Improving Autonomous AI Agents with Reflective Tree Search and Self-Learning
Xiao Yu, Baolin Peng, Vineeth Vajipey, Hao Cheng, Michel Galley, Jianfeng Gao, Zhou Yu
2024-10-04
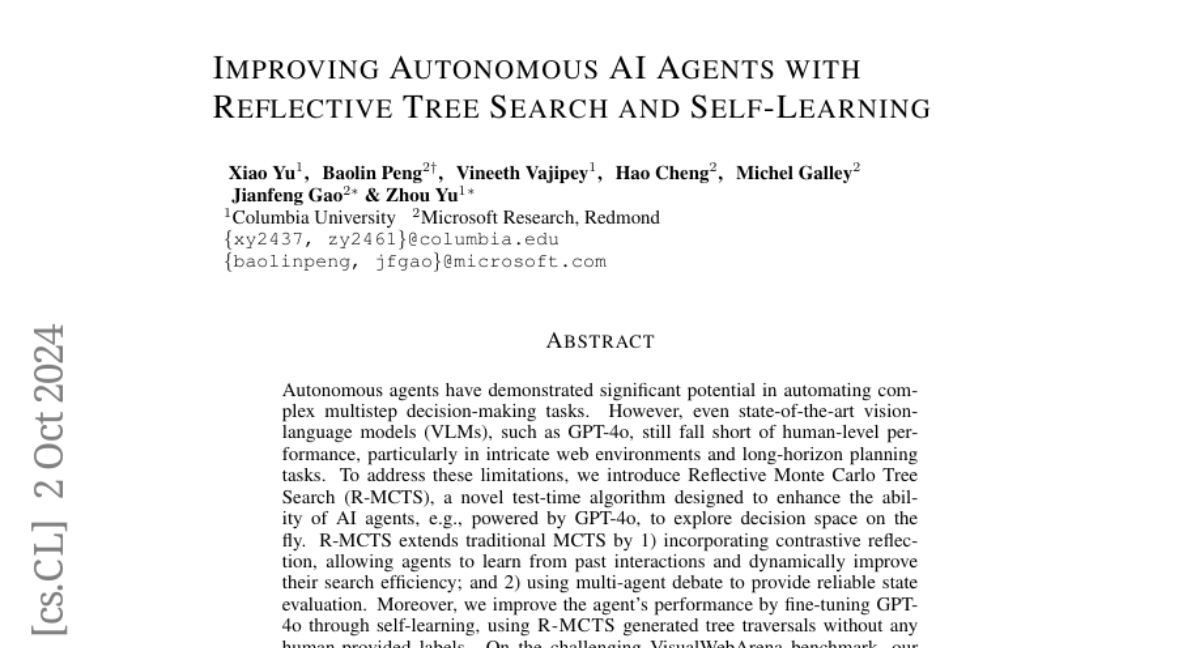
Summary
This paper discusses a new method called Reflective Monte Carlo Tree Search (R-MCTS) that improves how autonomous AI agents make decisions by allowing them to learn from past experiences in real-time.
What's the problem?
Autonomous AI agents, like those powered by advanced language models, often struggle with complex decision-making tasks that require multiple steps. Despite improvements in AI technology, these agents still don't perform as well as humans, especially in complicated environments like the web. They have difficulty adapting to new situations and can make mistakes without learning from them.
What's the solution?
To tackle this challenge, the authors introduce R-MCTS, which enhances traditional decision-making algorithms by incorporating two key features: contrastive reflection and multi-agent debate. Contrastive reflection allows the AI to learn from its past actions and improve its decision-making process on the fly. Multi-agent debate helps the AI evaluate different choices more reliably by simulating discussions among multiple agents. Additionally, the authors fine-tune the language model using self-learning techniques based on the insights gained during these decision-making processes, which helps the model perform better over time.
Why it matters?
This research is important because it significantly enhances the capabilities of autonomous AI agents, making them more effective at navigating complex tasks. By improving how these agents learn and adapt in real-time, R-MCTS can lead to better performance in applications like web navigation, automated customer service, and other areas where intelligent decision-making is crucial.
Abstract
Autonomous agents have demonstrated significant potential in automating complex multistep decision-making tasks. However, even state-of-the-art vision-language models (VLMs), such as GPT-4o, still fall short of human-level performance, particularly in intricate web environments and long-horizon planning tasks. To address these limitations, we introduce Reflective Monte Carlo Tree Search (R-MCTS), a novel test-time algorithm designed to enhance the ability of AI agents, e.g., powered by GPT-4o, to explore decision space on the fly. R-MCTS extends traditional MCTS by 1) incorporating contrastive reflection, allowing agents to learn from past interactions and dynamically improve their search efficiency; and 2) using multi-agent debate to provide reliable state evaluation. Moreover, we improve the agent's performance by fine-tuning GPT-4o through self-learning, using R-MCTS generated tree traversals without any human-provided labels. On the challenging VisualWebArena benchmark, our GPT-4o-based R-MCTS agent achieves a 6% to 30% relative improvement across various tasks compared to the previous state-of-the-art. Additionally, we show that the knowledge gained from test-time search can be effectively transferred back to GPT-4o via fine-tuning. The fine-tuned GPT-4o matches 97% of R-MCTS's performance while reducing compute usage by a factor of four at test time. Furthermore, qualitative results reveal that the fine-tuned GPT-4o model demonstrates the ability to explore the environment, evaluate a state, and backtrack to viable ones when it detects that the current state cannot lead to success. Moreover, our work demonstrates the compute scaling properties in both training - data collection with R-MCTS - and testing time. These results suggest a promising research direction to enhance VLMs' reasoning and planning capabilities for agentic applications via test-time search and self-learning.