Interpreting and Editing Vision-Language Representations to Mitigate Hallucinations
Nick Jiang, Anish Kachinthaya, Suzie Petryk, Yossi Gandelsman
2024-10-04
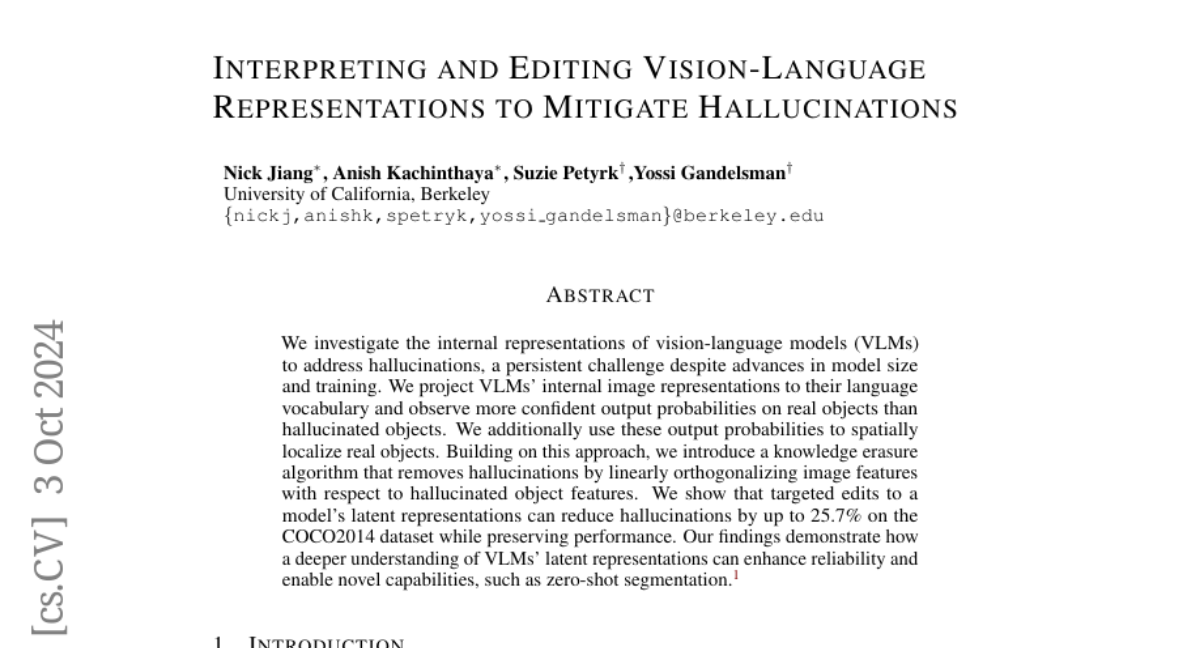
Summary
This paper explores ways to understand and improve vision-language models (VLMs) to reduce hallucinations, which are incorrect or made-up information that these models sometimes generate.
What's the problem?
Vision-language models are designed to understand and generate information based on both images and text. However, they often produce hallucinations, meaning they create details or objects that aren't actually present in the images. This issue is significant because it can lead to misleading or inaccurate results, making it hard for users to trust the model's outputs.
What's the solution?
To tackle this problem, the authors investigate how VLMs process visual information and how this relates to their tendency to hallucinate. They introduce a method that involves analyzing the internal representations of these models. By using a knowledge erasure algorithm, they can adjust the model's understanding to reduce the impact of hallucinated features. Their experiments show that this approach can decrease hallucinations by up to 25.7% while maintaining overall performance on tasks.
Why it matters?
This research is important because it helps improve the reliability of vision-language models, making them more trustworthy for real-world applications such as image captioning, visual question answering, and more. By reducing hallucinations, users can have greater confidence in the information provided by these AI systems.
Abstract
We investigate the internal representations of vision-language models (VLMs) to address hallucinations, a persistent challenge despite advances in model size and training. We project VLMs' internal image representations to their language vocabulary and observe more confident output probabilities on real objects than hallucinated objects. We additionally use these output probabilities to spatially localize real objects. Building on this approach, we introduce a knowledge erasure algorithm that removes hallucinations by linearly orthogonalizing image features with respect to hallucinated object features. We show that targeted edits to a model's latent representations can reduce hallucinations by up to 25.7% on the COCO2014 dataset while preserving performance. Our findings demonstrate how a deeper understanding of VLMs' latent representations can enhance reliability and enable novel capabilities, such as zero-shot segmentation.