Imagine yourself: Tuning-Free Personalized Image Generation
Zecheng He, Bo Sun, Felix Juefei-Xu, Haoyu Ma, Ankit Ramchandani, Vincent Cheung, Siddharth Shah, Anmol Kalia, Harihar Subramanyam, Alireza Zareian, Li Chen, Ankit Jain, Ning Zhang, Peizhao Zhang, Roshan Sumbaly, Peter Vajda, Animesh Sinha
2024-09-23
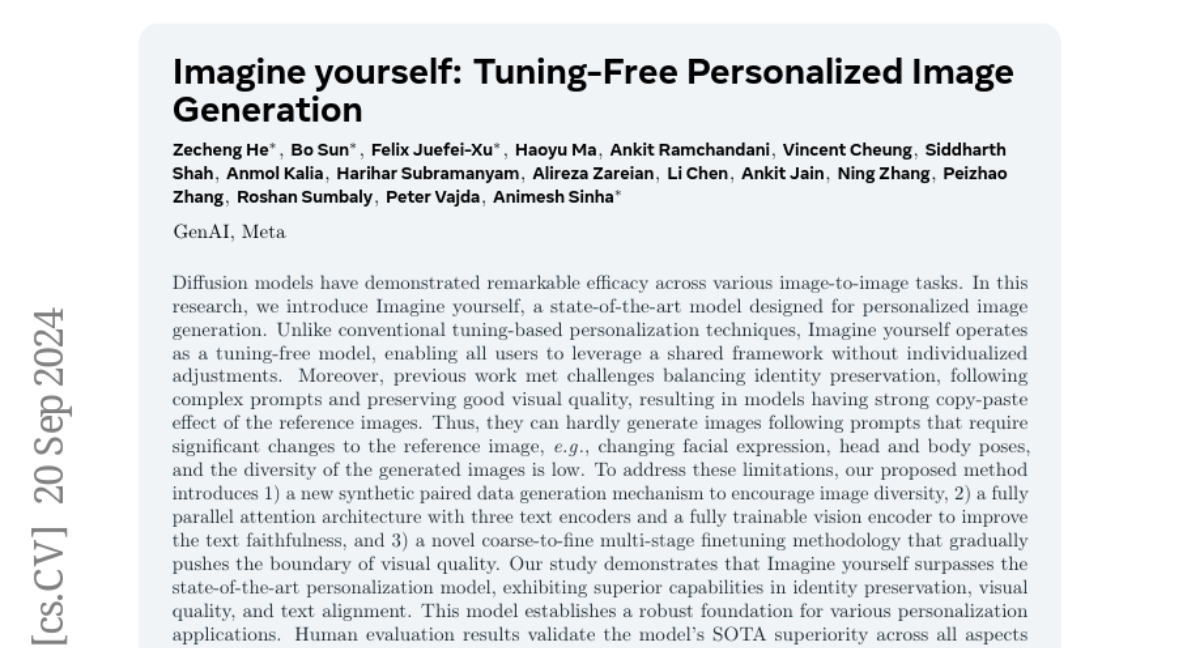
Summary
This paper introduces a new model called 'Imagine Yourself' that allows people to create personalized images without needing to adjust or fine-tune the underlying AI model. This makes it easier for users to generate images that reflect their individual styles and preferences.
What's the problem?
Traditionally, creating personalized images requires a lot of customization and tuning of the AI model, which can be complicated and time-consuming. Previous methods often struggled with maintaining the person's identity in the images while also following complex prompts and producing high-quality visuals. This led to issues like the model simply copying parts of reference images instead of generating unique ones.
What's the solution?
The researchers developed 'Imagine Yourself' as a tuning-free model that simplifies this process. They introduced several innovations: a new way to generate diverse image pairs, a parallel attention system that uses multiple text encoders to improve how well the generated images match the input prompts, and a multi-stage approach that gradually enhances image quality. These changes help the model create images that are more varied, visually appealing, and true to the user's identity without extensive adjustments.
Why it matters?
This research is significant because it opens up new possibilities for personalized image generation, making it accessible to more people. By eliminating the need for complex tuning, users can easily create images that express their unique styles and preferences, which can enhance creative projects in areas like art, marketing, and social media.
Abstract
Diffusion models have demonstrated remarkable efficacy across various image-to-image tasks. In this research, we introduce Imagine yourself, a state-of-the-art model designed for personalized image generation. Unlike conventional tuning-based personalization techniques, Imagine yourself operates as a tuning-free model, enabling all users to leverage a shared framework without individualized adjustments. Moreover, previous work met challenges balancing identity preservation, following complex prompts and preserving good visual quality, resulting in models having strong copy-paste effect of the reference images. Thus, they can hardly generate images following prompts that require significant changes to the reference image, \eg, changing facial expression, head and body poses, and the diversity of the generated images is low. To address these limitations, our proposed method introduces 1) a new synthetic paired data generation mechanism to encourage image diversity, 2) a fully parallel attention architecture with three text encoders and a fully trainable vision encoder to improve the text faithfulness, and 3) a novel coarse-to-fine multi-stage finetuning methodology that gradually pushes the boundary of visual quality. Our study demonstrates that Imagine yourself surpasses the state-of-the-art personalization model, exhibiting superior capabilities in identity preservation, visual quality, and text alignment. This model establishes a robust foundation for various personalization applications. Human evaluation results validate the model's SOTA superiority across all aspects (identity preservation, text faithfulness, and visual appeal) compared to the previous personalization models.