Immiscible Diffusion: Accelerating Diffusion Training with Noise Assignment
Yiheng Li, Heyang Jiang, Akio Kodaira, Masayoshi Tomizuka, Kurt Keutzer, Chenfeng Xu
2024-06-19
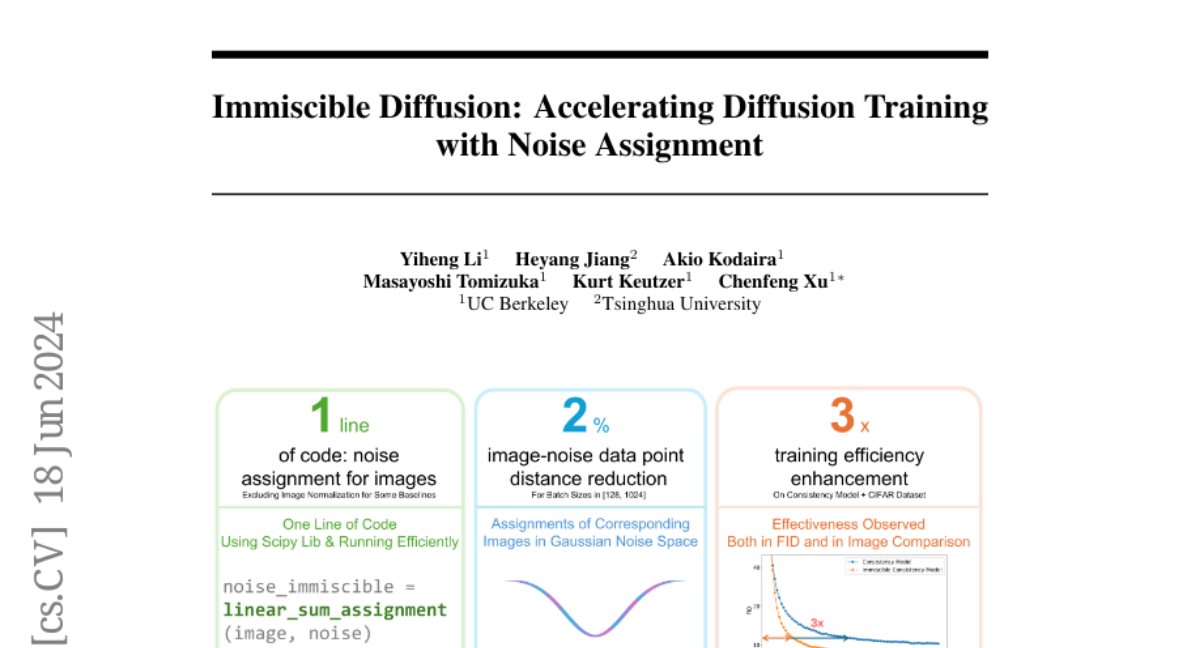
Summary
This paper introduces a new method called Immiscible Diffusion, which aims to speed up the training of diffusion models used in image processing. The authors point out that current methods for mixing noise with images are inefficient and propose a better way to handle this process.
What's the problem?
In the training of diffusion models, images are mixed with noise in a way that can slow down the learning process. This happens because current methods treat all images and noise equally, leading to confusion during training. When every image is mixed with every type of noise, it complicates how well the model can learn to remove noise from images, resulting in slower training times and less effective models.
What's the solution?
To solve this problem, the authors developed Immiscible Diffusion, which uses a strategy inspired by physics. Instead of mixing all images with all noises, this method first assigns specific types of noise to each image based on their similarities. By minimizing the distance between each image and its assigned noise, the model can focus on more relevant information. This approach only requires one line of code to implement and still keeps the noise distribution intact. Additionally, they introduced a quantized-assignment method to make the process faster and less complex. Experiments showed that this new method can train models up to three times faster on certain datasets.
Why it matters?
This research is important because it provides a more efficient way to train diffusion models, which are widely used in image generation and editing tasks. By improving training speed and effectiveness, Immiscible Diffusion could lead to better quality images being produced in less time. This advancement has practical implications for industries like gaming, film, and any field that relies on high-quality visual content.
Abstract
In this paper, we point out suboptimal noise-data mapping leads to slow training of diffusion models. During diffusion training, current methods diffuse each image across the entire noise space, resulting in a mixture of all images at every point in the noise layer. We emphasize that this random mixture of noise-data mapping complicates the optimization of the denoising function in diffusion models. Drawing inspiration from the immiscible phenomenon in physics, we propose Immiscible Diffusion, a simple and effective method to improve the random mixture of noise-data mapping. In physics, miscibility can vary according to various intermolecular forces. Thus, immiscibility means that the mixing of the molecular sources is distinguishable. Inspired by this, we propose an assignment-then-diffusion training strategy. Specifically, prior to diffusing the image data into noise, we assign diffusion target noise for the image data by minimizing the total image-noise pair distance in a mini-batch. The assignment functions analogously to external forces to separate the diffuse-able areas of images, thus mitigating the inherent difficulties in diffusion training. Our approach is remarkably simple, requiring only one line of code to restrict the diffuse-able area for each image while preserving the Gaussian distribution of noise. This ensures that each image is projected only to nearby noise. To address the high complexity of the assignment algorithm, we employ a quantized-assignment method to reduce the computational overhead to a negligible level. Experiments demonstrate that our method achieve up to 3x faster training for consistency models and DDIM on the CIFAR dataset, and up to 1.3x faster on CelebA datasets for consistency models. Besides, we conduct thorough analysis about the Immiscible Diffusion, which sheds lights on how it improves diffusion training speed while improving the fidelity.