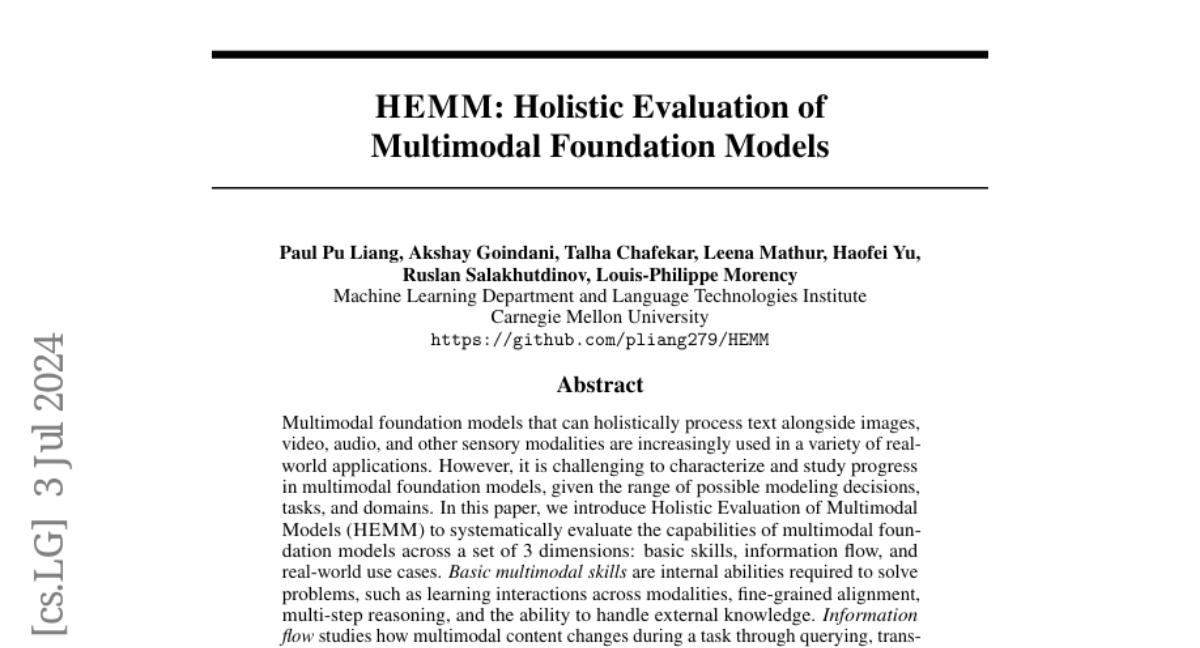
Summary
This paper talks about HEMM, a new framework designed to evaluate multimodal foundation models, which are AI systems that can understand and process multiple types of information like text, images, audio, and video.
What's the problem?
The main problem is that as these multimodal models become more common in real-world applications, it’s difficult to measure their progress and effectiveness. There are many different ways to design these models, and they can be used for various tasks, making it challenging to assess their capabilities consistently.
What's the solution?
To solve this issue, the authors introduce HEMM, which evaluates multimodal models based on three key areas: basic skills (like understanding how different types of data interact), information flow (how information changes during tasks), and real-world use cases (how well the models perform in specific applications). They conducted experiments using 30 different tasks to identify what challenges these models face and how different design choices affect their performance.
Why it matters?
This research is important because it provides a structured way to assess the abilities of multimodal foundation models. By understanding how well these models work across various tasks and domains, researchers can improve their designs and applications in fields like healthcare, education, and human-computer interaction. This ultimately leads to better AI systems that can help solve real-world problems.
Abstract
Multimodal foundation models that can holistically process text alongside images, video, audio, and other sensory modalities are increasingly used in a variety of real-world applications. However, it is challenging to characterize and study progress in multimodal foundation models, given the range of possible modeling decisions, tasks, and domains. In this paper, we introduce Holistic Evaluation of Multimodal Models (HEMM) to systematically evaluate the capabilities of multimodal foundation models across a set of 3 dimensions: basic skills, information flow, and real-world use cases. Basic multimodal skills are internal abilities required to solve problems, such as learning interactions across modalities, fine-grained alignment, multi-step reasoning, and the ability to handle external knowledge. Information flow studies how multimodal content changes during a task through querying, translation, editing, and fusion. Use cases span domain-specific challenges introduced in real-world multimedia, affective computing, natural sciences, healthcare, and human-computer interaction applications. Through comprehensive experiments across the 30 tasks in HEMM, we (1) identify key dataset dimensions (e.g., basic skills, information flows, and use cases) that pose challenges to today's models, and (2) distill performance trends regarding how different modeling dimensions (e.g., scale, pre-training data, multimodal alignment, pre-training, and instruction tuning objectives) influence performance. Our conclusions regarding challenging multimodal interactions, use cases, and tasks requiring reasoning and external knowledge, the benefits of data and model scale, and the impacts of instruction tuning yield actionable insights for future work in multimodal foundation models.