Kalman-Inspired Feature Propagation for Video Face Super-Resolution
Ruicheng Feng, Chongyi Li, Chen Change Loy
2024-08-12
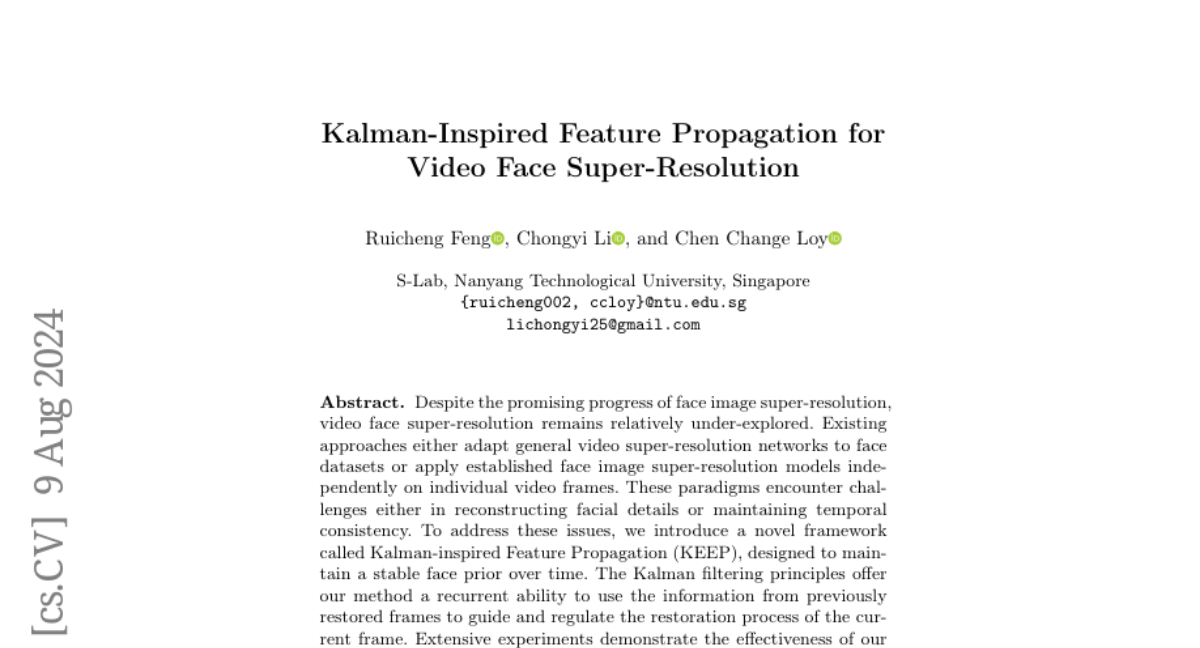
Summary
This paper introduces a new method called Kalman-inspired Feature Propagation (KEEP) for improving the quality of video face super-resolution, which means enhancing the clarity of faces in videos.
What's the problem?
While there has been progress in improving face images, enhancing faces in videos is still a challenge. Existing methods either focus on single frames or struggle to keep the details consistent across multiple frames, leading to problems with facial clarity and continuity in moving images.
What's the solution?
To solve these issues, the authors developed KEEP, which uses principles from Kalman filtering to maintain a stable and consistent representation of faces over time. This method allows the model to use information from previously processed frames to help improve the current frame, ensuring that facial details are restored accurately and consistently throughout the video. The authors conducted extensive experiments to show that KEEP effectively captures facial features across different frames.
Why it matters?
This research is important because it advances the technology used for enhancing video quality, particularly for faces. By improving how well AI can restore and maintain facial details in videos, KEEP can be useful in various applications such as film restoration, virtual reality, and video conferencing, making visuals more realistic and engaging.
Abstract
Despite the promising progress of face image super-resolution, video face super-resolution remains relatively under-explored. Existing approaches either adapt general video super-resolution networks to face datasets or apply established face image super-resolution models independently on individual video frames. These paradigms encounter challenges either in reconstructing facial details or maintaining temporal consistency. To address these issues, we introduce a novel framework called Kalman-inspired Feature Propagation (KEEP), designed to maintain a stable face prior over time. The Kalman filtering principles offer our method a recurrent ability to use the information from previously restored frames to guide and regulate the restoration process of the current frame. Extensive experiments demonstrate the effectiveness of our method in capturing facial details consistently across video frames. Code and video demo are available at https://jnjaby.github.io/projects/KEEP.