Hibou: A Family of Foundational Vision Transformers for Pathology
Dmitry Nechaev, Alexey Pchelnikov, Ekaterina Ivanova
2024-06-13
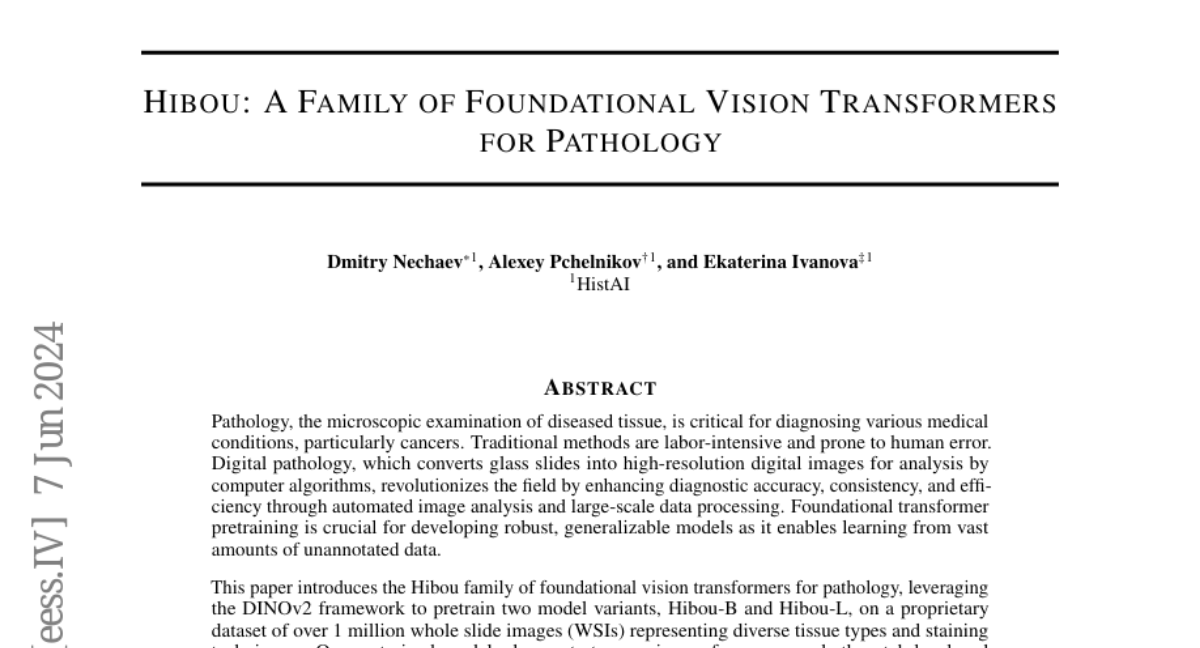
Summary
This paper introduces the Hibou family of vision transformers, which are advanced AI models designed to analyze medical images, particularly for diagnosing diseases like cancer. These models improve the accuracy and efficiency of pathology through automated analysis of digital images.
What's the problem?
Traditional pathology methods involve manually examining glass slides under a microscope, which can be time-consuming and prone to human error. This makes it challenging to diagnose conditions accurately and consistently. As technology advances, there is a need for better tools that can analyze medical images automatically and reliably.
What's the solution?
The authors developed the Hibou models using a framework called DINOv2 to pretrain two versions: Hibou-B and Hibou-L. They trained these models on a large dataset containing over 1 million high-resolution images of different tissue types and staining techniques. By doing this, the models learned to recognize patterns in the images more effectively. The results showed that Hibou models performed better than existing methods in various tests, including analyzing patches of tissue and entire slides.
Why it matters?
This research is important because it represents a significant step forward in digital pathology. By using AI models like Hibou, healthcare professionals can achieve faster and more accurate diagnoses, improving patient outcomes. The open-sourcing of the Hibou-B model also encourages further research and development in this field, helping to advance medical image analysis technology.
Abstract
Pathology, the microscopic examination of diseased tissue, is critical for diagnosing various medical conditions, particularly cancers. Traditional methods are labor-intensive and prone to human error. Digital pathology, which converts glass slides into high-resolution digital images for analysis by computer algorithms, revolutionizes the field by enhancing diagnostic accuracy, consistency, and efficiency through automated image analysis and large-scale data processing. Foundational transformer pretraining is crucial for developing robust, generalizable models as it enables learning from vast amounts of unannotated data. This paper introduces the Hibou family of foundational vision transformers for pathology, leveraging the DINOv2 framework to pretrain two model variants, Hibou-B and Hibou-L, on a proprietary dataset of over 1 million whole slide images (WSIs) representing diverse tissue types and staining techniques. Our pretrained models demonstrate superior performance on both patch-level and slide-level benchmarks, surpassing existing state-of-the-art methods. Notably, Hibou-L achieves the highest average accuracy across multiple benchmark datasets. To support further research and application in the field, we have open-sourced the Hibou-B model, which can be accessed at https://github.com/HistAI/hibou