JaColBERTv2.5: Optimising Multi-Vector Retrievers to Create State-of-the-Art Japanese Retrievers with Constrained Resources
Benjamin ClaviƩ
2024-07-31
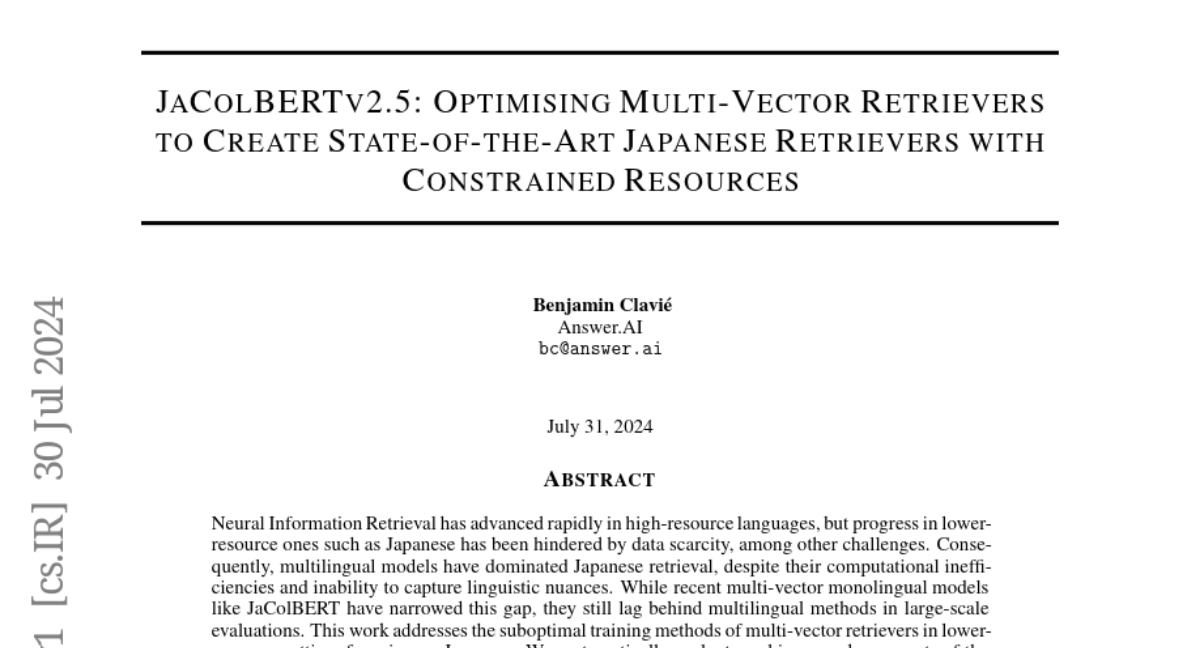
Summary
This paper introduces JaColBERTv2.5, an improved model for retrieving information in Japanese. It focuses on optimizing multi-vector retrieval methods to enhance performance while using fewer resources.
What's the problem?
While language models have made great progress in popular languages like English, they struggle with lower-resource languages such as Japanese. Existing models often rely on multilingual approaches that are not efficient and fail to capture the unique features of the Japanese language. This leads to poorer performance in tasks like document retrieval, where finding relevant information quickly is essential.
What's the solution?
To tackle these challenges, the authors developed JaColBERTv2.5 by refining the training methods for multi-vector retrieval models specifically for Japanese. They systematically evaluated and enhanced key aspects of the model's training and inference processes. A novel technique called checkpoint merging was introduced to combine the benefits of different training phases, resulting in a more effective model. JaColBERTv2.5 has only 110 million parameters and can be trained quickly, achieving better results than previous models across various benchmarks.
Why it matters?
This research is significant because it provides a specialized tool for retrieving information in Japanese, which can help improve access to knowledge and resources in this language. By enhancing the efficiency and effectiveness of document retrieval, JaColBERTv2.5 can support a wide range of applications, from academic research to everyday information searches, ultimately contributing to better communication and understanding in multilingual contexts.
Abstract
Neural Information Retrieval has advanced rapidly in high-resource languages, but progress in lower-resource ones such as Japanese has been hindered by data scarcity, among other challenges. Consequently, multilingual models have dominated Japanese retrieval, despite their computational inefficiencies and inability to capture linguistic nuances. While recent multi-vector monolingual models like JaColBERT have narrowed this gap, they still lag behind multilingual methods in large-scale evaluations. This work addresses the suboptimal training methods of multi-vector retrievers in lower-resource settings, focusing on Japanese. We systematically evaluate and improve key aspects of the inference and training settings of JaColBERT, and more broadly, multi-vector models. We further enhance performance through a novel checkpoint merging step, showcasing it to be an effective way of combining the benefits of fine-tuning with the generalization capabilities of the original checkpoint. Building on our analysis, we introduce a novel training recipe, resulting in the JaColBERTv2.5 model. JaColBERTv2.5, with only 110 million parameters and trained in under 15 hours on 4 A100 GPUs, significantly outperforms all existing methods across all common benchmarks, reaching an average score of 0.754, significantly above the previous best of 0.720. To support future research, we make our final models, intermediate checkpoints and all data used publicly available.