InternLM-XComposer-2.5: A Versatile Large Vision Language Model Supporting Long-Contextual Input and Output
Pan Zhang, Xiaoyi Dong, Yuhang Zang, Yuhang Cao, Rui Qian, Lin Chen, Qipeng Guo, Haodong Duan, Bin Wang, Linke Ouyang, Songyang Zhang, Wenwei Zhang, Yining Li, Yang Gao, Peng Sun, Xinyue Zhang, Wei Li, Jingwen Li, Wenhai Wang, Hang Yan, Conghui He, Xingcheng Zhang
2024-07-04
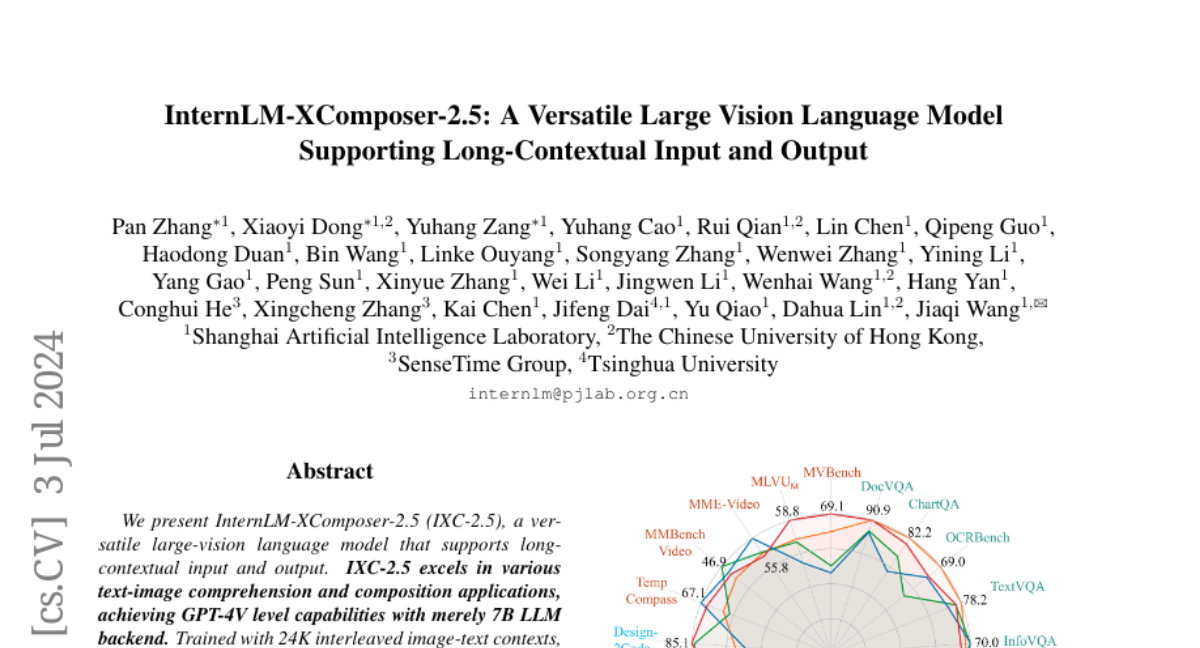
Summary
This paper talks about InternLM-XComposer-2.5 (IXC-2.5), a new advanced model that combines text and images, allowing it to handle long inputs and outputs effectively, making it great for various tasks like understanding videos and creating web content.
What's the problem?
The main problem is that many existing models struggle with long inputs and outputs, which limits their ability to understand complex information or generate detailed responses. Additionally, they often lack the ability to work well with both text and images together.
What's the solution?
To address these issues, the authors developed IXC-2.5, which can process up to 96,000 tokens of information at once, thanks to a special technique called RoPE extrapolation. This model has three major upgrades: it can understand ultra-high-resolution images, analyze videos in detail, and engage in multi-turn conversations involving multiple images. It also includes new features that allow it to create webpages and high-quality articles by combining text and images effectively.
Why it matters?
This research is important because it pushes the boundaries of what AI can do with visual and textual information. By improving how models handle long contexts and complex tasks, IXC-2.5 can enhance applications in education, content creation, and many other fields where understanding both text and images is crucial.
Abstract
We present InternLM-XComposer-2.5 (IXC-2.5), a versatile large-vision language model that supports long-contextual input and output. IXC-2.5 excels in various text-image comprehension and composition applications, achieving GPT-4V level capabilities with merely 7B LLM backend. Trained with 24K interleaved image-text contexts, it can seamlessly extend to 96K long contexts via RoPE extrapolation. This long-context capability allows IXC-2.5 to excel in tasks requiring extensive input and output contexts. Compared to its previous 2.0 version, InternLM-XComposer-2.5 features three major upgrades in vision-language comprehension: (1) Ultra-High Resolution Understanding, (2) Fine-Grained Video Understanding, and (3) Multi-Turn Multi-Image Dialogue. In addition to comprehension, IXC-2.5 extends to two compelling applications using extra LoRA parameters for text-image composition: (1) Crafting Webpages and (2) Composing High-Quality Text-Image Articles. IXC-2.5 has been evaluated on 28 benchmarks, outperforming existing open-source state-of-the-art models on 16 benchmarks. It also surpasses or competes closely with GPT-4V and Gemini Pro on 16 key tasks. The InternLM-XComposer-2.5 is publicly available at https://github.com/InternLM/InternLM-XComposer.