InfiMM-WebMath-40B: Advancing Multimodal Pre-Training for Enhanced Mathematical Reasoning
Xiaotian Han, Yiren Jian, Xuefeng Hu, Haogeng Liu, Yiqi Wang, Qihang Fan, Yuang Ai, Huaibo Huang, Ran He, Zhenheng Yang, Quanzeng You
2024-09-20
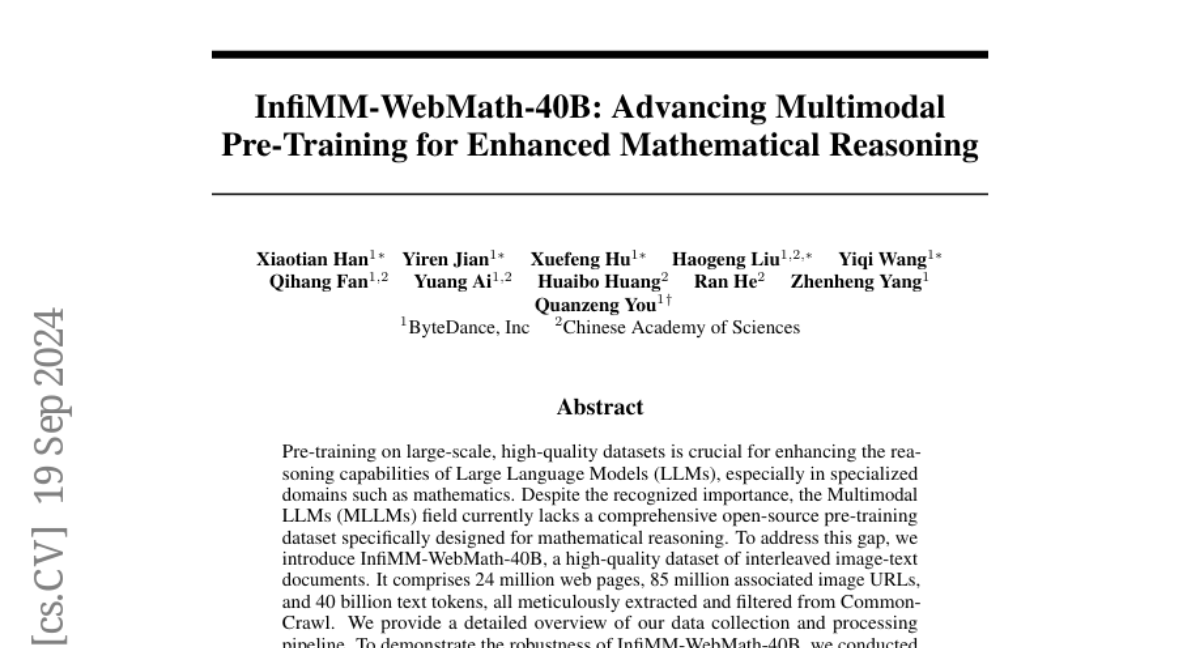
Summary
This paper introduces InfiMM-WebMath-40B, a new dataset designed to improve the ability of large language models (LLMs) to understand and solve mathematical problems by combining text and images.
What's the problem?
While large language models have made significant progress in understanding language, they often struggle with specialized tasks like mathematical reasoning. There hasn't been a comprehensive dataset specifically designed for training these models in multimodal math contexts, which limits their effectiveness in this area.
What's the solution?
To address this issue, the authors created InfiMM-WebMath-40B, which includes 24 million web pages, 85 million images, and 40 billion text tokens sourced from the internet. This dataset combines both text and images related to mathematics and science, providing a rich resource for training LLMs. The authors detail their data collection methods and demonstrate that models trained on this dataset perform exceptionally well on various math benchmarks, even outperforming existing models that use much larger datasets.
Why it matters?
This research is important because it fills a significant gap in the resources available for training AI models in mathematical reasoning. By providing a high-quality multimodal dataset, InfiMM-WebMath-40B enables better training of LLMs, which can lead to improved performance in applications requiring complex problem-solving skills, such as tutoring systems and educational tools.
Abstract
Pre-training on large-scale, high-quality datasets is crucial for enhancing the reasoning capabilities of Large Language Models (LLMs), especially in specialized domains such as mathematics. Despite the recognized importance, the Multimodal LLMs (MLLMs) field currently lacks a comprehensive open-source pre-training dataset specifically designed for mathematical reasoning. To address this gap, we introduce InfiMM-WebMath-40B, a high-quality dataset of interleaved image-text documents. It comprises 24 million web pages, 85 million associated image URLs, and 40 billion text tokens, all meticulously extracted and filtered from CommonCrawl. We provide a detailed overview of our data collection and processing pipeline. To demonstrate the robustness of InfiMM-WebMath-40B, we conducted evaluations in both text-only and multimodal settings. Our evaluations on text-only benchmarks show that, despite utilizing only 40 billion tokens, our dataset significantly enhances the performance of our 1.3B model, delivering results comparable to DeepSeekMath-1.3B, which uses 120 billion tokens for the same model size. Nevertheless, with the introduction of our multi-modal math pre-training dataset, our models set a new state-of-the-art among open-source models on multi-modal math benchmarks such as MathVerse and We-Math. We release our data at https://huggingface.co/datasets/Infi-MM/InfiMM-WebMath-40B.