L4GM: Large 4D Gaussian Reconstruction Model
Jiawei Ren, Kevin Xie, Ashkan Mirzaei, Hanxue Liang, Xiaohui Zeng, Karsten Kreis, Ziwei Liu, Antonio Torralba, Sanja Fidler, Seung Wook Kim, Huan Ling
2024-06-18
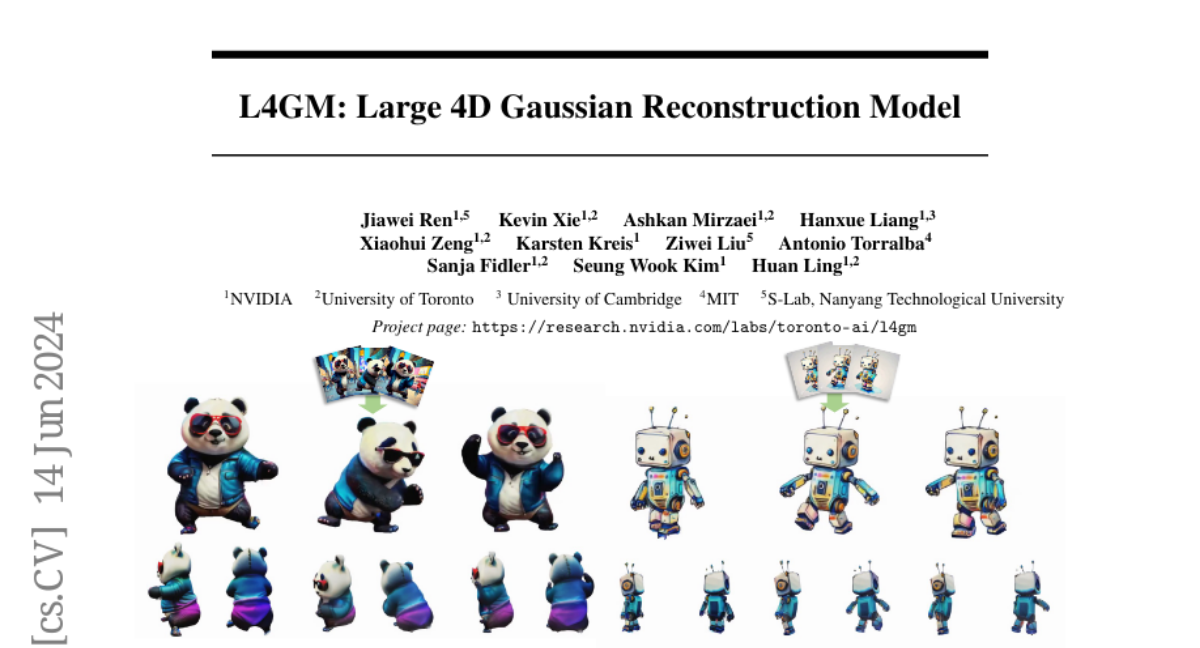
Summary
This paper introduces L4GM, a groundbreaking model that can create animated 3D objects from a single video input. It does this quickly, in just one second, and is designed to work efficiently with a new dataset of videos featuring animated objects.
What's the problem?
Creating realistic 3D animations from videos can be challenging because existing methods often require multiple views or extensive processing time. Many models struggle to generate smooth animations and maintain quality when working with just one video. This limits their usefulness in applications like gaming and animation where quick and accurate 3D representations are needed.
What's the solution?
To solve this problem, the authors developed L4GM, which builds on a previous model called LGM that creates 3D shapes from multiple images. L4GM uses a special dataset containing over 12 million videos of animated objects viewed from different angles. It processes these videos to produce a representation of the object in 3D for each frame. The model incorporates advanced techniques like temporal self-attention, which helps it keep track of changes over time, and an interpolation method to create smoother animations by generating frames between the original ones.
Why it matters?
This research is important because it makes it easier and faster to create high-quality animated 3D models from simple video inputs. By improving how AI can understand and generate animations, L4GM has the potential to enhance various industries, including film, video games, and virtual reality. This advancement could lead to more realistic and engaging experiences in digital media.
Abstract
We present L4GM, the first 4D Large Reconstruction Model that produces animated objects from a single-view video input -- in a single feed-forward pass that takes only a second. Key to our success is a novel dataset of multiview videos containing curated, rendered animated objects from Objaverse. This dataset depicts 44K diverse objects with 110K animations rendered in 48 viewpoints, resulting in 12M videos with a total of 300M frames. We keep our L4GM simple for scalability and build directly on top of LGM, a pretrained 3D Large Reconstruction Model that outputs 3D Gaussian ellipsoids from multiview image input. L4GM outputs a per-frame 3D Gaussian Splatting representation from video frames sampled at a low fps and then upsamples the representation to a higher fps to achieve temporal smoothness. We add temporal self-attention layers to the base LGM to help it learn consistency across time, and utilize a per-timestep multiview rendering loss to train the model. The representation is upsampled to a higher framerate by training an interpolation model which produces intermediate 3D Gaussian representations. We showcase that L4GM that is only trained on synthetic data generalizes extremely well on in-the-wild videos, producing high quality animated 3D assets.