Inference Scaling for Long-Context Retrieval Augmented Generation
Zhenrui Yue, Honglei Zhuang, Aijun Bai, Kai Hui, Rolf Jagerman, Hansi Zeng, Zhen Qin, Dong Wang, Xuanhui Wang, Michael Bendersky
2024-10-09
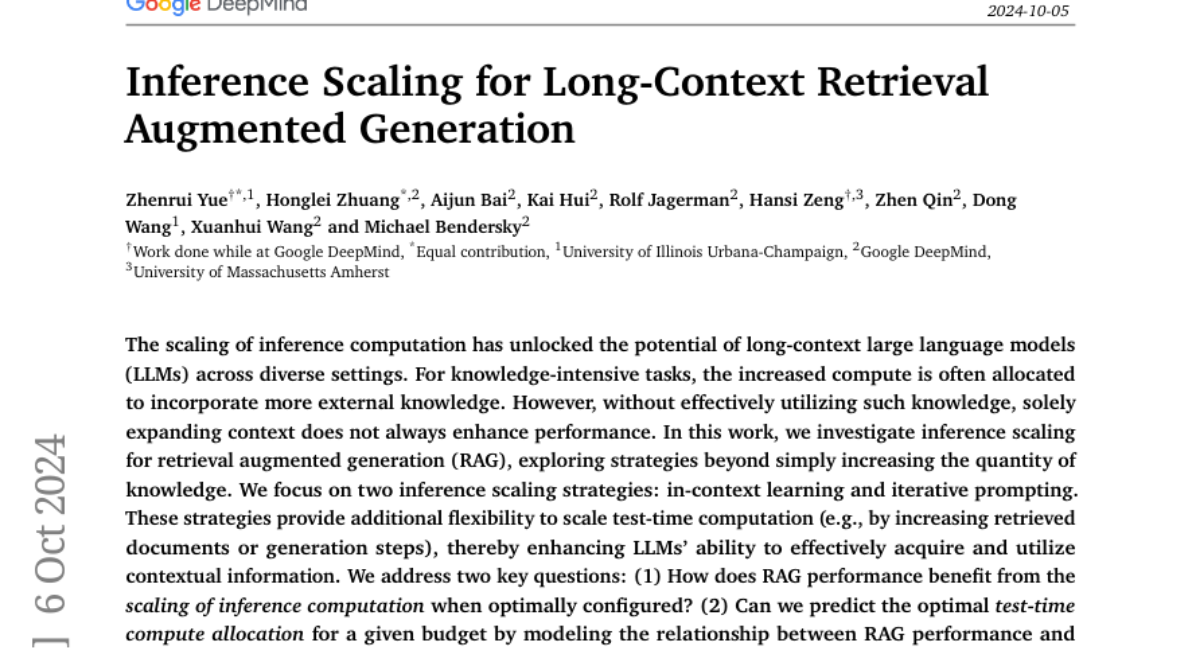
Summary
This paper discusses a method called Inference Scaling for improving how large language models (LLMs) generate text by effectively using external knowledge, especially when dealing with long pieces of information.
What's the problem?
While LLMs can handle long contexts, simply adding more information doesn't always lead to better performance. If the models don't use this knowledge effectively, they may not improve at all. This creates a challenge in optimizing how LLMs retrieve and generate information from large amounts of data.
What's the solution?
The authors explore two strategies for scaling inference: in-context learning and iterative prompting. These methods allow LLMs to dynamically adjust how they access and use information during the generation process. They also developed a model to predict the best way to allocate computational resources to maximize performance based on different tasks and constraints. Their experiments showed that when inference is optimally configured, LLMs can achieve significant performance improvements, up to 58.9% better than standard methods.
Why it matters?
This research is important because it provides insights into how to make LLMs more efficient and effective at generating high-quality text from long contexts. By optimizing how these models use external knowledge, it can lead to better applications in areas like content creation, search engines, and any task that requires understanding complex information.
Abstract
The scaling of inference computation has unlocked the potential of long-context large language models (LLMs) across diverse settings. For knowledge-intensive tasks, the increased compute is often allocated to incorporate more external knowledge. However, without effectively utilizing such knowledge, solely expanding context does not always enhance performance. In this work, we investigate inference scaling for retrieval augmented generation (RAG), exploring strategies beyond simply increasing the quantity of knowledge. We focus on two inference scaling strategies: in-context learning and iterative prompting. These strategies provide additional flexibility to scale test-time computation (e.g., by increasing retrieved documents or generation steps), thereby enhancing LLMs' ability to effectively acquire and utilize contextual information. We address two key questions: (1) How does RAG performance benefit from the scaling of inference computation when optimally configured? (2) Can we predict the optimal test-time compute allocation for a given budget by modeling the relationship between RAG performance and inference parameters? Our observations reveal that increasing inference computation leads to nearly linear gains in RAG performance when optimally allocated, a relationship we describe as the inference scaling laws for RAG. Building on this, we further develop the computation allocation model to estimate RAG performance across different inference configurations. The model predicts optimal inference parameters under various computation constraints, which align closely with the experimental results. By applying these optimal configurations, we demonstrate that scaling inference compute on long-context LLMs achieves up to 58.9% gains on benchmark datasets compared to standard RAG.