HumanSplat: Generalizable Single-Image Human Gaussian Splatting with Structure Priors
Panwang Pan, Zhuo Su, Chenguo Lin, Zhen Fan, Yongjie Zhang, Zeming Li, Tingting Shen, Yadong Mu, Yebin Liu
2024-06-19
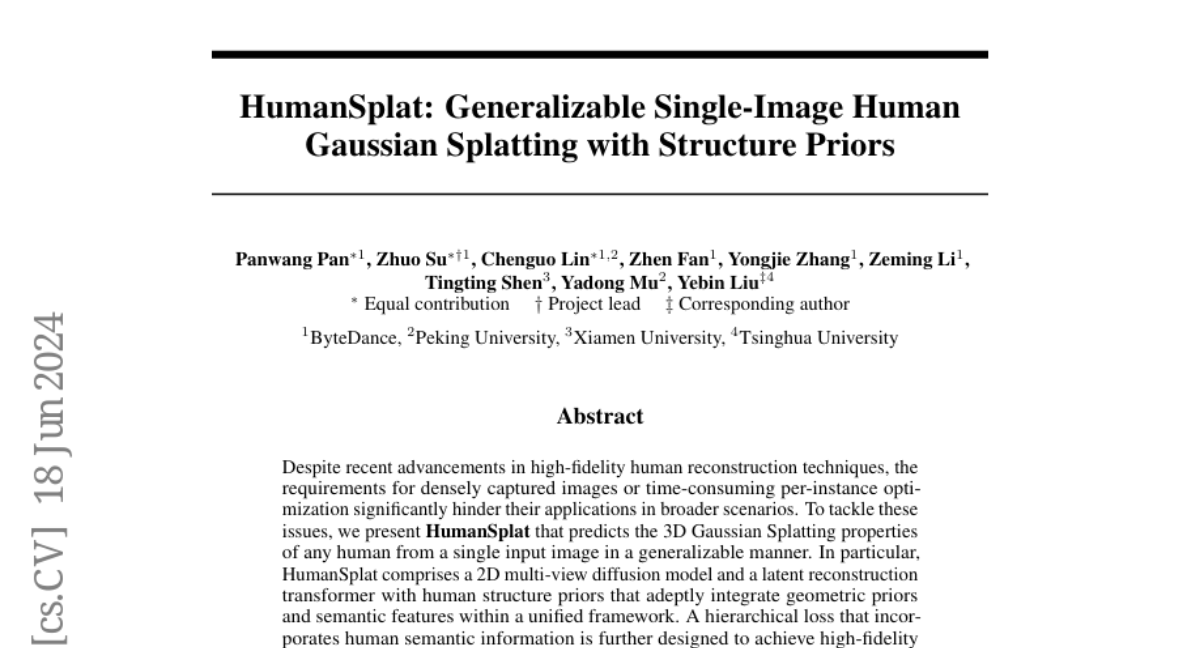
Summary
This paper presents HumanSplat, a new method for creating 3D models of people from just one photo. It uses advanced techniques to accurately predict how a person looks in three dimensions, even when only given a single image.
What's the problem?
Many current methods for making 3D models of humans require multiple images or a lot of time to optimize each model individually. This makes it hard to use these techniques in everyday situations where you might only have one photo. As a result, creating realistic 3D representations of people can be difficult and time-consuming.
What's the solution?
To solve this problem, the authors developed HumanSplat, which can generate 3D models from a single image by using a combination of a 2D multi-view diffusion model and a latent reconstruction transformer. This approach incorporates knowledge about human anatomy (like the typical shapes and sizes of body parts) to improve the accuracy of the 3D models. The method also includes a special loss function that helps the model focus on important details, resulting in high-quality textures and better overall models. The authors tested HumanSplat against other methods and found that it produces more realistic images from single photos than previous techniques.
Why it matters?
This research is important because it makes it easier to create realistic 3D representations of people using just one image, which has many practical applications. For example, this technology could be used in video games, virtual reality, online shopping (for virtual try-ons), and more. By improving how we can reconstruct human figures from simple photos, HumanSplat could change how we interact with digital content and enhance user experiences in various fields.
Abstract
Despite recent advancements in high-fidelity human reconstruction techniques, the requirements for densely captured images or time-consuming per-instance optimization significantly hinder their applications in broader scenarios. To tackle these issues, we present HumanSplat which predicts the 3D Gaussian Splatting properties of any human from a single input image in a generalizable manner. In particular, HumanSplat comprises a 2D multi-view diffusion model and a latent reconstruction transformer with human structure priors that adeptly integrate geometric priors and semantic features within a unified framework. A hierarchical loss that incorporates human semantic information is further designed to achieve high-fidelity texture modeling and better constrain the estimated multiple views. Comprehensive experiments on standard benchmarks and in-the-wild images demonstrate that HumanSplat surpasses existing state-of-the-art methods in achieving photorealistic novel-view synthesis.