Large Language Models Assume People are More Rational than We Really are
Ryan Liu, Jiayi Geng, Joshua C. Peterson, Ilia Sucholutsky, Thomas L. Griffiths
2024-06-26
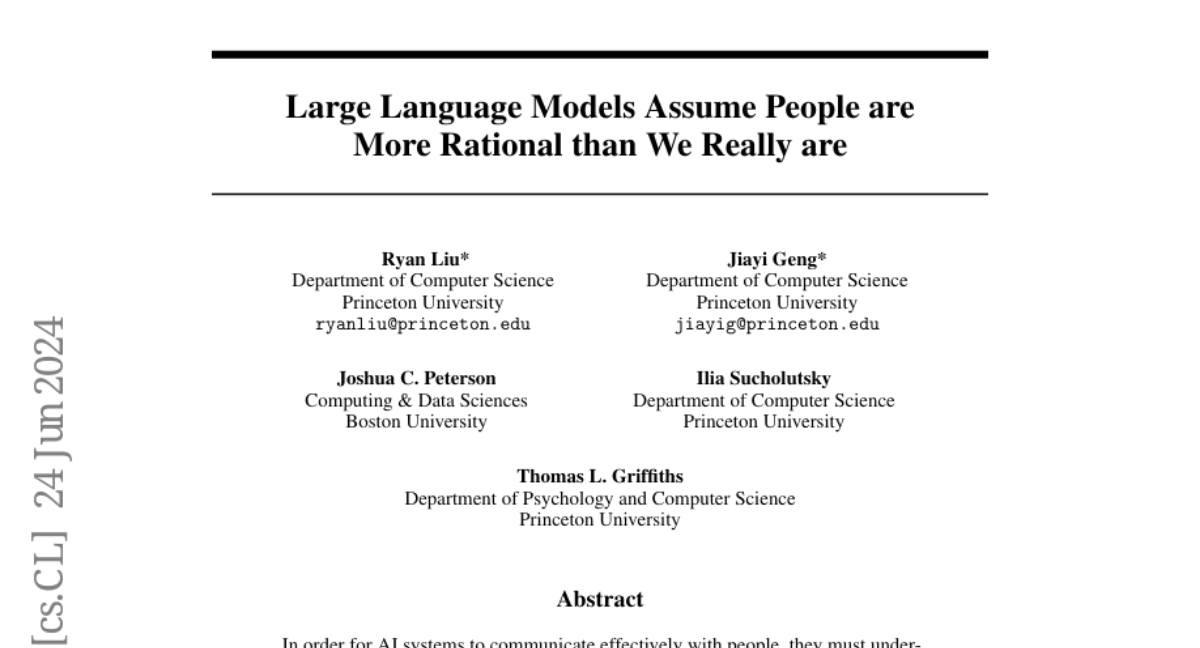
Summary
This paper discusses how large language models (LLMs) like GPT-4 and Claude 3 make assumptions about human decision-making. It reveals that these models often think people are more rational than they actually are, which can lead to misunderstandings in how AI interacts with humans.
What's the problem?
For AI systems to communicate effectively with people, they need to understand how humans make decisions. However, people don't always make rational choices; sometimes they act based on emotions, biases, or other factors. If LLMs assume that people are always rational, they might not accurately predict or respond to human behavior, which can lead to ineffective interactions.
What's the solution?
The authors analyzed the behavior of several advanced LLMs and compared their predictions about human decisions to actual human decision-making data. They found that these models tend to align more closely with traditional theories of rational choice, which suggest that people always make the best possible decisions based on available information. In reality, people often make less rational choices. The study highlights that while LLMs mimic human behavior in some ways, they fail to capture the full complexity of human decision-making.
Why it matters?
This research is important because it sheds light on the limitations of AI in understanding human behavior. By recognizing that LLMs may misinterpret how people actually think and decide, developers can work on improving these models to create better AI systems that interact more effectively with humans. Understanding these differences is crucial for applications in fields like customer service, mental health support, and any area where AI needs to understand human emotions and choices.
Abstract
In order for AI systems to communicate effectively with people, they must understand how we make decisions. However, people's decisions are not always rational, so the implicit internal models of human decision-making in Large Language Models (LLMs) must account for this. Previous empirical evidence seems to suggest that these implicit models are accurate -- LLMs offer believable proxies of human behavior, acting how we expect humans would in everyday interactions. However, by comparing LLM behavior and predictions to a large dataset of human decisions, we find that this is actually not the case: when both simulating and predicting people's choices, a suite of cutting-edge LLMs (GPT-4o & 4-Turbo, Llama-3-8B & 70B, Claude 3 Opus) assume that people are more rational than we really are. Specifically, these models deviate from human behavior and align more closely with a classic model of rational choice -- expected value theory. Interestingly, people also tend to assume that other people are rational when interpreting their behavior. As a consequence, when we compare the inferences that LLMs and people draw from the decisions of others using another psychological dataset, we find that these inferences are highly correlated. Thus, the implicit decision-making models of LLMs appear to be aligned with the human expectation that other people will act rationally, rather than with how people actually act.