IFAdapter: Instance Feature Control for Grounded Text-to-Image Generation
Yinwei Wu, Xianpan Zhou, Bing Ma, Xuefeng Su, Kai Ma, Xinchao Wang
2024-09-13
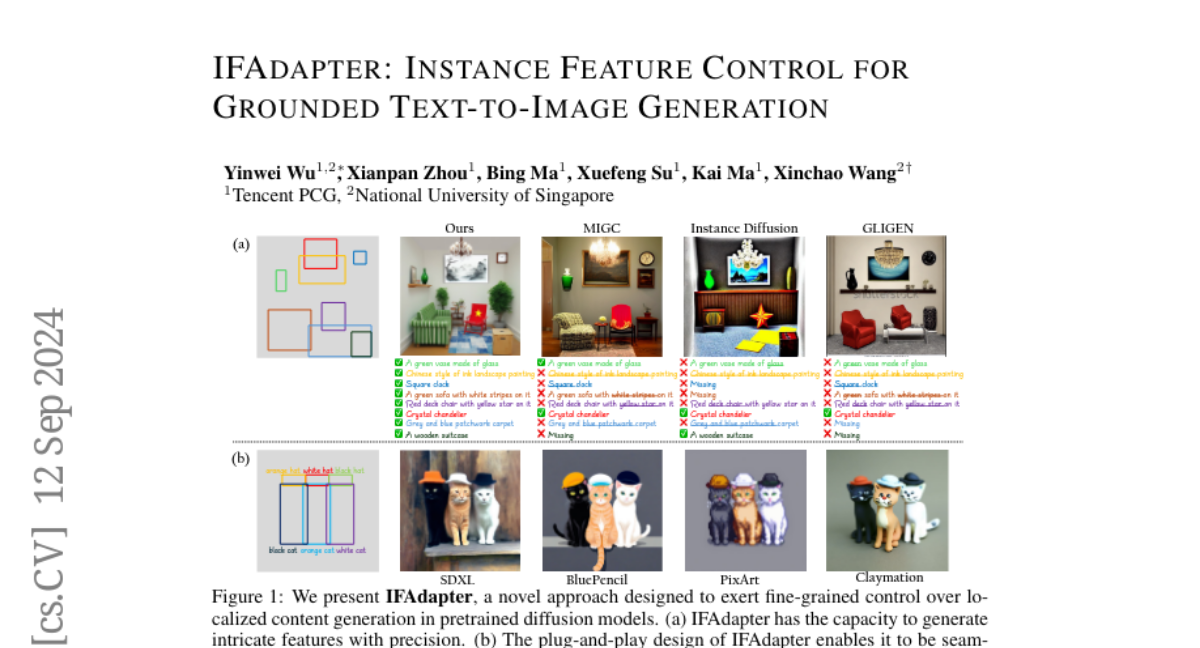
Summary
This paper introduces IFAdapter, a new method for improving how AI generates images from text descriptions, focusing on making sure that multiple objects in an image are accurately positioned and depicted.
What's the problem?
Current Text-to-Image (T2I) models can create nice-looking images but often struggle with placing multiple objects correctly and ensuring each object looks realistic. Previous methods like Layout-to-Image (L2I) tried to help with positioning but didn't fully solve the problem of accurately representing the features of each object.
What's the solution?
The authors propose a new task called Instance Feature Generation (IFG) and introduce the Instance Feature Adapter (IFAdapter). This tool uses special tokens and a semantic map to better control how features of different objects are generated and positioned in the image. It can be easily added to existing models without needing to retrain them. They also created a benchmark to evaluate how well different models perform in generating accurate images.
Why it matters?
This research is important because it enhances the ability of AI to generate images that closely match specific descriptions, which is useful in fields like product design, advertising, and entertainment. By improving how AI handles multiple objects in images, it opens up new possibilities for creative applications.
Abstract
While Text-to-Image (T2I) diffusion models excel at generating visually appealing images of individual instances, they struggle to accurately position and control the features generation of multiple instances. The Layout-to-Image (L2I) task was introduced to address the positioning challenges by incorporating bounding boxes as spatial control signals, but it still falls short in generating precise instance features. In response, we propose the Instance Feature Generation (IFG) task, which aims to ensure both positional accuracy and feature fidelity in generated instances. To address the IFG task, we introduce the Instance Feature Adapter (IFAdapter). The IFAdapter enhances feature depiction by incorporating additional appearance tokens and utilizing an Instance Semantic Map to align instance-level features with spatial locations. The IFAdapter guides the diffusion process as a plug-and-play module, making it adaptable to various community models. For evaluation, we contribute an IFG benchmark and develop a verification pipeline to objectively compare models' abilities to generate instances with accurate positioning and features. Experimental results demonstrate that IFAdapter outperforms other models in both quantitative and qualitative evaluations.