In Case You Missed It: ARC 'Challenge' Is Not That Challenging
Łukasz Borchmann
2024-12-25
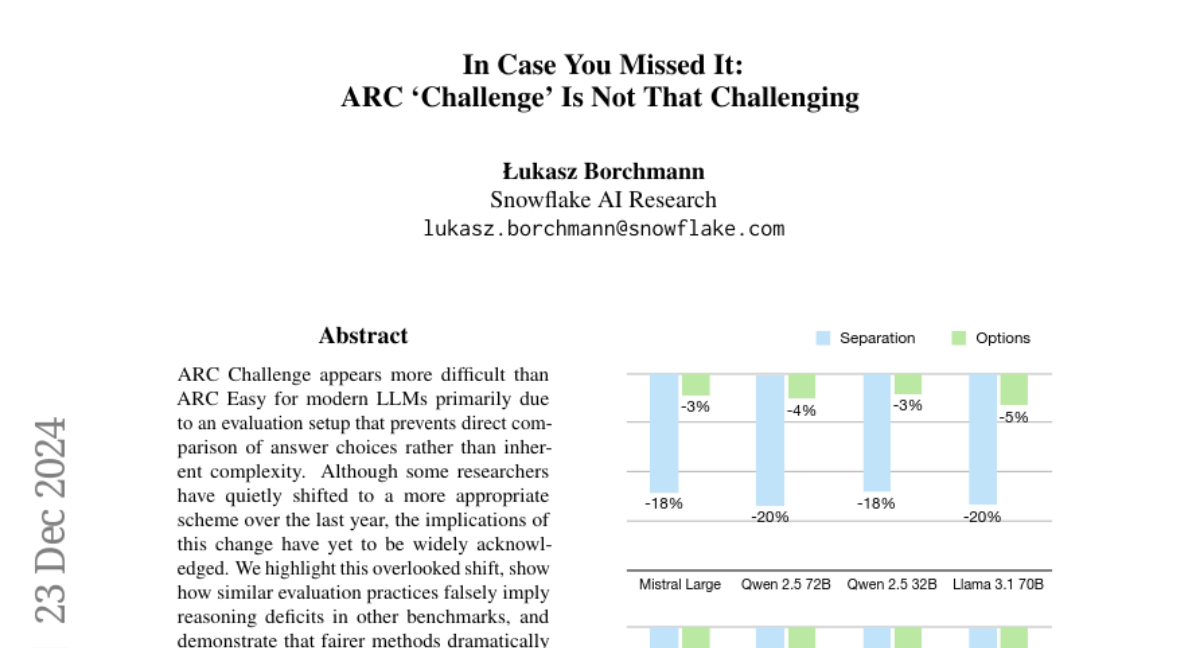
Summary
This paper talks about how the ARC Challenge, a test designed to measure AI reasoning abilities, may not be as difficult as it seems for modern large language models (LLMs) due to the way the evaluation is set up.
What's the problem?
The ARC Challenge appears harder than the ARC Easy version mainly because of how the answers are evaluated. This setup makes it difficult to compare different answer choices directly, which can create a false impression that LLMs are not performing well on complex reasoning tasks. Some researchers have started using better evaluation methods, but this change hasn't been widely recognized yet.
What's the solution?
The authors highlight this shift in evaluation practices and show that using fairer methods can significantly reduce the performance gaps seen in other benchmarks. They demonstrate that with these improved evaluation techniques, LLMs can achieve much better results, even surpassing human-level performance on certain tasks. They also provide guidelines to ensure that multiple-choice evaluations accurately reflect what models can actually do.
Why it matters?
This research is important because it reveals how the way we test AI systems can affect our understanding of their abilities. By improving evaluation methods, we can get a clearer picture of how well these models perform and ensure that they are being assessed fairly. This could lead to better AI development and more accurate interpretations of their capabilities.
Abstract
ARC Challenge appears more difficult than ARC Easy for modern LLMs primarily due to an evaluation setup that prevents direct comparison of answer choices rather than inherent complexity. Although some researchers have quietly shifted to a more appropriate scheme over the last year, the implications of this change have yet to be widely acknowledged. We highlight this overlooked shift, show how similar evaluation practices falsely imply reasoning deficits in other benchmarks, and demonstrate that fairer methods dramatically reduce performance gaps (e.g. on SIQA) and even yield superhuman results (OpenBookQA). In doing so, we reveal how evaluation shapes perceived difficulty and offer guidelines to ensure that multiple-choice evaluations accurately reflect actual model capabilities.