Hi3D: Pursuing High-Resolution Image-to-3D Generation with Video Diffusion Models
Haibo Yang, Yang Chen, Yingwei Pan, Ting Yao, Zhineng Chen, Chong-Wah Ngo, Tao Mei
2024-09-12
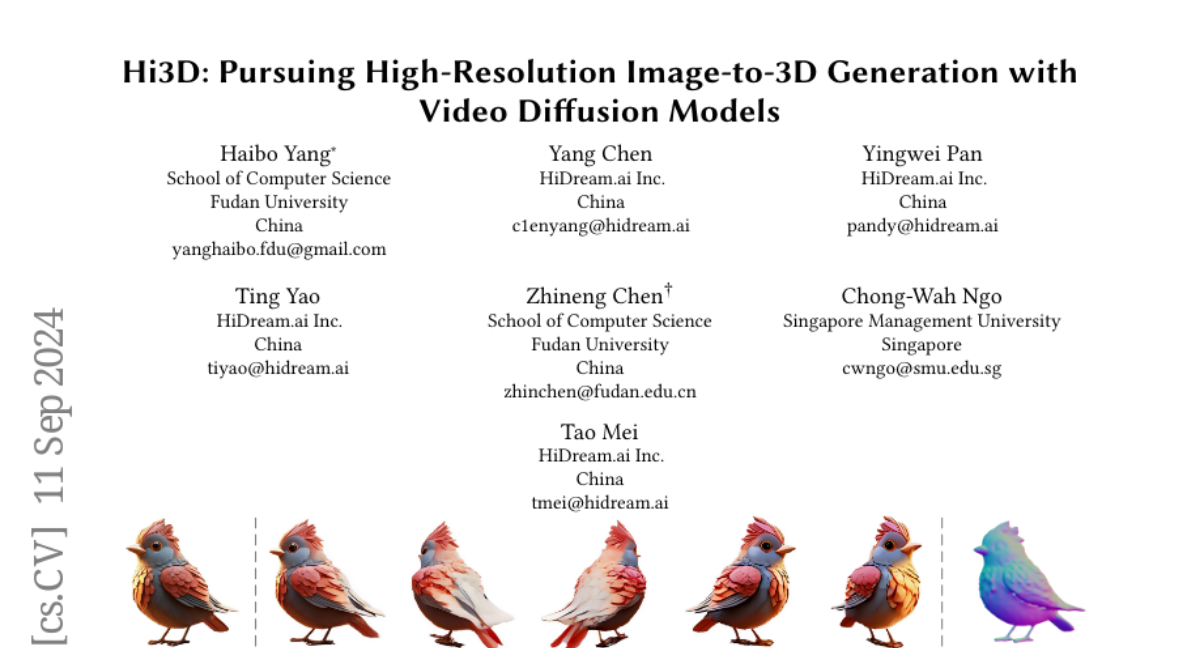
Summary
This paper talks about Hi3D, a new method for generating high-quality 3D images from a single image using advanced video diffusion techniques.
What's the problem?
Although there has been significant progress in creating 3D images from 2D images, existing methods often struggle to produce consistent and detailed images from different viewpoints. Many of these methods do not effectively consider the three-dimensional nature of objects, leading to lower quality results.
What's the solution?
To solve this issue, the authors developed Hi3D, which uses a video diffusion model to transform a single image into multiple views that are aware of 3D structure. Hi3D first enhances a pre-trained video model with information about camera angles to create initial low-resolution images. Then, it uses a special process to refine these images into high-resolution versions. Additionally, it generates new views through a technique called 3D Gaussian Splatting and creates detailed 3D models through reconstruction.
Why it matters?
This research is important because it significantly improves the ability to generate realistic 3D images from simple 2D inputs. This advancement can be applied in various fields such as gaming, virtual reality, and animation, making it easier to create immersive experiences that require high-quality visual content.
Abstract
Despite having tremendous progress in image-to-3D generation, existing methods still struggle to produce multi-view consistent images with high-resolution textures in detail, especially in the paradigm of 2D diffusion that lacks 3D awareness. In this work, we present High-resolution Image-to-3D model (Hi3D), a new video diffusion based paradigm that redefines a single image to multi-view images as 3D-aware sequential image generation (i.e., orbital video generation). This methodology delves into the underlying temporal consistency knowledge in video diffusion model that generalizes well to geometry consistency across multiple views in 3D generation. Technically, Hi3D first empowers the pre-trained video diffusion model with 3D-aware prior (camera pose condition), yielding multi-view images with low-resolution texture details. A 3D-aware video-to-video refiner is learnt to further scale up the multi-view images with high-resolution texture details. Such high-resolution multi-view images are further augmented with novel views through 3D Gaussian Splatting, which are finally leveraged to obtain high-fidelity meshes via 3D reconstruction. Extensive experiments on both novel view synthesis and single view reconstruction demonstrate that our Hi3D manages to produce superior multi-view consistency images with highly-detailed textures. Source code and data are available at https://github.com/yanghb22-fdu/Hi3D-Official.