Knowledge Composition using Task Vectors with Learned Anisotropic Scaling
Frederic Z. Zhang, Paul Albert, Cristian Rodriguez-Opazo, Anton van den Hengel, Ehsan Abbasnejad
2024-07-10
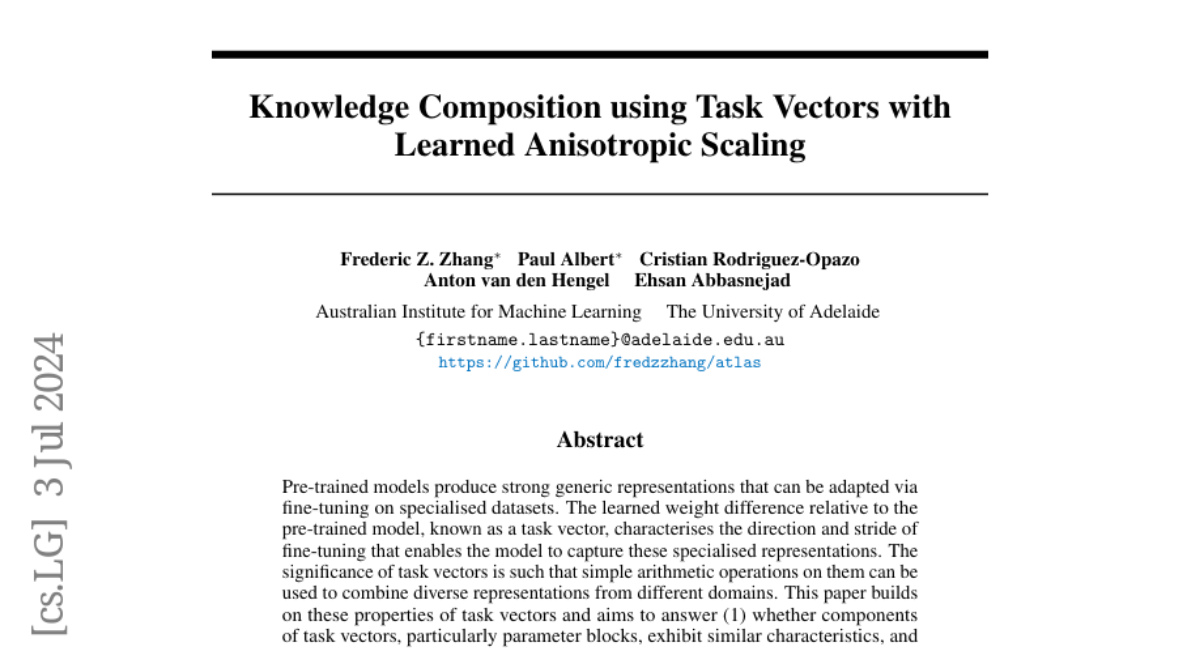
Summary
This paper talks about a new algorithm called aTLAS, which helps improve how we use pre-trained models in machine learning. It focuses on combining different parts of these models effectively to enhance their ability to learn and adapt to new tasks.
What's the problem?
The main problem is that while pre-trained models can be adapted for specific tasks, they often require a lot of data and can struggle to combine information from different areas effectively. This can lead to inefficiencies and difficulties in learning when there isn't much data available or when the tasks are very different from what the model was originally trained on.
What's the solution?
To address this issue, the authors introduced aTLAS, which stands for a new algorithm that combines different parts of task vectors—these are learned representations that help guide how models adapt. aTLAS uses a method called anisotropic scaling, which means it adjusts the way these parts are combined in a flexible manner. This allows the model to better utilize previously learned information, making it less reliant on large datasets. The authors tested aTLAS in various scenarios, showing that it works well even with little or no labeled data and improves the model's ability to generalize across different tasks.
Why it matters?
This research is important because it provides a more efficient way to adapt pre-trained models for new tasks, especially when data is limited. By improving how models combine and use their learned knowledge, aTLAS can lead to better performance in machine learning applications, making AI systems more effective and easier to train.
Abstract
Pre-trained models produce strong generic representations that can be adapted via fine-tuning. The learned weight difference relative to the pre-trained model, known as a task vector, characterises the direction and stride of fine-tuning. The significance of task vectors is such that simple arithmetic operations on them can be used to combine diverse representations from different domains. This paper builds on these properties of task vectors and aims to answer (1) whether components of task vectors, particularly parameter blocks, exhibit similar characteristics, and (2) how such blocks can be used to enhance knowledge composition and transfer. To this end, we introduce aTLAS, an algorithm that linearly combines parameter blocks with different learned coefficients, resulting in anisotropic scaling at the task vector level. We show that such linear combinations explicitly exploit the low intrinsic dimensionality of pre-trained models, with only a few coefficients being the learnable parameters. Furthermore, composition of parameter blocks leverages the already learned representations, thereby reducing the dependency on large amounts of data. We demonstrate the effectiveness of our method in task arithmetic, few-shot recognition and test-time adaptation, with supervised or unsupervised objectives. In particular, we show that (1) learned anisotropic scaling allows task vectors to be more disentangled, causing less interference in composition; (2) task vector composition excels with scarce or no labeled data and is less prone to domain shift, thus leading to better generalisability; (3) mixing the most informative parameter blocks across different task vectors prior to training can reduce the memory footprint and improve the flexibility of knowledge transfer. Moreover, we show the potential of aTLAS as a PEFT method, particularly with less data, and demonstrate that its scalibility.