Large-Scale Text-to-Image Model with Inpainting is a Zero-Shot Subject-Driven Image Generator
Chaehun Shin, Jooyoung Choi, Heeseung Kim, Sungroh Yoon
2024-11-25
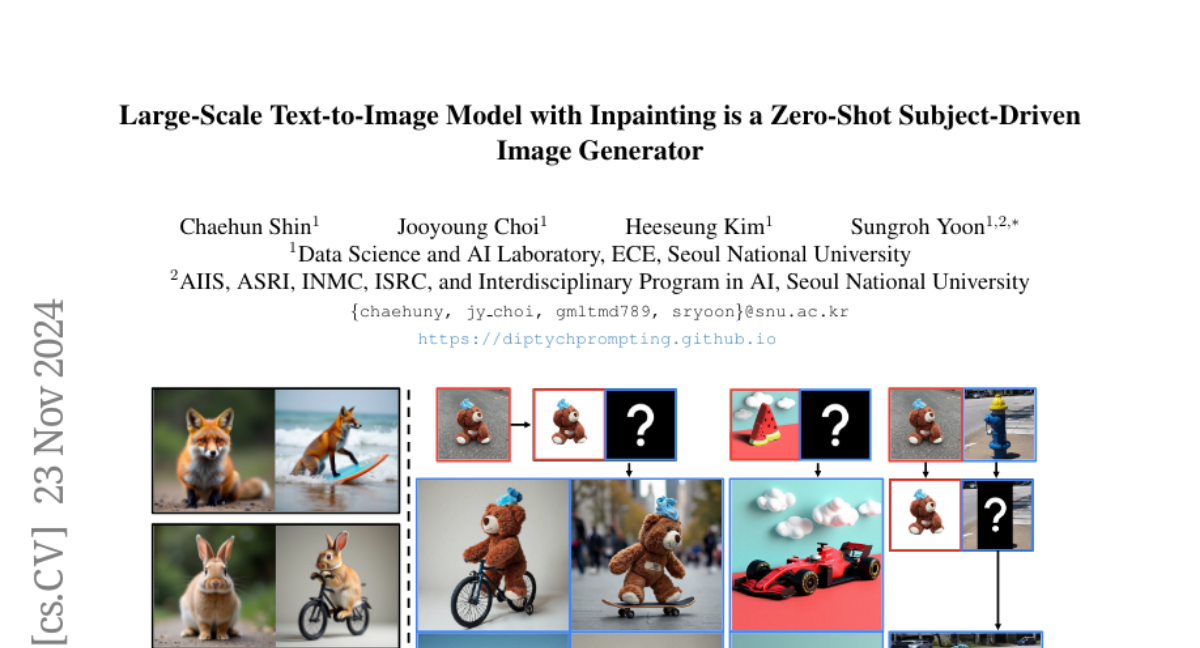
Summary
This paper introduces Diptych Prompting, a new method for generating images based on a specific subject and context without needing extensive prior training, using a technique called inpainting.
What's the problem?
Generating images that accurately reflect a new subject in a specific context can be challenging. Traditional methods often require a lot of time and resources to fine-tune the model for each new subject, which can be inefficient. Recent zero-shot approaches try to generate images quickly but often fail to align the subject correctly with the context described in the text prompt.
What's the solution?
Diptych Prompting reinterprets the image generation task as an inpainting problem. It uses a two-panel setup where the left panel contains a reference image of the subject, while the right panel is generated based on a text description. This method allows the model to focus on creating an accurate representation of the subject while ensuring it fits well within the desired context. The authors also improve the process by removing backgrounds from reference images to avoid unwanted elements and enhancing details during generation.
Why it matters?
This research is important because it provides a more efficient way to create high-quality images that accurately represent specific subjects and contexts. By improving how models generate images without extensive training, Diptych Prompting opens up new possibilities for applications in art, design, and content creation, making it easier for users to produce tailored visual content.
Abstract
Subject-driven text-to-image generation aims to produce images of a new subject within a desired context by accurately capturing both the visual characteristics of the subject and the semantic content of a text prompt. Traditional methods rely on time- and resource-intensive fine-tuning for subject alignment, while recent zero-shot approaches leverage on-the-fly image prompting, often sacrificing subject alignment. In this paper, we introduce Diptych Prompting, a novel zero-shot approach that reinterprets as an inpainting task with precise subject alignment by leveraging the emergent property of diptych generation in large-scale text-to-image models. Diptych Prompting arranges an incomplete diptych with the reference image in the left panel, and performs text-conditioned inpainting on the right panel. We further prevent unwanted content leakage by removing the background in the reference image and improve fine-grained details in the generated subject by enhancing attention weights between the panels during inpainting. Experimental results confirm that our approach significantly outperforms zero-shot image prompting methods, resulting in images that are visually preferred by users. Additionally, our method supports not only subject-driven generation but also stylized image generation and subject-driven image editing, demonstrating versatility across diverse image generation applications. Project page: https://diptychprompting.github.io/