Language Models And A Second Opinion Use Case: The Pocket Professional
David Noever
2024-10-29
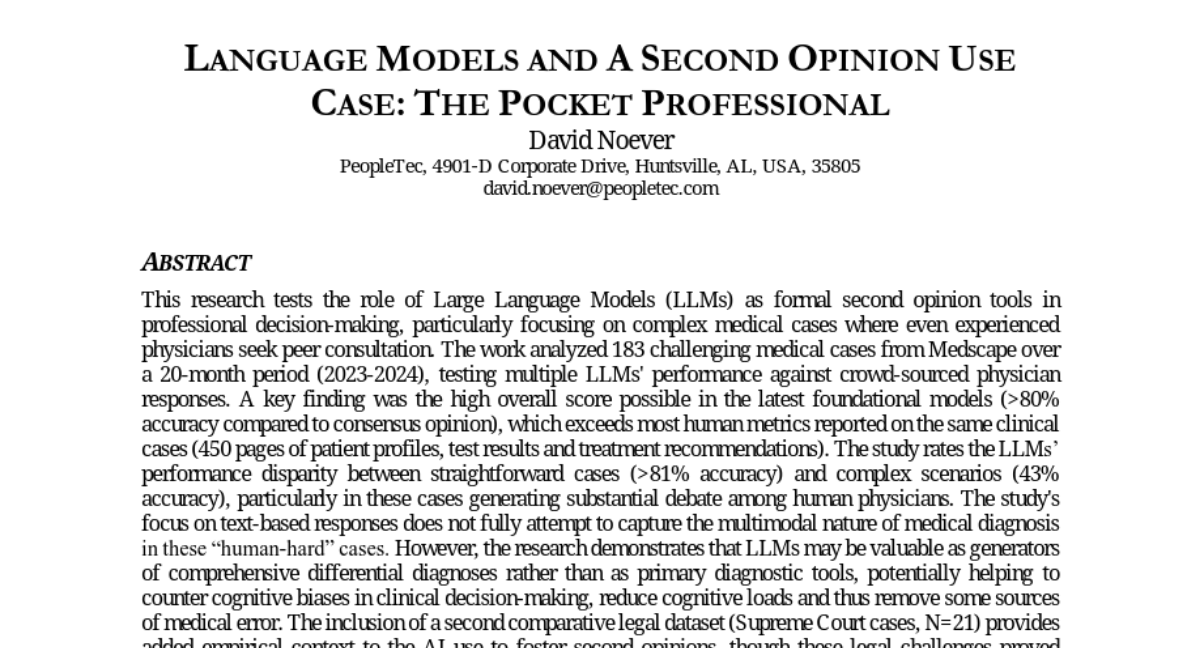
Summary
This paper explores how Large Language Models (LLMs) can be used as second opinion tools in medical decision-making, especially for complex cases where doctors might seek additional advice.
What's the problem?
In complex medical situations, even experienced doctors sometimes need a second opinion to ensure they are making the right decisions. However, getting reliable second opinions can be difficult and time-consuming. Traditional methods of peer consultation may not always be available or efficient, leading to potential errors in diagnosis and treatment.
What's the solution?
The authors studied 183 challenging medical cases and tested various LLMs to see how well they could provide second opinions compared to human doctors. They found that the latest LLMs achieved over 80% accuracy in diagnosing cases, which is better than what many human doctors could do. The study showed that LLMs could generate useful differential diagnoses (lists of possible conditions) based on the information provided, helping to reduce cognitive load on physicians and potentially improving patient outcomes.
Why it matters?
This research is significant because it suggests that LLMs could serve as valuable tools for doctors, especially in difficult cases. By providing accurate second opinions, these models can help improve diagnostic accuracy and support better decision-making in healthcare. This could ultimately lead to better patient care and outcomes, making LLMs an important addition to the medical field.
Abstract
This research tests the role of Large Language Models (LLMs) as formal second opinion tools in professional decision-making, particularly focusing on complex medical cases where even experienced physicians seek peer consultation. The work analyzed 183 challenging medical cases from Medscape over a 20-month period, testing multiple LLMs' performance against crowd-sourced physician responses. A key finding was the high overall score possible in the latest foundational models (>80% accuracy compared to consensus opinion), which exceeds most human metrics reported on the same clinical cases (450 pages of patient profiles, test results). The study rates the LLMs' performance disparity between straightforward cases (>81% accuracy) and complex scenarios (43% accuracy), particularly in these cases generating substantial debate among human physicians. The research demonstrates that LLMs may be valuable as generators of comprehensive differential diagnoses rather than as primary diagnostic tools, potentially helping to counter cognitive biases in clinical decision-making, reduce cognitive loads, and thus remove some sources of medical error. The inclusion of a second comparative legal dataset (Supreme Court cases, N=21) provides added empirical context to the AI use to foster second opinions, though these legal challenges proved considerably easier for LLMs to analyze. In addition to the original contributions of empirical evidence for LLM accuracy, the research aggregated a novel benchmark for others to score highly contested question and answer reliability between both LLMs and disagreeing human practitioners. These results suggest that the optimal deployment of LLMs in professional settings may differ substantially from current approaches that emphasize automation of routine tasks.