Judging the Judges: Evaluating Alignment and Vulnerabilities in LLMs-as-Judges
Aman Singh Thakur, Kartik Choudhary, Venkat Srinik Ramayapally, Sankaran Vaidyanathan, Dieuwke Hupkes
2024-06-24
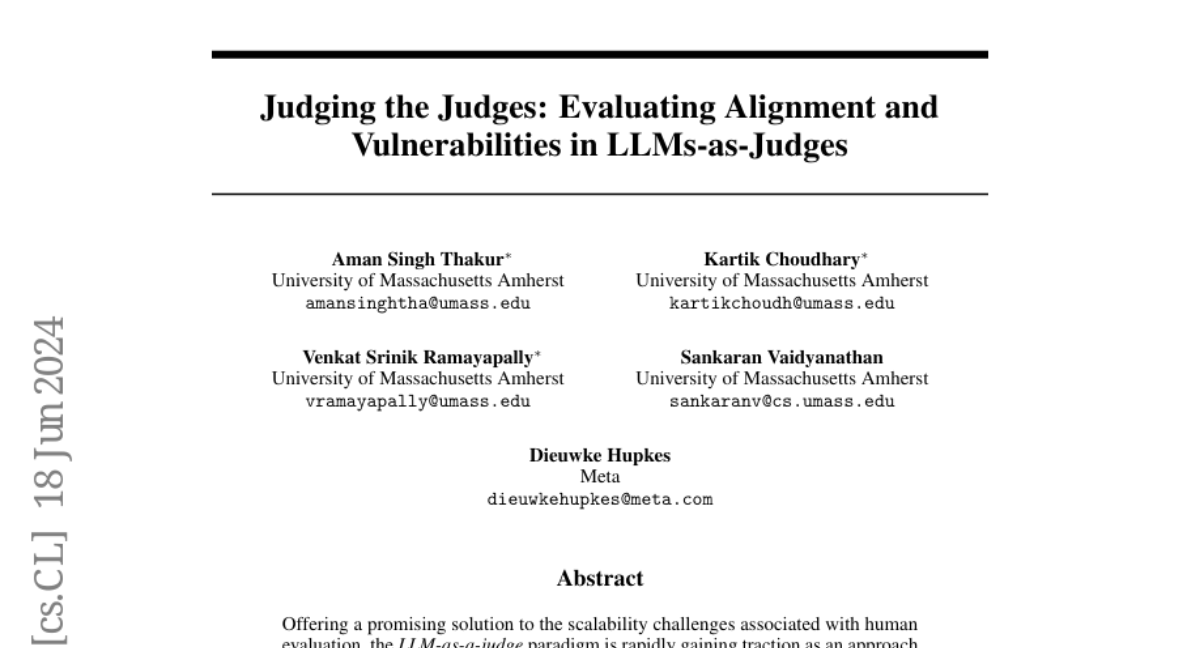
Summary
This paper explores how well large language models (LLMs) can act as judges in evaluating other models, focusing on their strengths, weaknesses, and potential biases.
What's the problem?
As AI systems become more complex, it’s important to find efficient ways to evaluate their performance. Traditionally, human evaluators assess these models, but this can be slow and not scalable. The idea of using LLMs as judges offers a promising solution. However, there are still many questions about how reliable these LLMs are when judging other models and whether they might have biases that affect their evaluations.
What's the solution?
The researchers conducted a detailed study using a benchmark called TriviaQA to test the knowledge reasoning abilities of various LLMs acting as judges. They compared the judgments of 9 different judge models against human evaluations to see how well they aligned. They discovered that while some models like Llama-3 70B and GPT-4 Turbo performed well, other models like JudgeLM-7B and a lexical judge called Contains actually did better in ranking the performance of exam-taker models. They emphasized the importance of using a statistical measure called Cohen's kappa to assess alignment instead of just looking at simple percentages, which can be misleading.
Why it matters?
This research is important because it provides insights into how we can effectively use LLMs as judges for evaluating other AI systems. By highlighting the strengths and weaknesses of these models, the study helps inform future developments in AI evaluation methods. Understanding how these models perform can lead to more accurate assessments and improvements in AI technology overall.
Abstract
Offering a promising solution to the scalability challenges associated with human evaluation, the LLM-as-a-judge paradigm is rapidly gaining traction as an approach to evaluating large language models (LLMs). However, there are still many open questions about the strengths and weaknesses of this paradigm, and what potential biases it may hold. In this paper, we present a comprehensive study of the performance of various LLMs acting as judges. We leverage TriviaQA as a benchmark for assessing objective knowledge reasoning of LLMs and evaluate them alongside human annotations which we found to have a high inter-annotator agreement. Our study includes 9 judge models and 9 exam taker models -- both base and instruction-tuned. We assess the judge model's alignment across different model sizes, families, and judge prompts. Among other results, our research rediscovers the importance of using Cohen's kappa as a metric of alignment as opposed to simple percent agreement, showing that judges with high percent agreement can still assign vastly different scores. We find that both Llama-3 70B and GPT-4 Turbo have an excellent alignment with humans, but in terms of ranking exam taker models, they are outperformed by both JudgeLM-7B and the lexical judge Contains, which have up to 34 points lower human alignment. Through error analysis and various other studies, including the effects of instruction length and leniency bias, we hope to provide valuable lessons for using LLMs as judges in the future.