Large-Scale Multi-omic Biosequence Transformers for Modeling Peptide-Nucleotide Interactions
Sully F. Chen, Robert J. Steele, Beakal Lemeneh, Shivanand P. Lad, Eric Oermann
2024-09-02
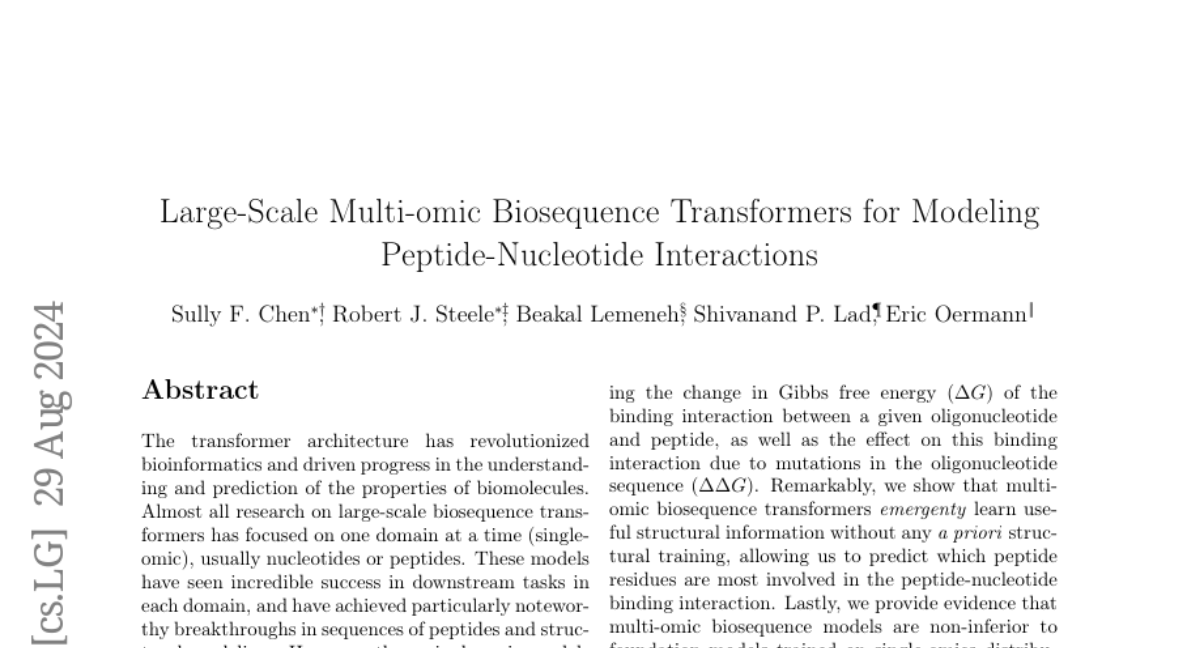
Summary
This paper talks about a new approach to using transformer models in bioinformatics to study how peptides (short chains of amino acids) and nucleotides (building blocks of DNA and RNA) interact with each other.
What's the problem?
Most research in bioinformatics has focused on either peptides or nucleotides separately, which means we lack models that can effectively analyze how these two important biological components interact. This limits our understanding of many biological processes.
What's the solution?
The authors introduce a new type of model called a multi-omic foundation model that can learn from both peptide and nucleotide data at the same time. They trained this model using a dataset that includes various sequences without needing any labeled data. The model can predict important interactions, such as how changes in nucleotide sequences affect their binding with peptides, achieving high accuracy in its predictions.
Why it matters?
This research is significant because it helps scientists better understand the complex interactions between different biological molecules. By developing models that can analyze multiple types of biological data together, we can gain insights that could lead to advancements in areas like drug development and personalized medicine.
Abstract
The transformer architecture has revolutionized bioinformatics and driven progress in the understanding and prediction of the properties of biomolecules. Almost all research on large-scale biosequence transformers has focused on one domain at a time (single-omic), usually nucleotides or peptides. These models have seen incredible success in downstream tasks in each domain and have achieved particularly noteworthy breakthroughs in sequences of peptides and structural modeling. However, these single-omic models are naturally incapable of modeling multi-omic tasks, one of the most biologically critical being nucleotide-peptide interactions. We present our work training the first multi-omic nucleotide-peptide foundation models. We show that these multi-omic models (MOMs) can learn joint representations between various single-omic distributions that are emergently consistent with the Central Dogma of molecular biology, despite only being trained on unlabeled biosequences. We further demonstrate that MOMs can be fine-tuned to achieve state-of-the-art results on peptide-nucleotide interaction tasks, namely predicting the change in Gibbs free energy ({\Delta}G) of the binding interaction between a given oligonucleotide and peptide, as well as the effect on this binding interaction due to mutations in the oligonucleotide sequence ({\Delta}{\Delta}G). Remarkably, we show that multi-omic biosequence transformers emergently learn useful structural information without any prior structural training, allowing us to predict which peptide residues are most involved in the peptide-nucleotide binding interaction. Lastly, we provide evidence that multi-omic biosequence models are non-inferior to foundation models trained on single-omics distributions, suggesting a more generalized or foundational approach to building these models.