LARP: Tokenizing Videos with a Learned Autoregressive Generative Prior
Hanyu Wang, Saksham Suri, Yixuan Ren, Hao Chen, Abhinav Shrivastava
2024-10-29
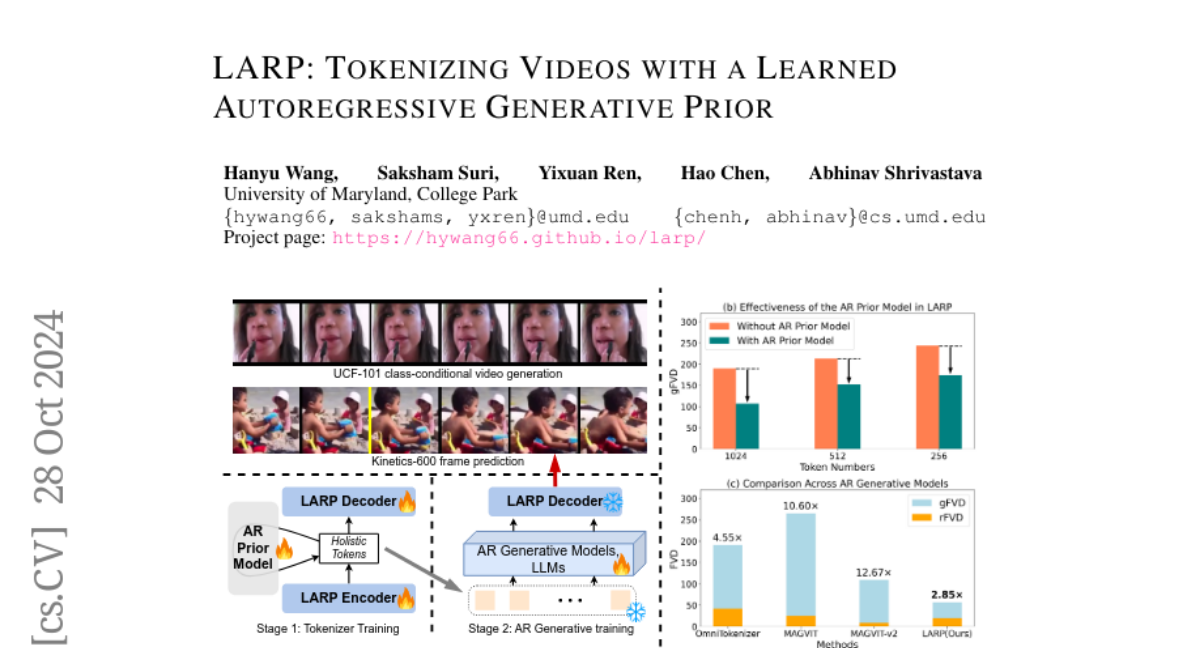
Summary
This paper introduces LARP, a new method for converting videos into a format that can be easily processed by AI models, using advanced techniques to improve video tokenization.
What's the problem?
Current methods for tokenizing videos often break them down into small parts, which limits the model's ability to understand the overall context of the video. These traditional methods focus on local details but miss out on the bigger picture, making it hard for AI models to generate or analyze videos effectively.
What's the solution?
LARP (Learned Autoregressive Generative Prior) proposes a new way to tokenize videos by using holistic queries that capture more global information about the video content. Instead of just looking at small patches, LARP allows for flexible tokenization that adapts to the needs of different tasks. It also uses an autoregressive transformer model during training to predict the next token based on previous ones, which helps create a more organized and efficient way to handle video data. This method results in better performance in generating and analyzing videos compared to older techniques.
Why it matters?
This research is important because it enhances how AI models can work with video data, making them more effective for tasks like video editing, compression, and generation. By improving video tokenization, LARP opens up new possibilities for creating high-quality multimedia content and could lead to advancements in various applications, including entertainment and education.
Abstract
We present LARP, a novel video tokenizer designed to overcome limitations in current video tokenization methods for autoregressive (AR) generative models. Unlike traditional patchwise tokenizers that directly encode local visual patches into discrete tokens, LARP introduces a holistic tokenization scheme that gathers information from the visual content using a set of learned holistic queries. This design allows LARP to capture more global and semantic representations, rather than being limited to local patch-level information. Furthermore, it offers flexibility by supporting an arbitrary number of discrete tokens, enabling adaptive and efficient tokenization based on the specific requirements of the task. To align the discrete token space with downstream AR generation tasks, LARP integrates a lightweight AR transformer as a training-time prior model that predicts the next token on its discrete latent space. By incorporating the prior model during training, LARP learns a latent space that is not only optimized for video reconstruction but is also structured in a way that is more conducive to autoregressive generation. Moreover, this process defines a sequential order for the discrete tokens, progressively pushing them toward an optimal configuration during training, ensuring smoother and more accurate AR generation at inference time. Comprehensive experiments demonstrate LARP's strong performance, achieving state-of-the-art FVD on the UCF101 class-conditional video generation benchmark. LARP enhances the compatibility of AR models with videos and opens up the potential to build unified high-fidelity multimodal large language models (MLLMs).