How to Synthesize Text Data without Model Collapse?
Xuekai Zhu, Daixuan Cheng, Hengli Li, Kaiyan Zhang, Ermo Hua, Xingtai Lv, Ning Ding, Zhouhan Lin, Zilong Zheng, Bowen Zhou
2024-12-20
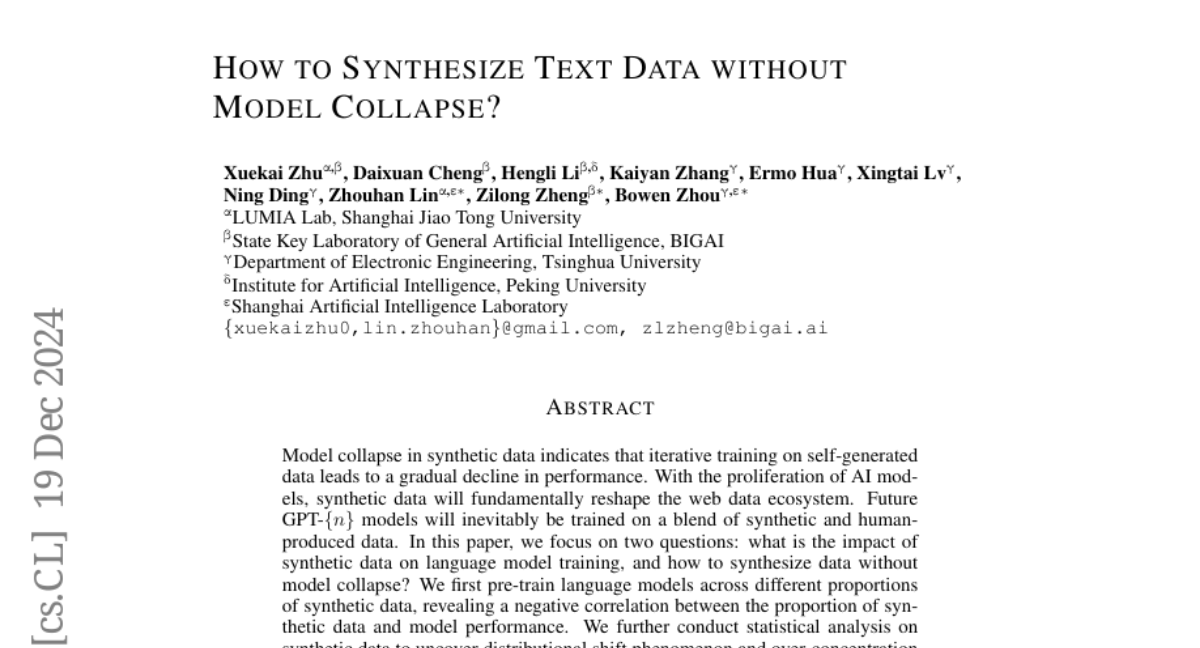
Summary
This paper discusses how to create synthetic text data for training language models without causing a problem known as model collapse, where the model's performance decreases over time due to reliance on its own generated data.
What's the problem?
As AI models increasingly use synthetic data (data generated by other AI), there's a risk that they will start to perform worse because they keep training on their own outputs, which can lead to repetitive and low-quality results. This is called model collapse, and it makes the models less effective.
What's the solution?
The authors propose a method called token editing, which involves making small changes to real human-produced data to create semi-synthetic data. This approach helps maintain the quality of the training data and prevents model collapse. They conducted experiments showing that this method improves the performance of language models by ensuring that the training data remains diverse and high-quality.
Why it matters?
This research is important because it helps improve how AI models learn from both synthetic and human-generated data. By preventing model collapse, it ensures that future AI systems can continue to improve and adapt without losing their effectiveness, which is crucial for applications like chatbots, translation services, and more.
Abstract
Model collapse in synthetic data indicates that iterative training on self-generated data leads to a gradual decline in performance. With the proliferation of AI models, synthetic data will fundamentally reshape the web data ecosystem. Future GPT-{n} models will inevitably be trained on a blend of synthetic and human-produced data. In this paper, we focus on two questions: what is the impact of synthetic data on language model training, and how to synthesize data without model collapse? We first pre-train language models across different proportions of synthetic data, revealing a negative correlation between the proportion of synthetic data and model performance. We further conduct statistical analysis on synthetic data to uncover distributional shift phenomenon and over-concentration of n-gram features. Inspired by the above findings, we propose token editing on human-produced data to obtain semi-synthetic data. As a proof of concept, we theoretically demonstrate that token-level editing can prevent model collapse, as the test error is constrained by a finite upper bound. We conduct extensive experiments on pre-training from scratch, continual pre-training, and supervised fine-tuning. The results validate our theoretical proof that token-level editing improves data quality and enhances model performance.