Integrating Large Language Models into a Tri-Modal Architecture for Automated Depression Classification
Santosh V. Patapati
2024-07-30
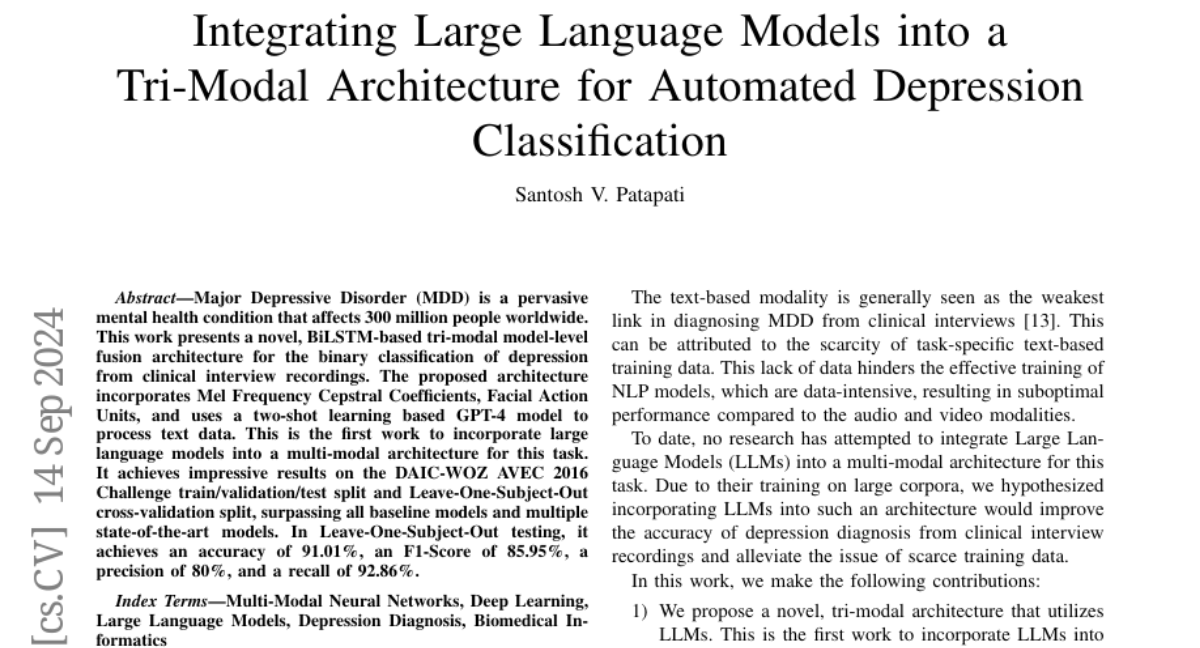
Summary
This paper presents SaulLM-54B and SaulLM-141B, two large language models specifically designed for the legal field. These models are built to better understand and process legal texts, improving how AI can assist in legal tasks.
What's the problem?
Understanding legal documents can be very challenging due to the complex language and specific terminology used in the legal field. Traditional language models often struggle with this because they are not specifically trained on legal texts, which can lead to inaccuracies and misunderstandings in legal applications.
What's the solution?
To address this problem, the authors developed two large language models, SaulLM-54B and SaulLM-141B, which contain 54 billion and 141 billion parameters, respectively. They used a method called domain adaptation, which involves three main strategies: continuing to train the models with over 540 billion tokens of legal text, implementing a special protocol for following legal instructions, and aligning the model's outputs with human preferences in interpreting legal information. This approach helps the models generate more accurate and relevant responses when dealing with legal texts.
Why it matters?
This research is significant because it enhances the ability of AI systems to work effectively in the legal domain, making them more useful for tasks like legal research, contract analysis, and case summarization. By improving how machines understand legal language, these models can help lawyers and other professionals save time and reduce errors in their work, ultimately making legal services more accessible.
Abstract
Major Depressive Disorder (MDD) is a pervasive mental health condition that affects 300 million people worldwide. This work presents a novel, BiLSTM-based tri-modal model-level fusion architecture for the binary classification of depression from clinical interview recordings. The proposed architecture incorporates Mel Frequency Cepstral Coefficients, Facial Action Units, and uses a two-shot learning based GPT-4 model to process text data. This is the first work to incorporate large language models into a multi-modal architecture for this task. It achieves impressive results on the DAIC-WOZ AVEC 2016 Challenge cross-validation split and Leave-One-Subject-Out cross-validation split, surpassing all baseline models and multiple state-of-the-art models. In Leave-One-Subject-Out testing, it achieves an accuracy of 91.01%, an F1-Score of 85.95%, a precision of 80%, and a recall of 92.86%.