Kvasir-VQA: A Text-Image Pair GI Tract Dataset
Sushant Gautam, Andrea Storås, Cise Midoglu, Steven A. Hicks, Vajira Thambawita, Pål Halvorsen, Michael A. Riegler
2024-09-04
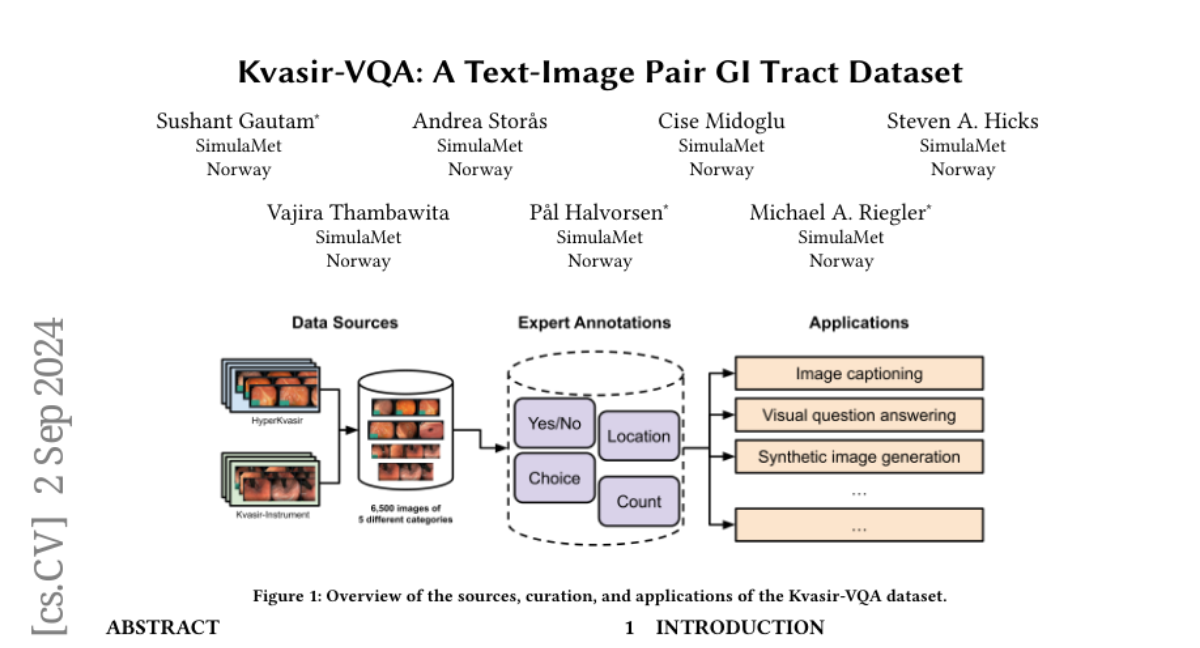
Summary
This paper talks about Kvasir-VQA, a new dataset designed to help improve machine learning models for diagnosing gastrointestinal (GI) conditions using images and questions.
What's the problem?
In the field of medical diagnostics, there is a growing need for better tools that can analyze images of the GI tract and answer questions about various conditions. Traditional datasets often lack the necessary annotations and diversity to train effective machine learning models for these tasks.
What's the solution?
Kvasir-VQA addresses this issue by providing a dataset with 6,500 annotated images related to different GI tract conditions and surgical instruments. It includes various types of questions, such as yes/no, multiple choice, and numerical counts. This dataset can be used for several applications, including image captioning, Visual Question Answering (VQA), object detection, and classification. The authors conducted experiments to show how effective this dataset is for training models in medical image analysis.
Why it matters?
This research is important because it enhances the tools available for medical professionals to diagnose GI conditions more accurately and efficiently. By providing a comprehensive dataset with diverse annotations, Kvasir-VQA can lead to better training of AI models, ultimately improving patient care and outcomes in healthcare.
Abstract
We introduce Kvasir-VQA, an extended dataset derived from the HyperKvasir and Kvasir-Instrument datasets, augmented with question-and-answer annotations to facilitate advanced machine learning tasks in Gastrointestinal (GI) diagnostics. This dataset comprises 6,500 annotated images spanning various GI tract conditions and surgical instruments, and it supports multiple question types including yes/no, choice, location, and numerical count. The dataset is intended for applications such as image captioning, Visual Question Answering (VQA), text-based generation of synthetic medical images, object detection, and classification. Our experiments demonstrate the dataset's effectiveness in training models for three selected tasks, showcasing significant applications in medical image analysis and diagnostics. We also present evaluation metrics for each task, highlighting the usability and versatility of our dataset. The dataset and supporting artifacts are available at https://datasets.simula.no/kvasir-vqa.