Hierarchical Prompting Taxonomy: A Universal Evaluation Framework for Large Language Models
Devichand Budagam, Sankalp KJ, Ashutosh Kumar, Vinija Jain, Aman Chadha
2024-06-19
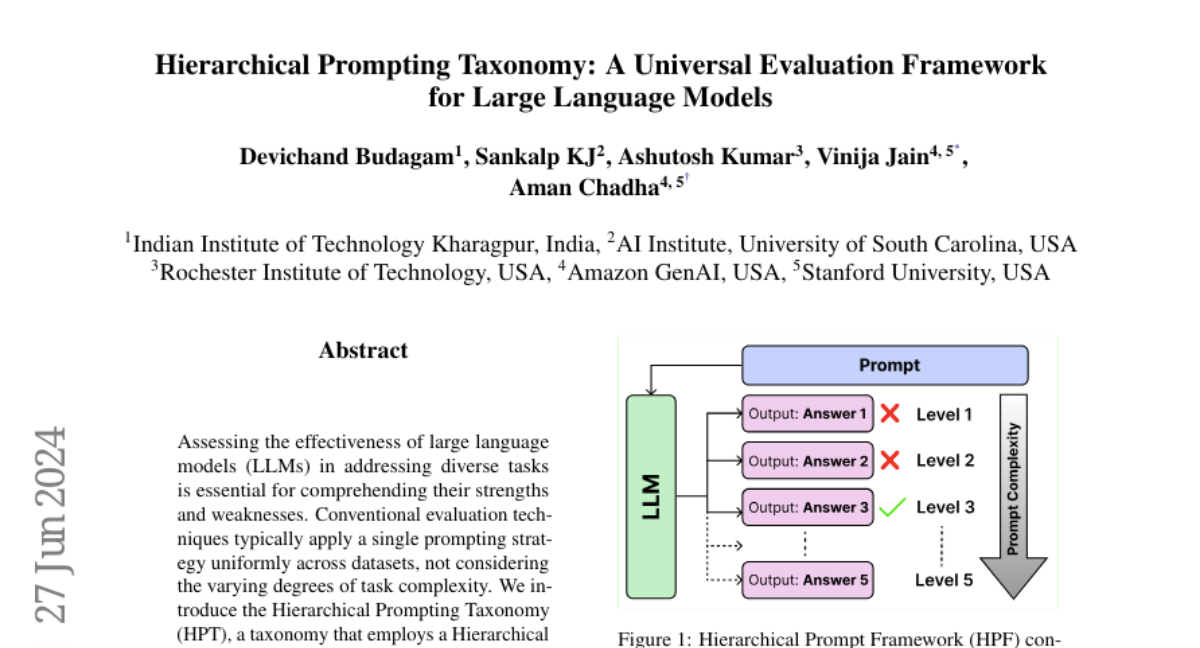
Summary
This paper introduces the Hierarchical Prompting Taxonomy (HPT), a new framework designed to evaluate how well large language models (LLMs) perform on various tasks. It organizes tasks into different levels of complexity to better understand the strengths and weaknesses of these models.
What's the problem?
Traditional methods for evaluating LLMs often use a one-size-fits-all approach, applying the same prompting strategy to all tasks regardless of their complexity. This can lead to inaccurate assessments because it doesn't account for how different tasks require different skills and levels of understanding. As a result, we may not get a clear picture of how capable a model really is.
What's the solution?
To solve this problem, the authors developed HPT, which categorizes tasks into a hierarchical structure with five levels, ranging from simple tasks like answering questions to more complex ones that involve reasoning or multi-step problem-solving. Each level has its own prompting strategy tailored to its complexity. The framework also includes an Adaptive Hierarchical Prompt system that automatically selects the best prompting strategy for each task. This allows for a more precise evaluation of LLMs based on how well they handle different types of challenges.
Why it matters?
This research is important because it provides a more effective way to evaluate language models, helping researchers and developers understand their capabilities better. By using HPT, we can identify areas where models excel and where they need improvement, ultimately leading to the development of more advanced and reliable AI systems that can perform a wide range of tasks.
Abstract
Assessing the effectiveness of large language models (LLMs) in addressing diverse tasks is essential for comprehending their strengths and weaknesses. Conventional evaluation techniques typically apply a single prompting strategy uniformly across datasets, not considering the varying degrees of task complexity. We introduce the Hierarchical Prompting Taxonomy (HPT), a taxonomy that employs a Hierarchical Prompt Framework (HPF) composed of five unique prompting strategies, arranged from the simplest to the most complex, to assess LLMs more precisely and to offer a clearer perspective. This taxonomy assigns a score, called the Hierarchical Prompting Score (HP-Score), to datasets as well as LLMs based on the rules of the taxonomy, providing a nuanced understanding of their ability to solve diverse tasks and offering a universal measure of task complexity. Additionally, we introduce the Adaptive Hierarchical Prompt framework, which automates the selection of appropriate prompting strategies for each task. This study compares manual and adaptive hierarchical prompt frameworks using four instruction-tuned LLMs, namely Llama 3 8B, Phi 3 3.8B, Mistral 7B, and Gemma 7B, across four datasets: BoolQ, CommonSenseQA (CSQA), IWSLT-2017 en-fr (IWSLT), and SamSum. Experiments demonstrate the effectiveness of HPT, providing a reliable way to compare different tasks and LLM capabilities. This paper leads to the development of a universal evaluation metric that can be used to evaluate both the complexity of the datasets and the capabilities of LLMs. The implementation of both manual HPF and adaptive HPF is publicly available.