Jamba-1.5: Hybrid Transformer-Mamba Models at Scale
Jamba Team, Barak Lenz, Alan Arazi, Amir Bergman, Avshalom Manevich, Barak Peleg, Ben Aviram, Chen Almagor, Clara Fridman, Dan Padnos, Daniel Gissin, Daniel Jannai, Dor Muhlgay, Dor Zimberg, Edden M Gerber, Elad Dolev, Eran Krakovsky, Erez Safahi, Erez Schwartz, Gal Cohen, Gal Shachaf, Haim Rozenblum
2024-08-23
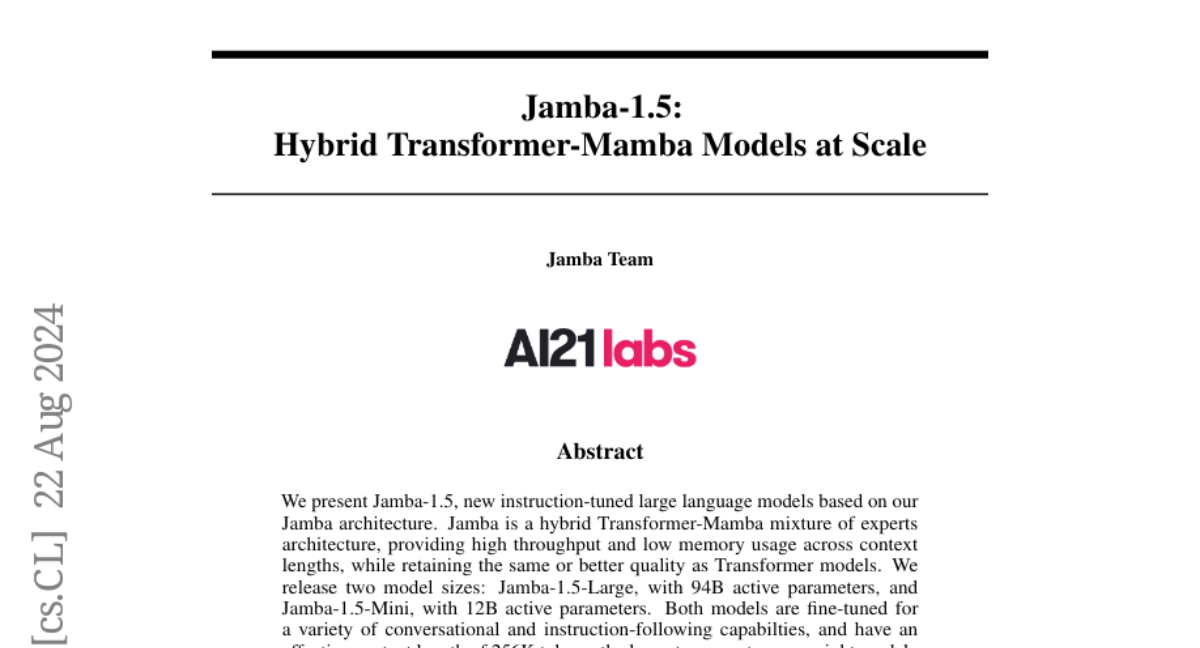
Summary
This paper introduces Jamba-1.5, a new type of large language model designed to efficiently handle long text inputs while maintaining high performance.
What's the problem?
Many existing language models struggle with processing long pieces of text because they require a lot of memory and computational power. This can lead to slower performance and higher costs when trying to generate or understand lengthy information.
What's the solution?
The authors developed Jamba-1.5, which uses a hybrid architecture called Transformer-Mamba that combines the strengths of different model types. They created two versions: Jamba-1.5-Large with 94 billion parameters and Jamba-1.5-Mini with 12 billion parameters. These models can handle up to 256,000 tokens, the longest context length available in open-weight models. They also introduced a new technique called ExpertsInt8 that allows the larger model to run efficiently on fewer GPUs without losing quality.
Why it matters?
This research is important because it enables better performance for tasks that involve long texts, such as summarizing documents or answering questions based on extensive information. By improving how language models work, it can enhance applications in various fields like education, customer service, and content creation.
Abstract
We present Jamba-1.5, new instruction-tuned large language models based on our Jamba architecture. Jamba is a hybrid Transformer-Mamba mixture of experts architecture, providing high throughput and low memory usage across context lengths, while retaining the same or better quality as Transformer models. We release two model sizes: Jamba-1.5-Large, with 94B active parameters, and Jamba-1.5-Mini, with 12B active parameters. Both models are fine-tuned for a variety of conversational and instruction-following capabilties, and have an effective context length of 256K tokens, the largest amongst open-weight models. To support cost-effective inference, we introduce ExpertsInt8, a novel quantization technique that allows fitting Jamba-1.5-Large on a machine with 8 80GB GPUs when processing 256K-token contexts without loss of quality. When evaluated on a battery of academic and chatbot benchmarks, Jamba-1.5 models achieve excellent results while providing high throughput and outperforming other open-weight models on long-context benchmarks. The model weights for both sizes are publicly available under the Jamba Open Model License and we release ExpertsInt8 as open source.