ITACLIP: Boosting Training-Free Semantic Segmentation with Image, Text, and Architectural Enhancements
M. Arda Aydın, Efe Mert Çırpar, Elvin Abdinli, Gozde Unal, Yusuf H. Sahin
2024-11-20
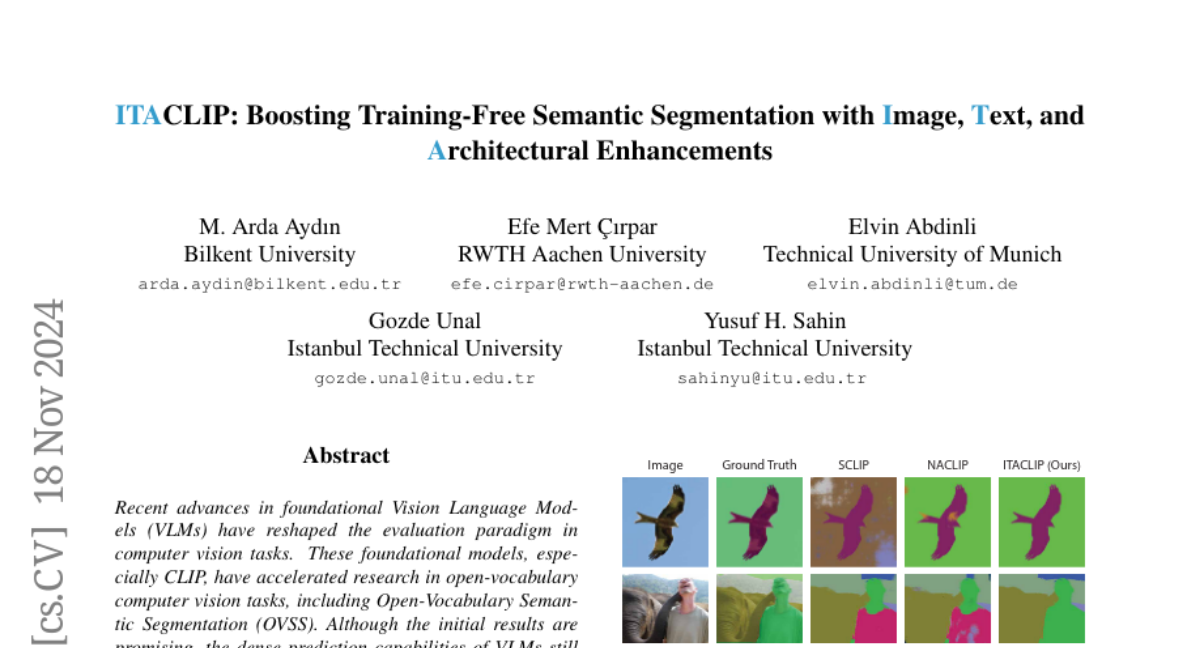
Summary
This paper introduces ITACLIP, a new method to improve semantic segmentation using the CLIP model by enhancing its architecture and incorporating text and image modifications.
What's the problem?
While large language models like CLIP have shown promise in understanding images and text together, they still struggle with tasks that require precise pixel-level understanding, such as semantic segmentation. This means that the model can identify objects in images but often lacks the accuracy needed to label each pixel correctly.
What's the solution?
To improve this, the authors propose ITACLIP, which involves three main enhancements: (1) changing the architecture of the model to better combine information from different layers, (2) applying data augmentations to improve how images are represented, and (3) using large language models to generate definitions and synonyms for class names to help the model understand them better. This approach allows ITACLIP to perform semantic segmentation without needing additional training, making it more efficient.
Why it matters?
This research is significant because it advances the capabilities of models like CLIP in performing detailed image analysis tasks. By improving semantic segmentation, ITACLIP can enhance applications in various fields such as medical imaging, autonomous vehicles, and any area where accurate image interpretation is crucial.
Abstract
Recent advances in foundational Vision Language Models (VLMs) have reshaped the evaluation paradigm in computer vision tasks. These foundational models, especially CLIP, have accelerated research in open-vocabulary computer vision tasks, including Open-Vocabulary Semantic Segmentation (OVSS). Although the initial results are promising, the dense prediction capabilities of VLMs still require further improvement. In this study, we enhance the semantic segmentation performance of CLIP by introducing new modules and modifications: 1) architectural changes in the last layer of ViT and the incorporation of attention maps from the middle layers with the last layer, 2) Image Engineering: applying data augmentations to enrich input image representations, and 3) using Large Language Models (LLMs) to generate definitions and synonyms for each class name to leverage CLIP's open-vocabulary capabilities. Our training-free method, ITACLIP, outperforms current state-of-the-art approaches on segmentation benchmarks such as COCO-Stuff, COCO-Object, Pascal Context, and Pascal VOC. Our code is available at https://github.com/m-arda-aydn/ITACLIP.