Image Conductor: Precision Control for Interactive Video Synthesis
Yaowei Li, Xintao Wang, Zhaoyang Zhang, Zhouxia Wang, Ziyang Yuan, Liangbin Xie, Yuexian Zou, Ying Shan
2024-06-26
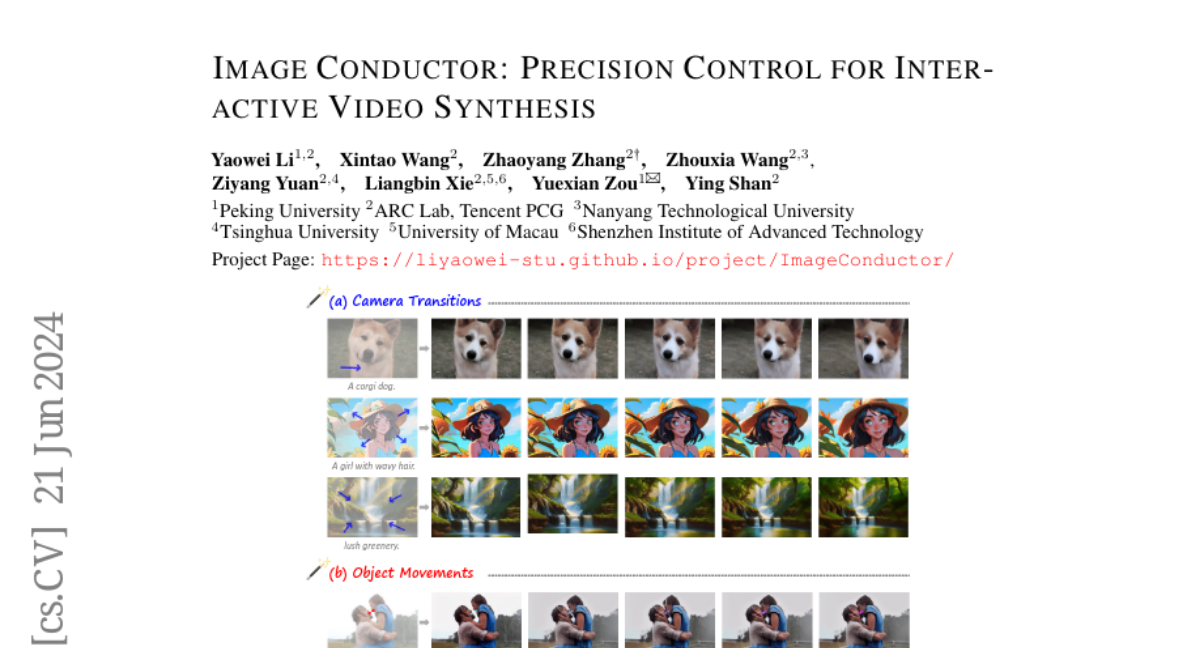
Summary
This paper introduces Image Conductor, a new method that allows filmmakers and animators to have precise control over how cameras move and how objects animate in videos, all starting from just a single image.
What's the problem?
Creating videos often requires complex techniques to manage how the camera moves and how objects are animated. Traditional methods can be very labor-intensive and time-consuming because they rely on capturing real-world footage. Even with advancements in AI, it has been difficult to achieve the level of control needed for interactive video production, making it hard to create high-quality animations efficiently.
What's the solution?
Image Conductor addresses these challenges by providing a way to control camera transitions and object movements precisely without needing extensive real-world capturing. It uses a special training strategy that separates the movements of the camera from the movements of objects in the scene. The method also includes a camera-free guidance technique that improves how objects move while removing the need for complex camera transitions. Additionally, it develops a system for curating video motion data to train the model effectively.
Why it matters?
This research is important because it enhances the capabilities of video synthesis, allowing creators to produce interactive and dynamic video content more easily and efficiently. By improving control over animations and camera movements, Image Conductor can significantly benefit fields like filmmaking, animation, and game design, making it easier for creators to bring their ideas to life.
Abstract
Filmmaking and animation production often require sophisticated techniques for coordinating camera transitions and object movements, typically involving labor-intensive real-world capturing. Despite advancements in generative AI for video creation, achieving precise control over motion for interactive video asset generation remains challenging. To this end, we propose Image Conductor, a method for precise control of camera transitions and object movements to generate video assets from a single image. An well-cultivated training strategy is proposed to separate distinct camera and object motion by camera LoRA weights and object LoRA weights. To further address cinematographic variations from ill-posed trajectories, we introduce a camera-free guidance technique during inference, enhancing object movements while eliminating camera transitions. Additionally, we develop a trajectory-oriented video motion data curation pipeline for training. Quantitative and qualitative experiments demonstrate our method's precision and fine-grained control in generating motion-controllable videos from images, advancing the practical application of interactive video synthesis. Project webpage available at https://liyaowei-stu.github.io/project/ImageConductor/