HoloPart: Generative 3D Part Amodal Segmentation
Yunhan Yang, Yuan-Chen Guo, Yukun Huang, Zi-Xin Zou, Zhipeng Yu, Yangguang Li, Yan-Pei Cao, Xihui Liu
2025-04-11
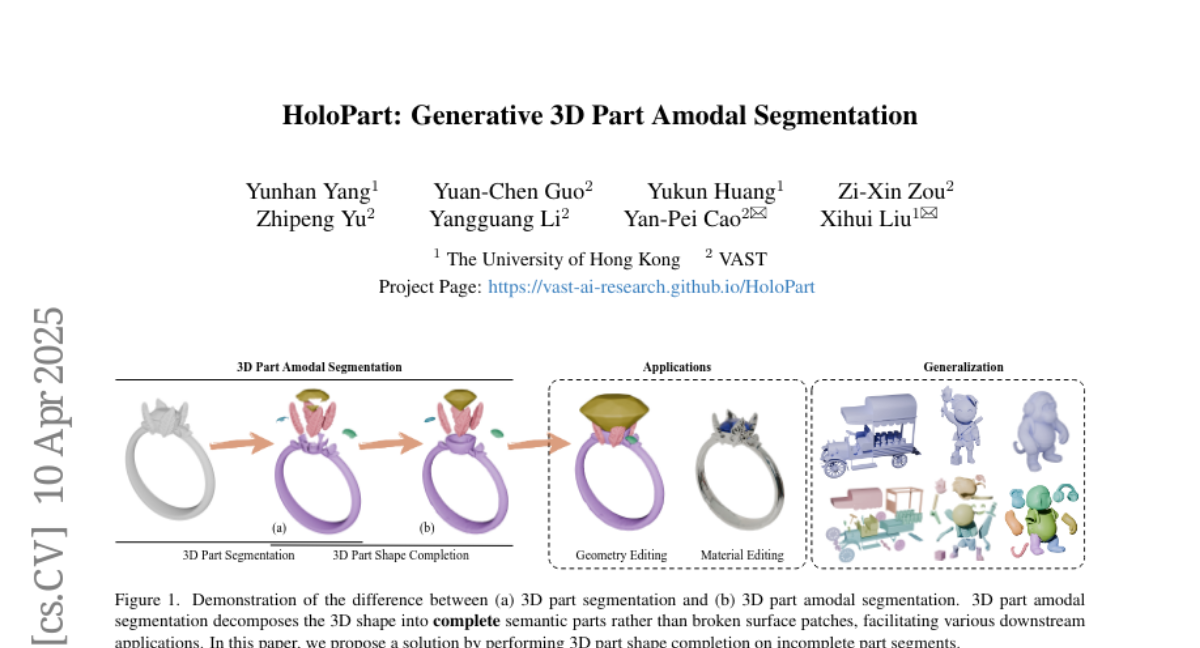
Summary
This paper talks about HoloPart, a tool that helps AI understand 3D objects by figuring out what their hidden or missing parts look like, like guessing the full shape of a bike wheel even if part of it is blocked by another object.
What's the problem?
Current 3D models can only identify the visible parts of objects, leaving out hidden sections, which makes editing or animating 3D models difficult since you can’t work with complete pieces.
What's the solution?
HoloPart uses a two-step process: first, it finds visible parts using existing tools, then it uses a smart AI model to ‘imagine’ and fill in the hidden parts while keeping the whole object’s shape consistent.
Why it matters?
This helps creators edit 3D models more easily, like adjusting individual parts of a car or animating a character’s limbs, even if parts are hidden, speeding up 3D design for games, movies, or VR.
Abstract
3D part amodal segmentation--decomposing a 3D shape into complete, semantically meaningful parts, even when occluded--is a challenging but crucial task for 3D content creation and understanding. Existing 3D part segmentation methods only identify visible surface patches, limiting their utility. Inspired by 2D amodal segmentation, we introduce this novel task to the 3D domain and propose a practical, two-stage approach, addressing the key challenges of inferring occluded 3D geometry, maintaining global shape consistency, and handling diverse shapes with limited training data. First, we leverage existing 3D part segmentation to obtain initial, incomplete part segments. Second, we introduce HoloPart, a novel diffusion-based model, to complete these segments into full 3D parts. HoloPart utilizes a specialized architecture with local attention to capture fine-grained part geometry and global shape context attention to ensure overall shape consistency. We introduce new benchmarks based on the ABO and PartObjaverse-Tiny datasets and demonstrate that HoloPart significantly outperforms state-of-the-art shape completion methods. By incorporating HoloPart with existing segmentation techniques, we achieve promising results on 3D part amodal segmentation, opening new avenues for applications in geometry editing, animation, and material assignment.