KV Prediction for Improved Time to First Token
Maxwell Horton, Qingqing Cao, Chenfan Sun, Yanzi Jin, Sachin Mehta, Mohammad Rastegari, Moin Nabi
2024-10-14
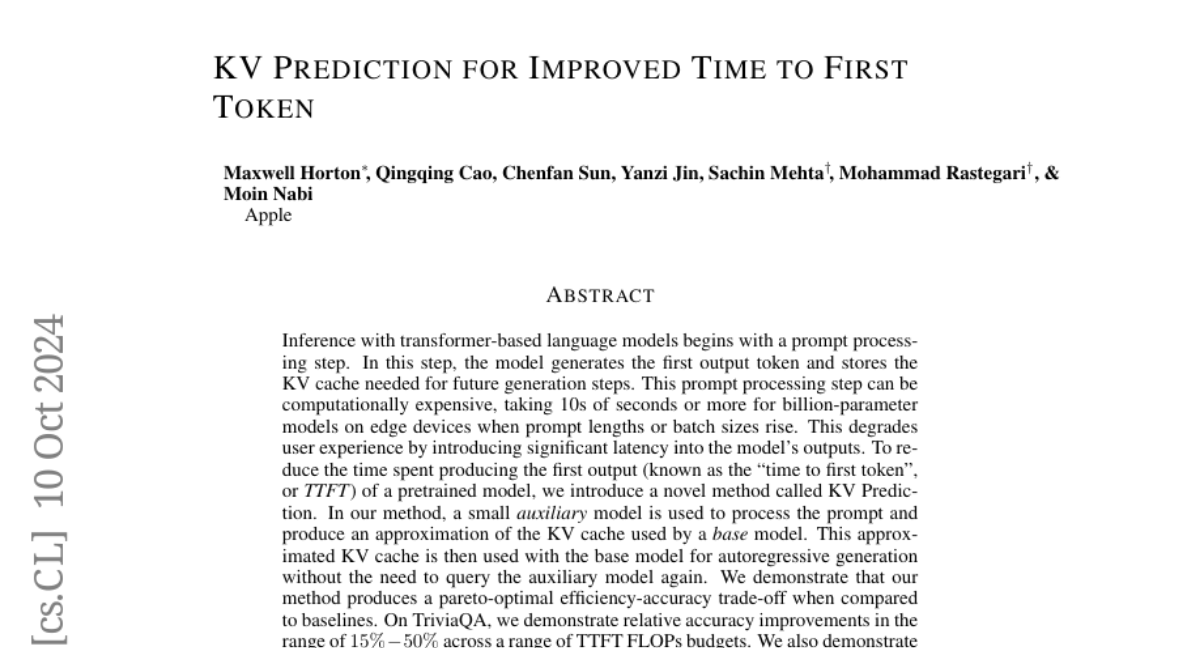
Summary
This paper presents KV Prediction, a new method designed to reduce the time it takes for large language models (LLMs) to generate their first response, known as the 'time to first token' (TTFT).
What's the problem?
When LLMs receive a prompt, they often take a long time to process it and generate the first output. This delay can be frustrating for users, especially when using large models that require significant computing power. As the length of prompts or batch sizes increases, this processing time can extend to several seconds, negatively impacting user experience.
What's the solution?
To solve this problem, the authors propose using a smaller auxiliary model to predict key-value (KV) pairs in advance. This auxiliary model processes the prompt and creates an approximation of the KV cache needed by the main model. By using this predicted KV cache, the main model can start generating output more quickly without needing to repeatedly consult the auxiliary model. The results show that this method significantly improves efficiency while maintaining accuracy.
Why it matters?
This research is important because it enhances the responsiveness of language models, making them more user-friendly for applications like chatbots and virtual assistants. By reducing the time it takes for models to start generating responses, KV Prediction can lead to a better overall experience for users interacting with AI systems.
Abstract
Inference with transformer-based language models begins with a prompt processing step. In this step, the model generates the first output token and stores the KV cache needed for future generation steps. This prompt processing step can be computationally expensive, taking 10s of seconds or more for billion-parameter models on edge devices when prompt lengths or batch sizes rise. This degrades user experience by introducing significant latency into the model's outputs. To reduce the time spent producing the first output (known as the ``time to first token'', or TTFT) of a pretrained model, we introduce a novel method called KV Prediction. In our method, a small auxiliary model is used to process the prompt and produce an approximation of the KV cache used by a base model. This approximated KV cache is then used with the base model for autoregressive generation without the need to query the auxiliary model again. We demonstrate that our method produces a pareto-optimal efficiency-accuracy trade-off when compared to baselines. On TriviaQA, we demonstrate relative accuracy improvements in the range of 15%-50% across a range of TTFT FLOPs budgets. We also demonstrate accuracy improvements of up to 30% on HumanEval python code completion at fixed TTFT FLOPs budgets. Additionally, we benchmark models on an Apple M2 Pro CPU and demonstrate that our improvement in FLOPs translates to a TTFT speedup on hardware. We release our code at https://github.com/apple/corenet/tree/main/projects/kv-prediction .