InstantDrag: Improving Interactivity in Drag-based Image Editing
Joonghyuk Shin, Daehyeon Choi, Jaesik Park
2024-09-16
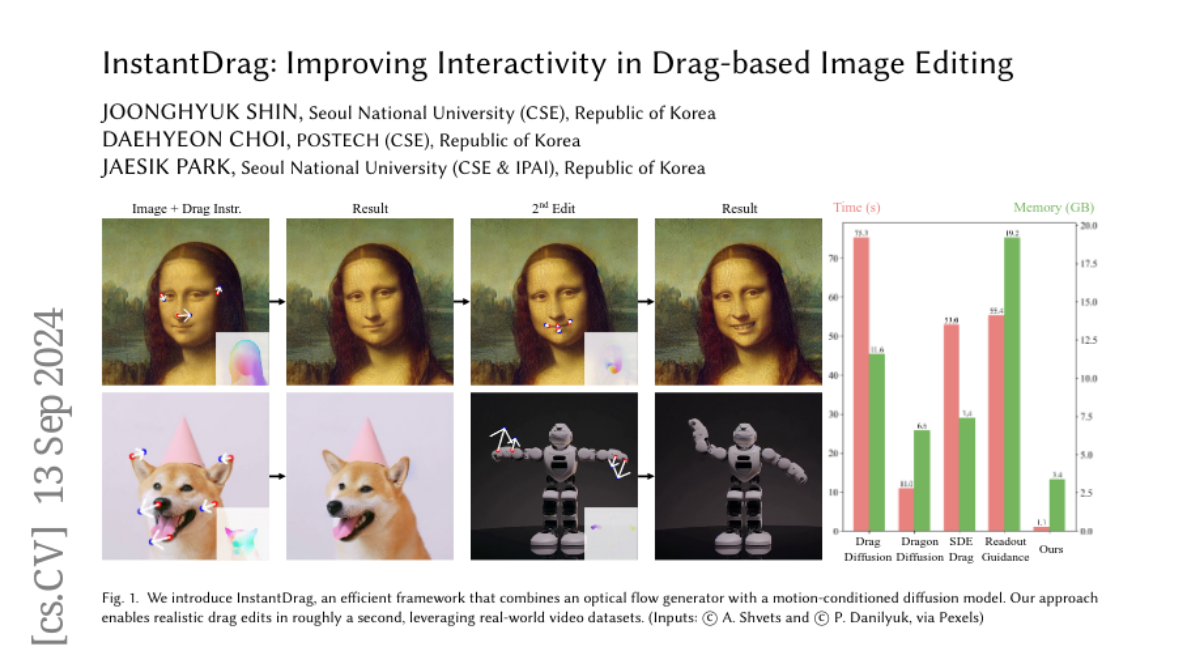
Summary
This paper introduces InstantDrag, a new method that improves how users can interactively edit images by dragging parts of the image around, making the editing process faster and easier.
What's the problem?
Drag-based image editing is popular because it allows for precise control, but existing methods are slow and require complicated setups, like using masks or text prompts. This makes the editing process less interactive and can frustrate users who want quick results.
What's the solution?
InstantDrag simplifies the process by using two specialized networks: FlowGen and FlowDiffusion. FlowGen generates detailed motion data based on simple drag instructions, while FlowDiffusion uses this data to make realistic edits to the image. This method does not require extra inputs like masks or text prompts, allowing for fast edits—typically completed in about a second.
Why it matters?
This research is important because it enhances user experience in image editing software. By making drag-based editing quicker and more intuitive, it opens up new possibilities for artists and designers to create and modify images in real-time without the usual complexities.
Abstract
Drag-based image editing has recently gained popularity for its interactivity and precision. However, despite the ability of text-to-image models to generate samples within a second, drag editing still lags behind due to the challenge of accurately reflecting user interaction while maintaining image content. Some existing approaches rely on computationally intensive per-image optimization or intricate guidance-based methods, requiring additional inputs such as masks for movable regions and text prompts, thereby compromising the interactivity of the editing process. We introduce InstantDrag, an optimization-free pipeline that enhances interactivity and speed, requiring only an image and a drag instruction as input. InstantDrag consists of two carefully designed networks: a drag-conditioned optical flow generator (FlowGen) and an optical flow-conditioned diffusion model (FlowDiffusion). InstantDrag learns motion dynamics for drag-based image editing in real-world video datasets by decomposing the task into motion generation and motion-conditioned image generation. We demonstrate InstantDrag's capability to perform fast, photo-realistic edits without masks or text prompts through experiments on facial video datasets and general scenes. These results highlight the efficiency of our approach in handling drag-based image editing, making it a promising solution for interactive, real-time applications.