Holistic Unlearning Benchmark: A Multi-Faceted Evaluation for Text-to-Image Diffusion Model Unlearning
Saemi Moon, Minjong Lee, Sangdon Park, Dongwoo Kim
2024-10-10
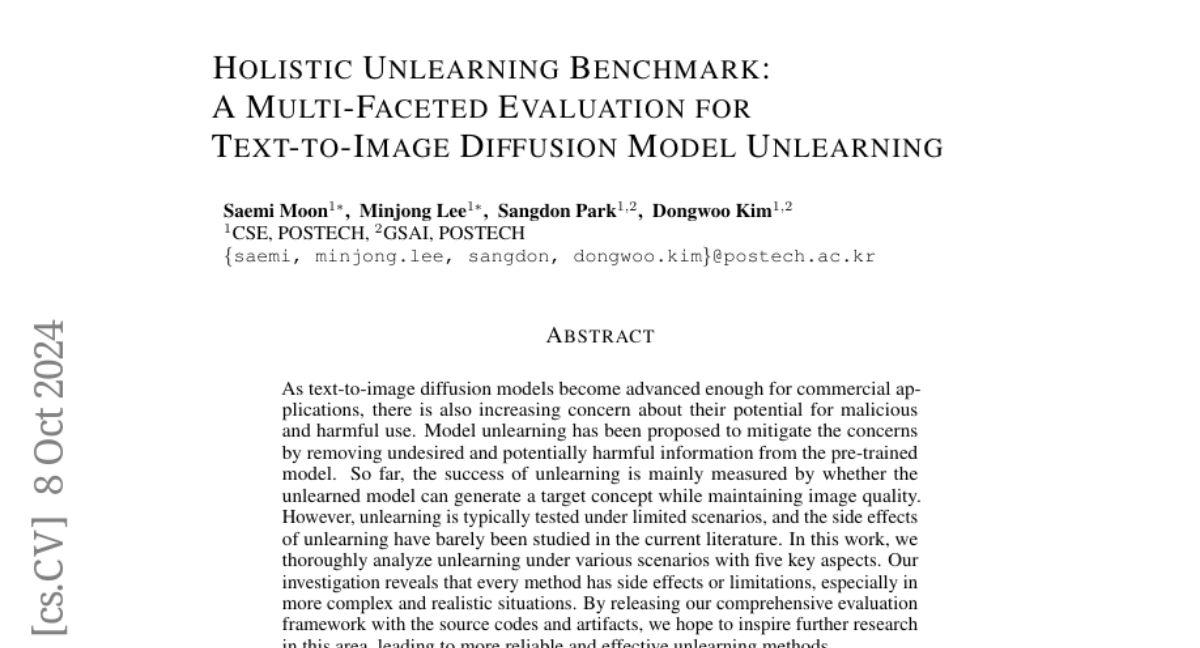
Summary
This paper introduces the Holistic Unlearning Benchmark, which evaluates how effectively text-to-image diffusion models can forget harmful or unwanted information.
What's the problem?
As text-to-image models become more popular for commercial use, there are growing concerns about their potential to generate harmful or inappropriate content. Current methods for 'unlearning'—removing unwanted information from these models—often only check if the model can still generate desired images without considering the side effects or limitations of the unlearning process.
What's the solution?
The authors propose a comprehensive evaluation framework that examines unlearning across various scenarios. They analyze five key aspects of unlearning and reveal that existing methods often have negative side effects, especially in complex situations. By releasing their evaluation framework along with source codes, they aim to inspire further research into developing more reliable unlearning methods.
Why it matters?
This research is important because it addresses a critical issue in AI safety and ethics. By improving how models can safely forget harmful information, the work contributes to creating safer AI systems that can be used responsibly in real-world applications, ultimately helping to prevent misuse of technology.
Abstract
As text-to-image diffusion models become advanced enough for commercial applications, there is also increasing concern about their potential for malicious and harmful use. Model unlearning has been proposed to mitigate the concerns by removing undesired and potentially harmful information from the pre-trained model. So far, the success of unlearning is mainly measured by whether the unlearned model can generate a target concept while maintaining image quality. However, unlearning is typically tested under limited scenarios, and the side effects of unlearning have barely been studied in the current literature. In this work, we thoroughly analyze unlearning under various scenarios with five key aspects. Our investigation reveals that every method has side effects or limitations, especially in more complex and realistic situations. By releasing our comprehensive evaluation framework with the source codes and artifacts, we hope to inspire further research in this area, leading to more reliable and effective unlearning methods.