IDEAW: Robust Neural Audio Watermarking with Invertible Dual-Embedding
Pengcheng Li, Xulong Zhang, Jing Xiao, Jianzong Wang
2024-10-01
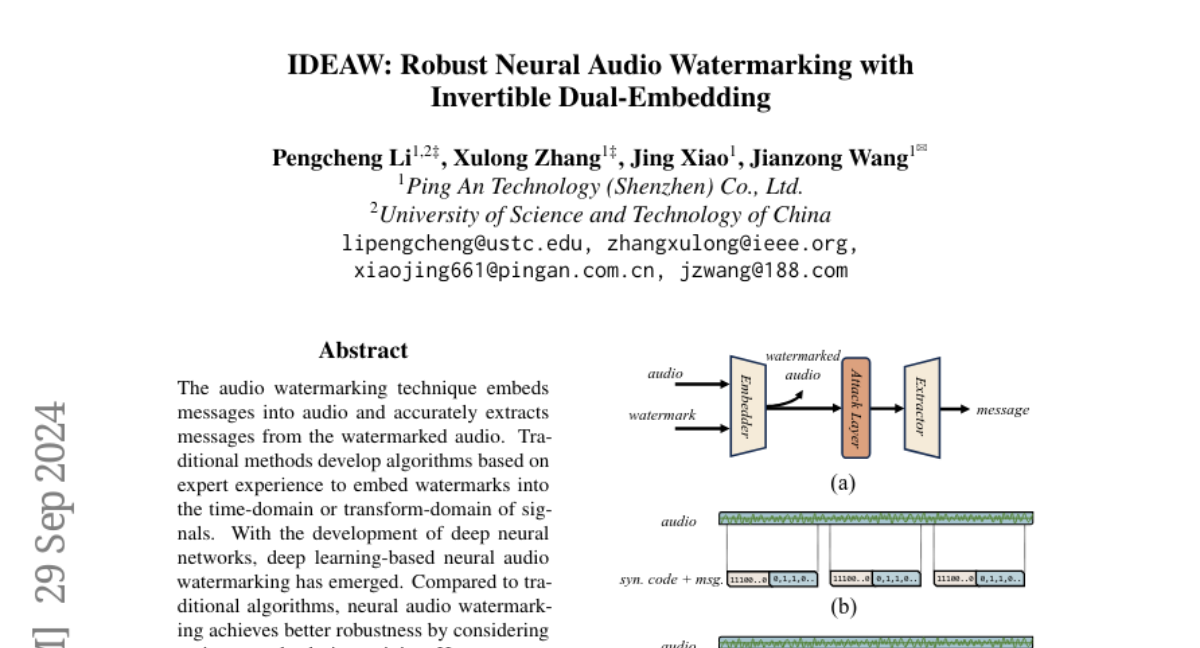
Summary
This paper presents IDEAW, a new method for audio watermarking that uses a dual-embedding approach to embed and extract messages from audio files while ensuring robustness against attacks.
What's the problem?
Traditional audio watermarking techniques often struggle with issues like low capacity (the amount of information they can embed) and poor imperceptibility (how noticeable the watermark is in the audio). Additionally, current methods do not effectively address the challenge of locating the watermark within the audio, which is crucial for retrieving the embedded information.
What's the solution?
The authors developed IDEAW, which uses a dual-embedding model to separately embed a message and a locating code into the audio. This model helps improve the ability to find the watermark while also allowing for more information to be embedded. They also introduced a balance block in their training process to enhance stability and robustness against attacks. The results showed that IDEAW can withstand various types of attacks better than existing methods, while also having higher capacity and more efficient locating capabilities.
Why it matters?
This research is significant because it enhances the effectiveness of audio watermarking, making it more reliable for protecting intellectual property in digital media. By improving how watermarks are embedded and extracted, IDEAW can help ensure that audio content remains secure and original, which is increasingly important in today's digital landscape.
Abstract
The audio watermarking technique embeds messages into audio and accurately extracts messages from the watermarked audio. Traditional methods develop algorithms based on expert experience to embed watermarks into the time-domain or transform-domain of signals. With the development of deep neural networks, deep learning-based neural audio watermarking has emerged. Compared to traditional algorithms, neural audio watermarking achieves better robustness by considering various attacks during training. However, current neural watermarking methods suffer from low capacity and unsatisfactory imperceptibility. Additionally, the issue of watermark locating, which is extremely important and even more pronounced in neural audio watermarking, has not been adequately studied. In this paper, we design a dual-embedding watermarking model for efficient locating. We also consider the impact of the attack layer on the invertible neural network in robustness training, improving the model to enhance both its reasonableness and stability. Experiments show that the proposed model, IDEAW, can withstand various attacks with higher capacity and more efficient locating ability compared to existing methods.